INTRODUCTION
The porcine reproductive trait is one of the most important factors in the competitiveness and efficiency of the pig industry, and the reproductive trait of female pigs in particular is directly related to porcine reproductive efficiency [1,2]. The reproductive efficiency in female pigs remarkably affects the litter size, fertility, and hormonal metabolism of pigs [3–5]. Various hormones interacting with one another according to the estrous cycle in turn influence reproductive efficiency [6–8]. Since hormones are secreted and regulated by several reproductive tissues during the estrous cycle, porcine reproductive efficiency is closely associated with molecular mechanisms involved in pig reproductive tissues [9]. Among these reproductive tissues, the ovaries are important because they are a source of reproductive hormones and follicles [10]. They also respond to vital hormones secreted by other organs, especially the pituitary gland, which produces major steroid hormones (estradiol [E4] and progesterone [P2]) and peptide growth factors implicated in the functioning of the ovaries [9,11]. In addition, the oviduct carries follicles from the ovary to the uterus, and its metabolites are affected by ovarian hormones such as E4 and P2 [12,13]. The follicles that ovulate from the ovary move through the oviduct into the uterus. The endometrium is an inner wall of the uterus that prepares for implantation through morphological and functional changes; it also communicates with the ovary through the secretion of prostaglandin F2α (PGF2α) [6,14,15]. Despite relevant studies, the interaction and hormone control mechanisms of reproductive tissues according to the estrous cycle remain difficult and complex. Previous studies showed that a co-expression network can be integrated using whole transcriptomes in each tissue [16,17] and multiple reproductive tissues [6,16,17], but the synchronized interaction of pig reproductive tissues according to the estrous cycle is still insufficient because it is too complicated to be identified only via analysis at the mRNA level.
With the development of next generation sequencing (NGS) technology, noncoding RNAs, which occupy much more areas but do not encode proteins, can be examined [18]. Among noncoding RNAs, long noncoding RNAs (lncRNAs) with a length of 200 bp or more have lower gene expression levels than protein-coding genes, but genes are regulated through transcriptive regulation, post-transcriptive regulation, and RNA interference [19–22]. Most lncRNAs are located in the chromatin and can interact with proteins to promote or inhibit DNA activity in the target DNA region [23]. Therefore, lncRNAs play an important role in genome studies, such as those focusing on mRNA regulation; their characterization in the animal genome can be a key to bridging the gap between genotypes and phenotypes [24]. In addition, the importance of lncRNA is being studied in the fields of infectious diseases, immunity, and pathology; it is considered an important factor in stress response and development regulation in animals [7,25,26]. Thus, lncRNAs have crucial roles in tissue- and species-specific regulation of biological processes (BP) in various species and tissues [27–30]. Currently, studies involving lncRNA analysis have been conducted in pig reproductive tissues, but only a few have explored a single tissue (ovary, oviduct, endometrium) [7,14,31].
Multi-omics analyses have been conducted to obtain complex BP holistically [32,33], and lncRNAs have been considered as a factor for such integrated analysis [34]. The limitations of mRNA-level analysis can be complemented by analyzing the mechanism by which lncRNA manipulates mRNA. In this study, candidate novel lncRNAs were determined, and novel and known lncRNA expression patterns were explored via RNA-seq in three reproductive tissues (ovary, oviduct, and endometrium) throughout the porcine estrous cycle. Significant lncRNA–mRNA pairs were determined to identify tissue- and period-specific lncRNA and mRNA expression and to construct the main lncRNA networks. This study was conducted to confirm the characteristics of lncRNAs according to estrous cycle and reproductive tissues and identify the relationship between lncRNAs and mRNAs by verifying the regulatory mechanisms of lncRNAs to mRNAs.
METHOD AND METHODS
All animal experimental procedures were approved by the Institutional Animal Care and Use Committee of the National Institute of Animal Science, Republic of Korea (No. 2015-137) and conducted following the Guide for Care and Use of Animals in Research.
Twenty-one crossbred (Landrace × Yorkshire) gilts (age = 6–8 months; weight = 100–120 kg) that underwent at least two normal estrous cycles were used in this study. Three reproductive tissues (ovary, oviduct, and endometrium) of the gilts were collected for sequencing (Fig. 1) on days 0 (designated as the first day of detection of estrous behavior; D00), 3 (D03), 6 (D06), 9 (D09), 12 (D12), 15 (D15), and 18 (D18).
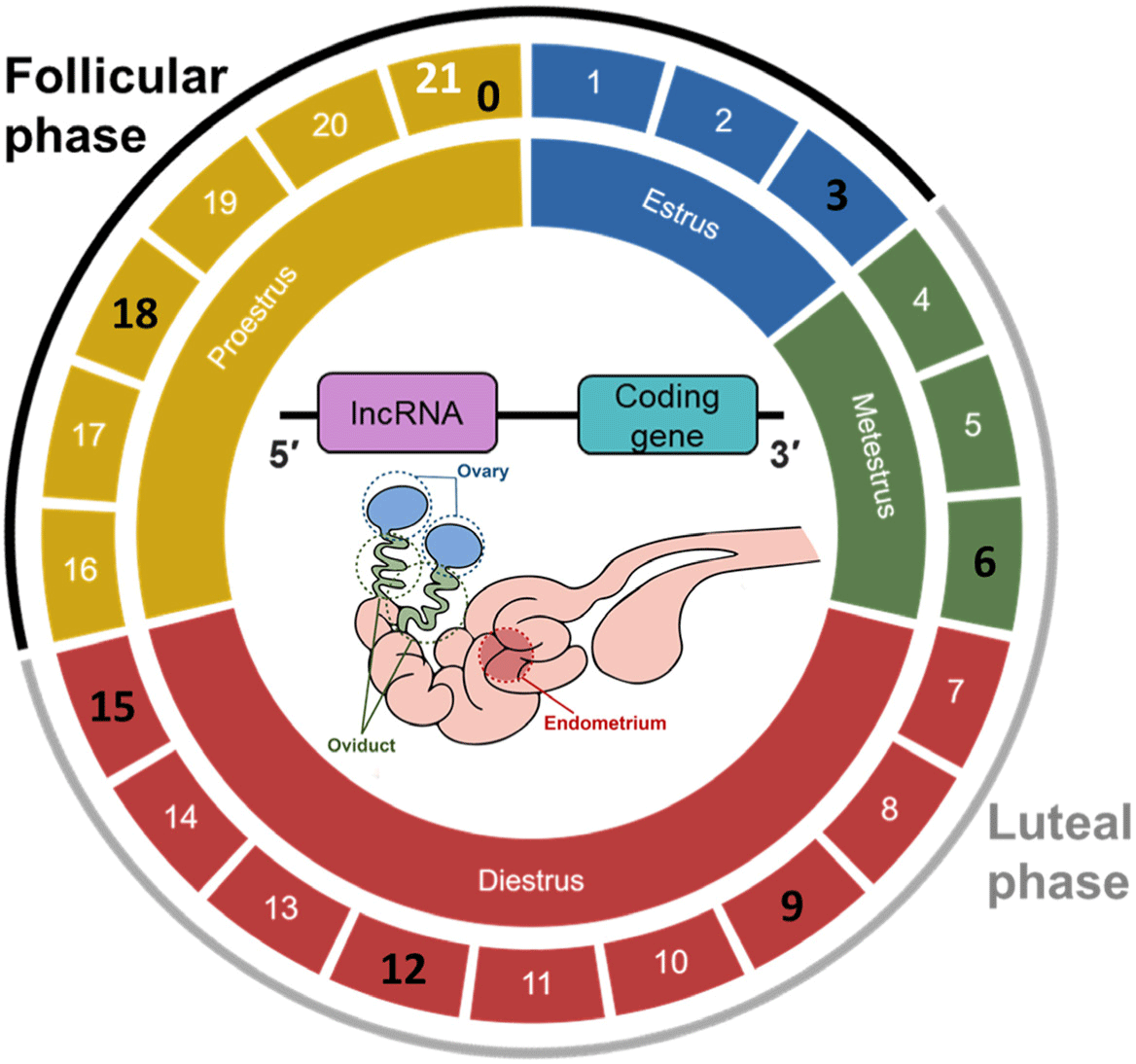
Total RNA was extracted from all reproductive tissues by using TRIzol reagent (Invitrogen, Life Technology, Carlsbad, CA, USA). RNA integrity was evaluated through electrophoresis in 1% agarose gel, and RNA quantity was verified using a NanoDrop ND-1000 spectrophotometer (NanoDrop Technologies, Wilmington, DE, USA). Individual libraries were prepared using an Illumina TruSeq RNA sample preparation kit, and sequencing was performed using cDNA libraries constructed via the 100-base pair (bp) pair-end method in llumina HiSeq 2000. All raw RNA-seq data were deposited at the NCBI Gene Expression Omnibus (https://www.ncbi.nlm.nih.gov/geo/) under the accession number GSE108570. Relevant information was described in detail in our previous study [6,16].
FastQC software (v.0.11.8) was used to check the quality of raw reads of each sample. For quality control, the reads were processed using Trimmomatic software (v0.39), which removes adaptors and low-quality reads. Hisat2 (v2.1.0) was utilized to map clean reads to the reference genome (Sus scrofa Sscrofa 11.1.99, GCA_000003025.6) of the Ensembl genome browser (http://www.ensembl.org/Sus_scrofa/) as a basis for vsubsequent StringTie application. Samtools (v1.9) was also used to sort the reads mapped in accordance with the criteria for subsequent StringTie application. The transcript assembly of each sample sorted by the StringTie (v2.1.3b) program was prepared using the reference annotation file (Sus scrofa Sscrofa 11.1.99). The GTF files of each obtained sample were merged and compared through the gffcompare program (v 0.11.6) and converted through the gffread (v 0.11.8). For the screening of potential novel lncRNAs, those with transcript lengths longer than 200 bp and those with class codes “I”, “j”, “o”, “u”, and “x” were selected. TransDecoder (v5.5.0) was used to determine the transcripts with an open reading frame (ORF) length of less than 300 bp. Furthermore, Coding Potential Calculator2 (CPC2), predictor of lncRNAs and messenger RNAs based on an improved k-mer scheme (PLEK) v1.2, RNA coding potential assessment tool (CPAT) v1.2.4, and Coding-Non-Coding Index (CNCI) were used to predict the coding potential of the remaining transcripts. Transcripts with a high probability of non-coding were selected using information from the protein families (PFAM) v33.0 and the RNA families (RFAM) v14.2 databases. The HMMER program (v3.3) was used to filter the known protein family domain, and the internal program (v1.1.2) was used to filter housekeeping RNAs. Transcripts presumed to be potential lncRNAs were removed in only 1 out of 68 samples (ovary, oviduct, endometrium). The processes used to identify novel lncRNAs are illustrated in Fig. 2A.
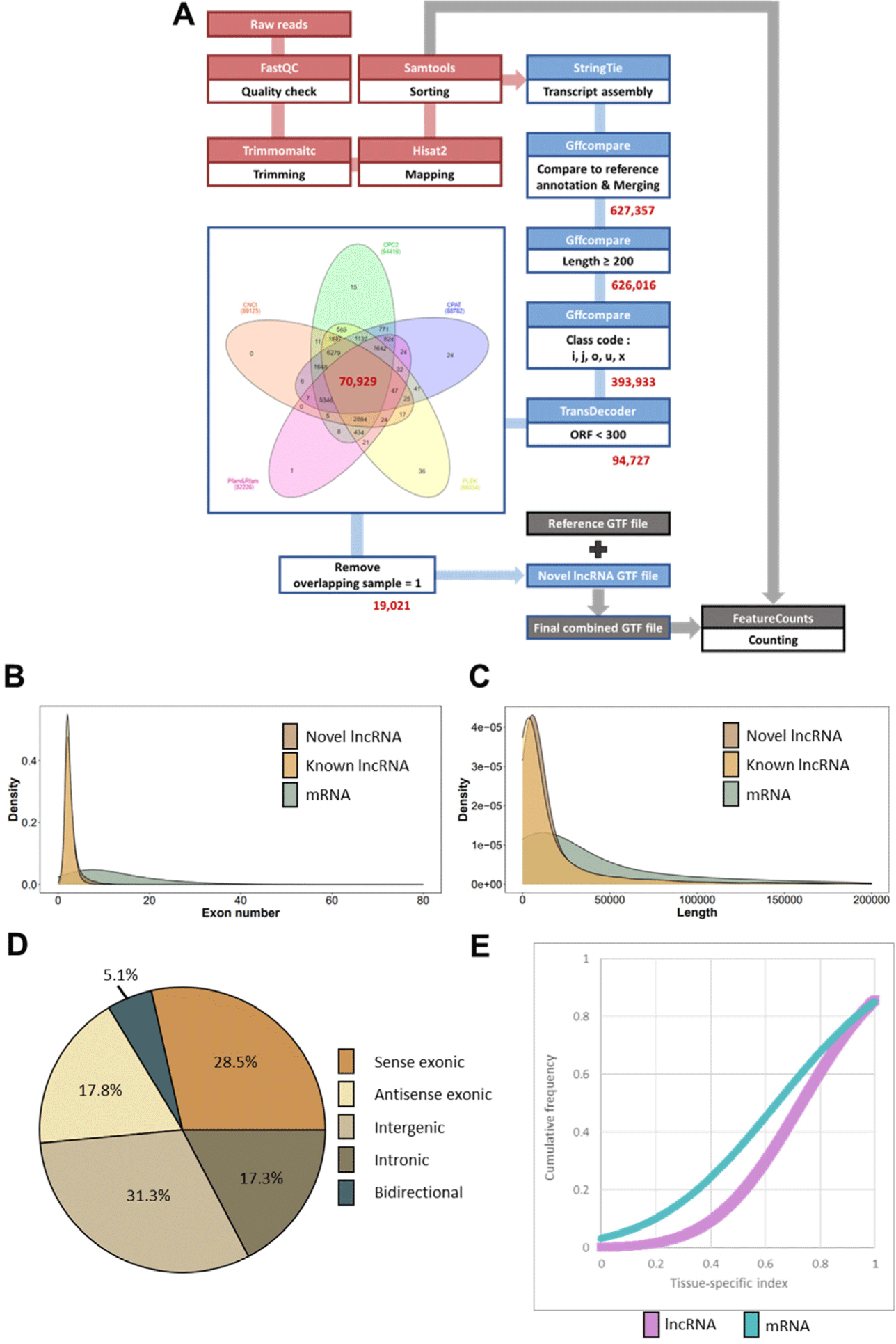
Various genomic characteristics were identified to confirm the specific properties of the newly obtained lncRNA. The number of exons, transcript length, and genomic location were determined through a final combined GTF file (Fig. 2A). Since tissue specificity is a notable feature of lncRNA, the tissue specificity index (TSI) of lncRNA was calculated. The lncRNAs used included the novel lncRNAs and known lncRNAs. The TSI is calculated as follows:
where N is the number of tissues, and xi is the fragment per kilobase of exon per million mapped fragments (FPKM) value of lncRNA/mRNA x in tissue i normalized by the maximum value of FPKM [35].
The expression patterns were obtained to determine tissue-specific and timepoint-specific lncRNAs and mRNAs according to the estrous cycle. The trimmed mean of M (TMM) value was used to normalize raw counts and estimate the expression levels of lncRNAs and mRNAs. Differential expression analyses were performed at each time point (D03, D06, D09, D12, D15, and D18) and compared with those at D00 for each tissue (ovary, oviduct, and endometrium) by using the R package (edge R 3.32.1); an absolute log2 fold-change (FC) of ≥ 1 and false discovery rate (FDR) of < 0.05 were used as cutoff criteria for differentially expressed (DE) lncRNAs and mRNAs. A multidimensional scaling (MDS) plot and a volcano plot of DE lncRNAs and mRNAs were drawn by ggplot2 and limma function of the R package.
Network analysis was conducted via weighted gene co-expression network analysis (WGCNA) package in R to examine their correlation between lncRNAs and phenotypes (tissue and time specific according to the estrous cycle and hormonal changes). Hormone-related traits consisting of steroid hormones (follicle-stimulating hormone [FSH], luteinizing hormone [LH], P4, E2, inhibin) that play important roles in the estrous cycle [11]. Hormone data were measured from blood samples at seven time points (D00, D03, 06, D09, D12, D15, and D18) over the estrous cycle, and detailed methods and explanations are described in the paper [36]. The content of these hormonal traits is summarized in Supplementary Table S1. Correlations were calculated on the basis of co-expression, and the module (network) with high correlation was constructed using the log2TMM value of lncRNA. In the case of hormone traits, the data corresponding to each estrous cycle at seven time points were analyzed for correlation and expressed as a correlation coefficient. Among the modules, three tissue-specific modules (ovary, oviduct, endometrium specific) and one hormone-specific module were selected. Network visualization was performed using the Cytoscape (v3.8.2) software.
Cis-acting genes were confirmed from the main lncRNAs in four networks. Cis-regulatory elements or cis-regulatory modules are noncoding DNA regions that regulate the transcription of neighboring genes [37]. In cis, lncRNA regulates nearby sequences in the genome, and various related results confirmed that such lncRNAs are found within 100 kb of transcriptional regulation-related coding genes [37]. Therefore, coding genes were searched within 100 kb upstream and downstream of the main lncRNA because the cis-activity of lncRNA is related to interaction with adjacent target genes [35,38]. The expression patterns of lncRNAs and their cis-acting genes of each network in tissues and time point were confirmed using Multi Experiment Viewer (MeV v4.9.0) by the k-means clustering algorithm. The number of the main lncRNAs and main DE lncRNAs was shown as a circos plot; their cis-acting genes and DE cis-acting genes were determined using the circa program (v1.2.1).
Database for annotation, visualization, and integrated discovery (DAVID) 2021 was used to annotate the gene ontology (GO) term and the Kyoto Encyclopedia of Genes and Genomes (KEGG) pathways by using the DE cis-acting gene of lncRNAs in the four networks. The ClueGo (v2.5.7) plugin of Cytoscape (v3.8.2) was used to visualize GO terms and KEGG pathways. A pie chart was drawn for the path that met the threshold value of p < 0.05, and the KEGG pathways were visualized as a bar graph with −log10p-value and fold enrichment.
RESULTS
The RNA-seq reads were generated at seven time points during the estrous cycle in three tissues (ovary, oviduct, and endometrium; Fig. 1) [6]. In 67 tissue samples (from three tissues), 1.3 billion sequence reads were produced with an average of 19.5 million per sample (Supplementary Table S2). The average number of the clean reads after trimming was 1.1 billion sequence reads. The average unique mapping rate (93.93%) and the average overall mapping rate (97.81%) were determined. The MDS results based on transcriptomes (lncRNA and mRNA) showed that the clusters clearly separated for each tissue were common in lncRNAs and mRNAs (Supplementary Fig. S1).
After 627,357 transcripts were obtained through assembly and merging, 626,016 transcripts remained except for those less than 200 bp in length. After the class codes (i, j, o, u, x) of the candidate lncRNA were extracted, 393,933 transcripts remained; of these transcripts, 94,727 had an ORF length of less than 300 bp. Then, 70,929 transcripts were derived using four coding potential assessment tools and two databases; finally, 19,021 novel lncRNA transcripts, excluding the transcripts identified in only one sample (Fig. 2A, Supplementary Table S3), were obtained.
Various types of information about the predicted lncRNA were first investigated and then compared with those of mRNA to determine the genomic characteristics of the acquired novel lncRNA. The average exon number of novel lncRNAs was 2.4, the known lncRNAs was 2.8, and the protein-coding mRNA was 12.3, indicating that lncRNAs tended to be less than mRNAs (Fig. 2B). The mean transcript length of the novel ncRNA was about 18,763 bp, the known lncRNA was about 22,846 bp, and the protein-coding mRNA was about 56,276 bp. The transcript length also showed that lncRNAs tend to be shorter than mRNAs (Fig. 2C), which were consistent with previous studies [39,40]. In addition, the transcript location of the novel lncRNA was classified as follows: 31.3% of intergenic regions that did not overlap with the protein-coding gene, 17.3% of intronic, 28.5% of sense exonic, 17.8% of antisense exonic, and 5.1% bidirectional (Fig. 2D). Finally, the TSI was calculated to determine the tissue specificity of lncRNAs. Similar to previous results [29], the average TSI (0.73) of lncRNAs was higher than the average TSI (0.64) of mRNAs (Fig. 2E).
The differential expression levels of lncRNAs and mRNAs in each of the three reproductive tissues (ovary, oviduct, and endometrium) were investigated at six time points (D03, D06, D09, D12, D15, and D18) based on the estrous cycle D00 (Fig. 1). The patterns of changes in the upregulated and downregulated expression levels of lncRNA and mRNA for each tissue were similar (Supplementary Fig. S2). The number of DE transcripts in the ovary gradually decreased until D18 after many differential expression levels were observed on D06 and D09. The number of DE transcripts in the endometrium also increased until D09 and then gradually decreased. In the oviduct, DE transcripts increased until D12 and decreased thereafter; the smallest number of DE transcripts was also found in the oviduct (Supplementary Fig. S3). In addition, the number of overlapping DE lncRNAs in the three tissues at each time point was relatively smaller than that of DE mRNA (Supplementary Fig. S4). The list and significant values of all DE lncRNAs and mRNAs are summarized in Supplementary Table S4.
Six modules (networks) of lncRNAs that correlated with hormones, reproductive tissues, and time points were calculated (Supplementary Fig. S5). Among them, four modules (blue, green, red, and yellow) were set to represent high significance with traits. Blue, green, and red modules were highly correlated in each of the three tissues (ovary, oviduct, and endometrium), and the yellow module was correlated with changes in each hormone (Fig. 3A). The blue, green, red, and yellow modules had 3,243, 2,725, 2,682, and 24 lncRNAs, respectively, and four co-expression networks were generated using the WGCNA from these four modules. The blue network, which showed an ovary tissue-specific correlation, consisted of 130 nodes (lncRNAs) and 234 edges (interactions); it also had the largest number of nodes (Fig. 3B). The green network, which showed oviduct tissue-specific correlation, comprised 45 nodes and 181 edges (Fig. 3C). The red network, which exhibited endometrium tissue-specific correlation, consisted of 93 nodes 265 edges (Fig. 3D). The yellow network, which indicated the correlation with hormones, had 20 nodes 30 edges (Fig. 3E). Among the lncRNAs constituting the network, DE lncRNA had the highest number of 70 in the blue network and the smallest number of 8 in the green network; furthermore, 27 and 16 DE lncRNAs were found in the red and yellow networks, respectively.
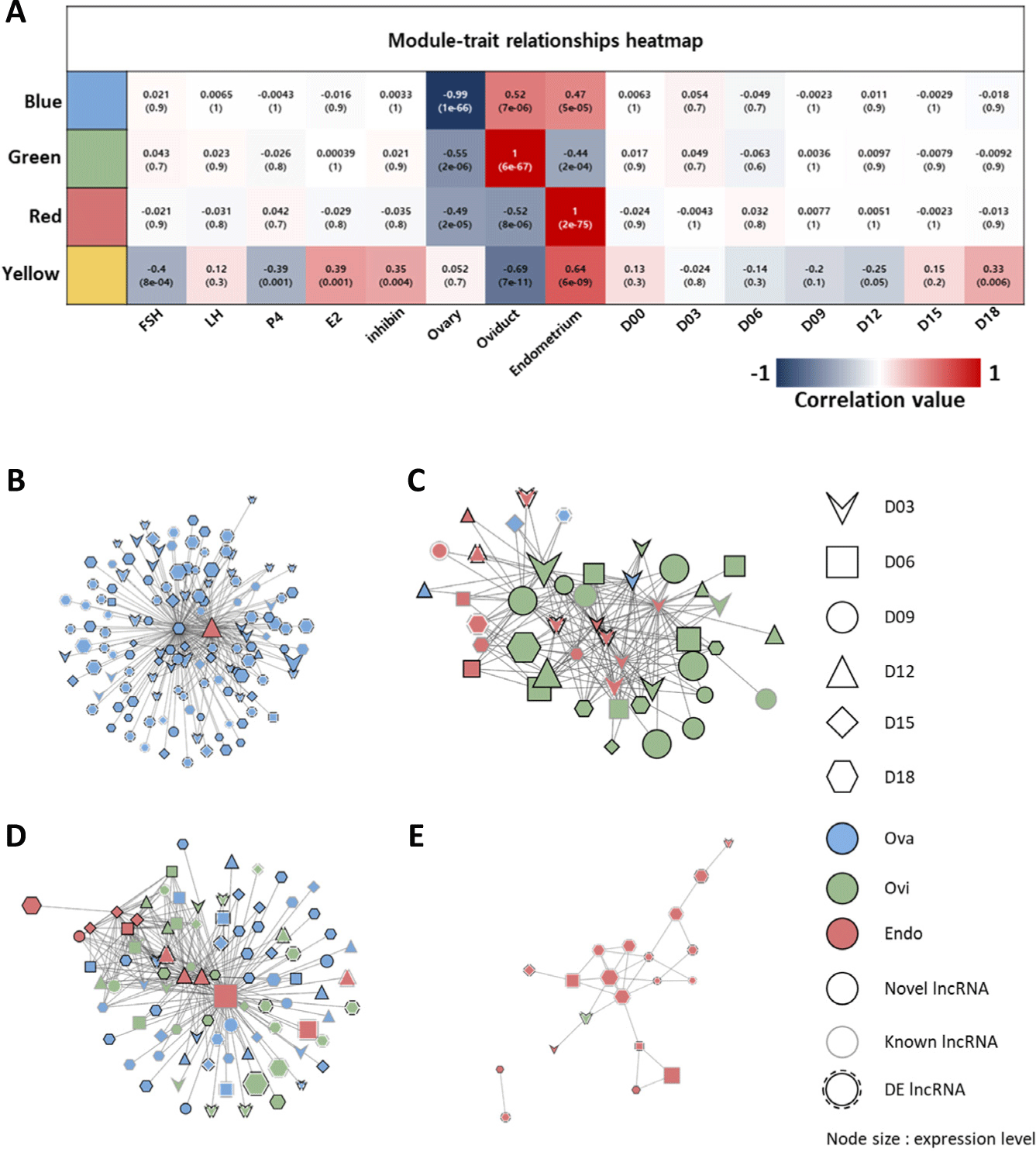
The cis-acting gene of each lncRNA was searched to identify the coding genes regulated by the main lncRNAs in the network [37]. These lncRNAs and cis-acting genes were distributed in all chromosomes except chromosome 7, and relatively many cis-acting genes were found in the blue and red networks in proportion to the number of lncRNAs (Fig. 4). Furthermore, 240 cis-acting genes were identified from 130 lncRNAs in the blue network, 152 cis-acting genes were identified from 45 lncRNAs in the green network, 262 cis-acting genes were identified from 93 lncRNAs in the red network, and 48 cis-acting genes were identified from 20 lncRNAs in the yellow network, which were visualized in the outer layer. Among the cis-acting genes searched in this way, 105 in blue networks, 56 in green networks, 112 in red networks, and 18 in yellow networks were DE, and they were shown in the middle layer of the cis-acting gene category. Among them, the DE cis-acting genes of DE lncRNAs in each network were found in the inner layer, with 48 blue networks, 13 green networks, 31 red networks, and 14 yellow networks. The cis interactions between them and the main DElncRNA were visualized as a line in the innermost circle. A list of lncRNAs and cis-acting genes is presented in Supplementary Table S5.
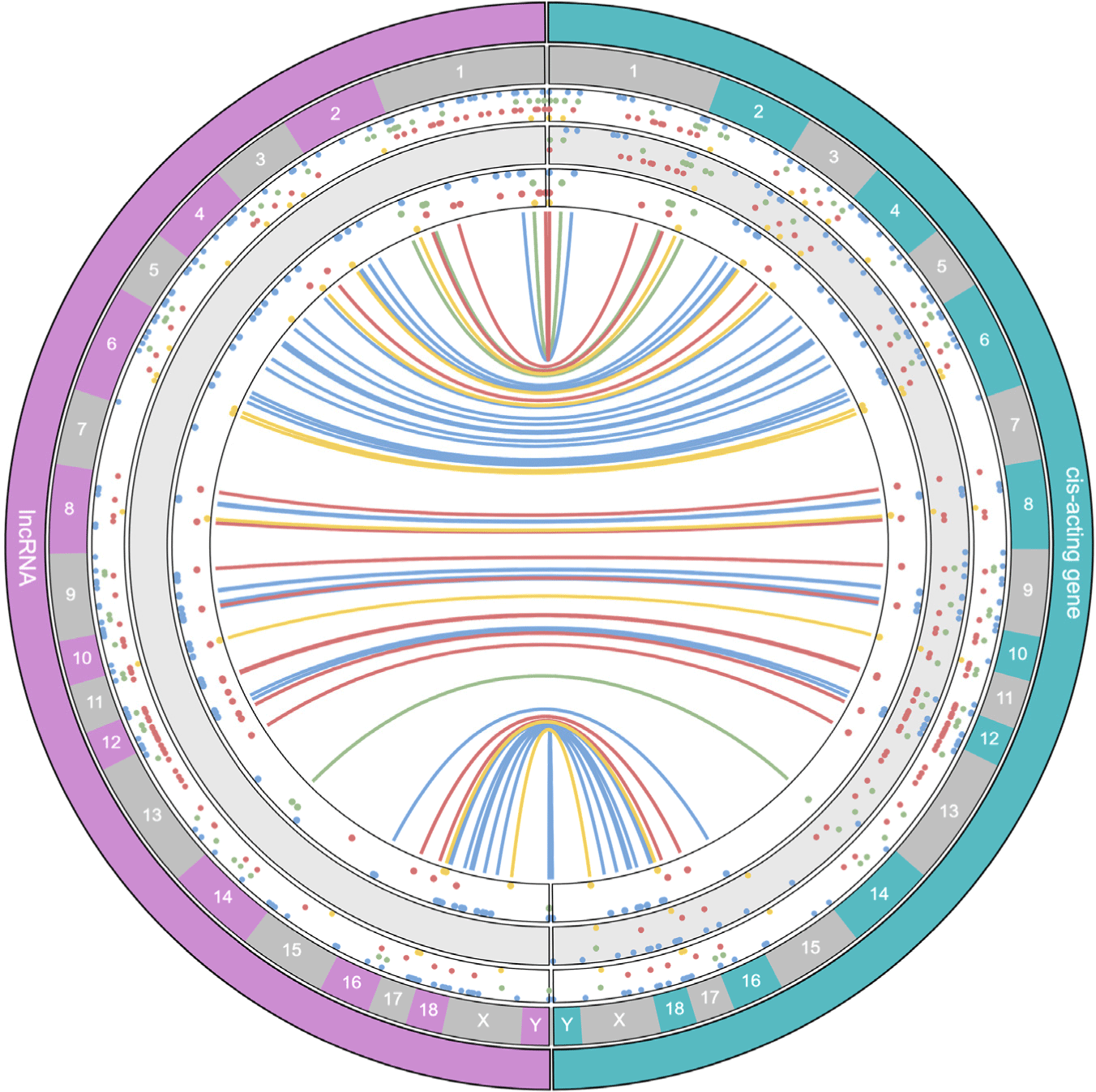
Naturally, lncRNAs with a cis-acting mechanism have low expression levels, and they have the potential to regulate mRNAs even with such a low expression [37]. Therefore, functional analysis was performed with the genes in the middle layer of the cis-acting gene category in Fig. 4. First, the expression patterns of DE cis-acting genes and lncRNAs interacting with one another were compared. In each of the three tissue-specific networks, two expression patterns were determined: a cluster in which the expression patterns of the two groups of genes were directly proportional and another cluster in which the expression patterns of the two groups of genes were inversely proportional to the ovarian tissue (Supplementary Figs. S6A, S6B, and S6C). In addition, in the hormone-specific network, the two gene groups were distinguished with two expression patterns: a cluster that was directly proportional to the whole tissue and a cluster that was inversely proportional to the whole tissue (Supplementary Fig. S6D).
From the DE cis-acting genes corresponding to the blue module network, the KEGG pathways (neuroactive ligand-receptor interaction, ubiquitin mediated proteolysis, etc.) and BP terms (regulation of ion transmembrane transport, protein polyubiquitination, etc.) were enriched (Fig. 5A). From the DE cis-acting genes corresponding to the green module network, the KEGG pathways (tight junction, thyroid hormone synthesis, etc.) and BP terms (positive regulation of MAP kinase activity, positive regulation of cell migration, epithelial cell morphogenesis, toxin transport, etc.) were enriched (Fig. 5B). From the DEcis-acting genes corresponding to the red module network, the KEGG pathway (metabolic pathways) and BP terms (vitamin A metabolic process, retinoic acid biosynthesis, N-terminal protein amino acid acetylation, T cell costimulation, etc.) were enriched (Fig. 5C). From the DE cis-acting genes corresponding to the yellow module network, BP terms (skin development, cellular-modified amnio acid catabolic process, organic acid catabolic process, etc.) were enriched (Fig. 5D). The detailed information on enriched genes and their significant values are summarized in Supplementary Table S6.
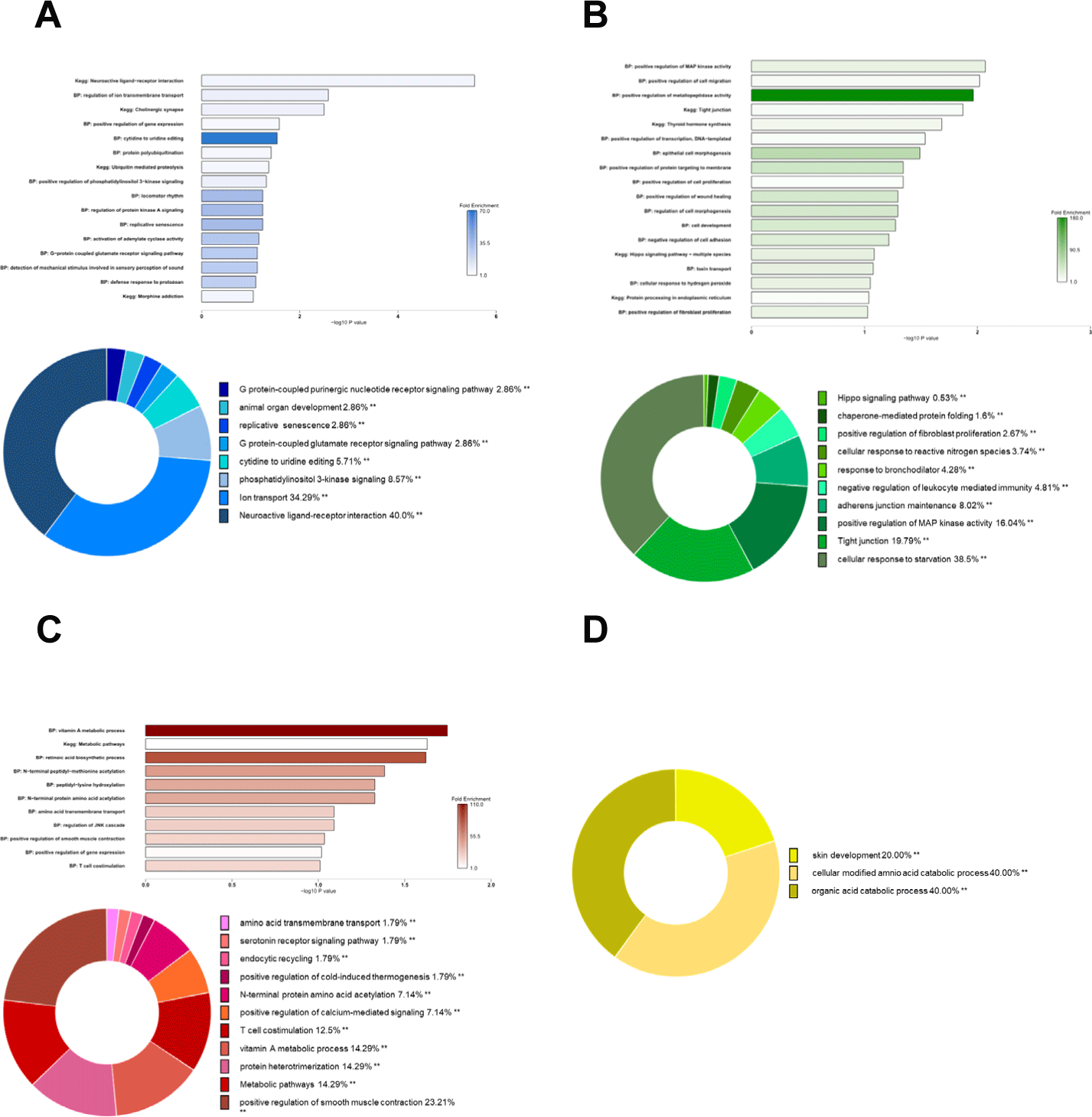
FSHR, KCND2, and KCNQ5 were the main cis-acing genes involved in functional analysis of the lncRNAs of the blue module network, and the lncRNAs with a cis-acting relationship were identified to be XLOC_021792, XLOC_017111, ENSSSCG00000050977, XLOC_000342, and ENSSSCG00000050380 (Fig. 6A). The main cis-acing genes involved in the functional analysis of the genes of the green module network were ERBB2 and DNM1; ENSSSCG00000045111, XLOC_003255, XLOC_003256, and XLOC_003257 were the lncRNAs having a cis-acting relationship with them (Fig. 6B). RBP1, NMNAT3, and DLX5 were the main cis-acing genes involved in the functional analysis of the genes of the red module network, and XLOC_008338, XLOC_037548, and ENSSSCG00000051124 were the lncRNAs showing a cis-acting relationship with them (Fig. 6C). KLF6, KLF11, and MYBL2 were the main cis-acing genes involved in the functional analysis of the genes of the yellow module network; XLOC_004128, ENSSSCG00000040267, and XLOC_016616 were the lncRNAs having a cis-acting relationship with them (Fig. 6D).
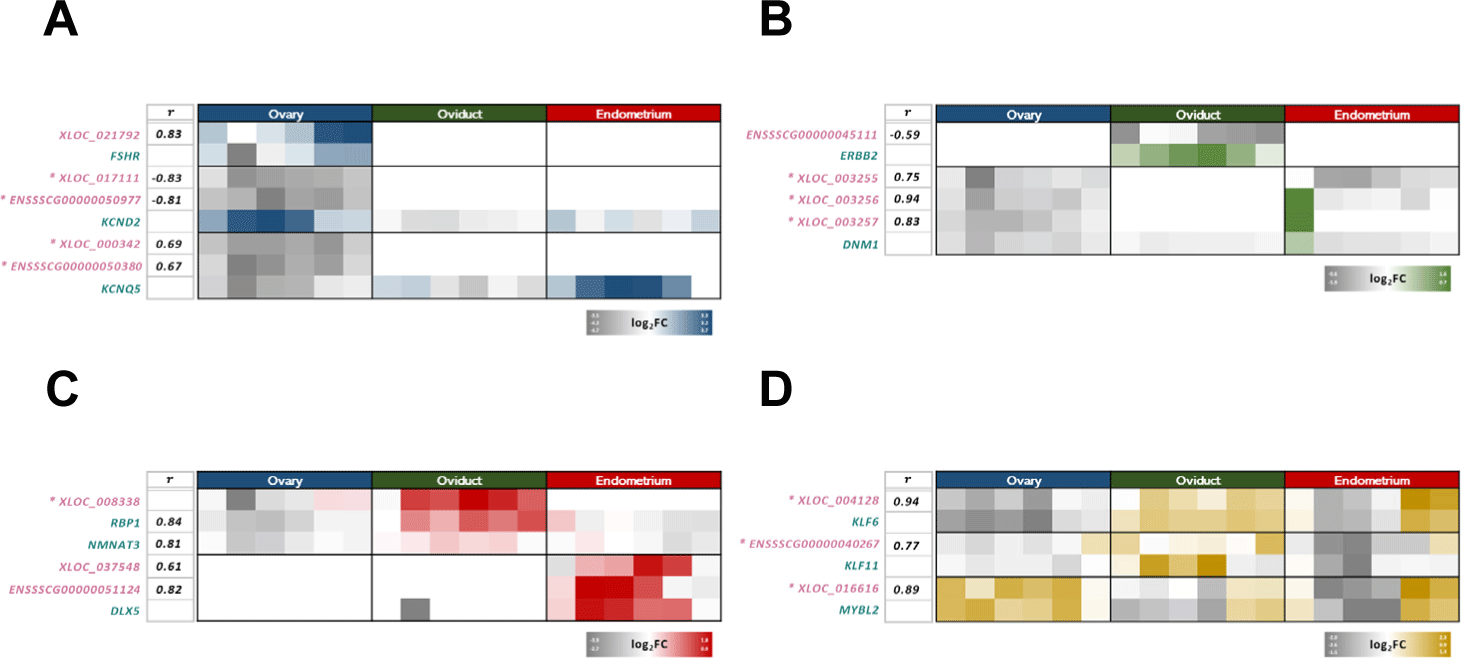
DISCUSSION
Swine reproductive tissues undergo various tissue-integrated and tissue-specific changes during the estrous cycle. During the proestrus period (D16–D21), FSH is secreted by GnRH in preparation for estrus, and estrogen secretion increases as the follicle matures for ovulation [9]. At this time, E2, an estrogen steroid hormone, thickens the lining of the uterus to create an environment where a follicle can be implanted when the follicle is fertilized [41]. After the peak of E2 and FSH secretion in the estrus period (D00–D03), fully mature follicles become ovulated [9]. In the metestrus period (D04–D06), luteinization begins, P4 secretion begins, and the follicle from the oviduct is released [17]. In diestrus (D07–D15), which is the longest phase of the estrus cycle, the corpus luteum continues to develop in the ovary, and P4 secretion increases continuously [9]. P4 helps keep the endometrium fertile and stimulates PGF2α secretion by the endometrium [42–44]. If pregnancy is not achieved until approximately D15, PGF2α is secreted from the endometrium to the ovary, and the corpus luteum is degenerated to reduce P4 secretion, contract the uterus, and return to the proestrus state [45,46]. With these changes, the mRNA expression undergoes tissue-integrated and tissue-specific changes during the estrous cycle [6]. The lncRNA expression also changes, and its expression pattern is similar to that of mRNA (Supplementary Figs. S2 and S3). This finding suggests that mRNAs and lncRNAs are involved in the changes in three reproductive tissues during the estrous cycle. In this study, WGCNA analysis was performed to determine the correlation between these lncRNAs. Among the modules constructed through lncRNA co-expression analysis, the tissue-specific lncRNAs of three reproductive tissues during the estrous cycle were investigated using three modules, and tissue-integrated lncRNAs were examined by identifying hormonal changes in the three tissues using one module. These generated modules revealed the existence of lncRNAs specifically regulated by tissue or hormonal changes.
First, the tissue-specific lncRNAs of the ovary were obtained from the network constructed through the blue module (Fig. 3B). BP terms and KEGG pathway enrichment analyses were performed to reveal the association between the molecular function of the corresponding DE cis-acting gene of lncRNAs and the estrous cycle. Numerous main terms essential for ovarian development and function were identified (Fig. 5A).
Among the lncRNA and cis-acting gene pairs involved in these functions, five pairs had significant expression patterns and correlations (Fig. 6A). Previous studies confirmed that the neuroactive ligand-receptor interaction pathway may play an important role in follicle production and ovarian function regulation in poultry and fish; it may also influence steroid hormone synthesis in the gonads [47]. FSHR is highly expressed in ovarian granulosa cells; it is a key gene involved in ovarian function and stimulated primarily by FSH to regulate follicle growth and maturation, as well as estrogen production [48]. In our study, FSHR and XLOC_021792 were highly expressed when FSH was the most secreted for ovulation (D16–D03); therefore, a novel lncRNA, namely, XLOC_021792, could positively regulate FSHR through a cis-acting relationship (Fig. 6A). In addition, ovarian follicles have a characteristic pattern of bioelectrical activity and ion transport mechanisms; they are also involved in a range of oogenesis through Na+, K+, and Cl− channel activation and ion transport [49,50]. Among the K+ genes, KCND2, which acts as a steroid sensor and generates bioelectrical signals for follicle production, and KCNQ5 from the KCNQ family, which is an important substance in ion channels in hamster ovarian cells, were identified in our sample [51,52]. Our results showed that KCND2could be negatively regulated as a cis-acting relationship from novel lncRNA XLOC_017111 and known lncRNA ENSSSCG00000050977; KCNQ5 was positively regulated as a cis-acting relationship from novel lncRNA XLOC_000342 and the known lncRNA ENSSSCG00000050380 (Fig. 6A). Since the period of the high KNCD2 expression (D7–D15), which acts as a steroid sensor, was very similar to the period of progesterone secretion in the corpus luteum, XLOC_017111 and ENSSSCG00000050977, which were negatively regulated in the cis-acting relationship, would affect progesterone secretion.
Tissue-specific lncRNAs of the oviduct were obtained from the network constructed through the green module (Fig. 3C). BP terms and KEGG pathways related to cell morphogenesis and cell development in oviductal tissues were identified from the DE cis-acting genes of these lncRNAs (Fig. 5B). Among the lncRNA and cis-acting gene pairs involved in these functions, four pairs had significant expression patterns and correlations (Fig. 6B). In the porcine oviductal tissue, MAP kinase activity stimulates gene expression and cell division; it also favors the formation of epithelial cells of the fallopian tubes and prevents the entry of toxic materials or pathogens by controlling permeability via tight junctions [53–55]. ERBB2, which is involved in both of these key terms and in epithelial cell development and signaling [56], is negatively regulated in a cis-acting relationship by ENSSSCG00000045111, a known lncRNA (Fig. 6B). In the period of high ERBB2 expression (D04–D15), the follicle moves to the uterus through the oviduct after ovulation to prepare for pregnancy. ENSSSCG00000045111 was likely involved in the formation of tubal epithelial tissue during this period.
The tissue-specific lncRNAs of the endometrium were obtained from the network constructed through the red module (Fig. 3D). The main terms related to uterine development for embryo implantation were identified from the DE cis-acting genes of these lncRNAs (Fig. 5C). Among the lncRNA and cis-acting gene pairs involved in these functions, four pairs had significant expression patterns and correlations (Fig. 6C). Retinol (vitamin A) and retinol-binding protein (RBP) secreted into the endometrium were implicated in the development of the porcine endometrium and embryonic formation. These RBPs participate in the storage, metabolism, and transport of retinol to target cells and in uterine growth and morphogenesis. RBP has four morphological categories that affect the endometrium in various ways [57–59]. In our study, among RBPs, RBP1 was positively correlated with XLOC_008338, a novel lncRNA; in comparison with other tissues, when XLOC_008338 was not expressed in the endometrium, the expression of RBP1 was also suppressed (Fig. 6C). The expression of RBP1 possibly increased for implantation at the time of ovulation (D03), but the expression of RBP1 likely decreased because implantation did not occur (D4–D21). At this time, NMNAT3 had a high positive correlation in the cis-acting relationship such as RBP1 and XLOC_008338, and it was affected by XLOC_008338. NMNAT3 likely participated in endometrial morphogenesis.
Tissue-integrated lncRNAs were obtained from the yellow module constructed according to the correlation of hormone changes (Fig. 3E). The genes correlated in the cis-acting relationship with these lncRNAs in all tissues and estrous cycles were CHST9, DECR2, KLF6, KLF11, MYBL2, and PCDH19 (Fig. 6D, Supplementary Fig. S7). The KLF family can be expressed in tissues that respond to steroid hormones in relation to the action of the progesterone receptor (PGR) and estrogen receptor (ESR) [60]. In the present study, KLF6 and KLF11 were highly expressed when PGF2α was secreted for ovarian P4 inhibition in the endometrium (D16–D21); thus, P4 was inhibited and E2 was highly expressed in th eovary during secretion. KLF6 was highly correlated with novel lncRNA XLOC_004128, and KLF11 was highly correlated with known lncRNA ENSSSCG00000040267; This high correlation seemed to be affected by the cis-acting relationship with lncRNAs (Fig. 6D). Therefore, these lncRNAs were likely closely related to the secretion of PGF2α from the endometrium and the secretion of E2 from the ovary. MYBL2 showed a similar expression pattern in the endometrium and an opposite expression pattern in the ovary. XLOC_016616 also showed an expression pattern similar to that of MYBL2, and this expression pattern was possibly regulated in the cis-relationship (Fig. 6D). Therefore, XLOC_016616 could be a lncRNA that could affect the secretion of PGF2α from the endometrium and the secretion of P4 from the ovary.
The trans-acting relationship between lncRNA and coding gene is as important and diverse as the cis-acting relationship although it is difficult to verify compared with the cis-acting relationship and vague in setting the standard [37]. Usually, the relationship between a lncRNA and a trans-acting gene means that they interact even when they are in different chromosomes [61]. In the present study, the core lncRNA was selected through co-expression network construction (Figs. 3B and 3D). Core lncRNAs were highly correlated with the other main lncRNAs in the network present in different chromosomes (Supplementary Fig. S8). Two core lncRNAs, namely, XLOC_000748 and ENSSSCG00000051124, are presented as trans-acting lncRNAs. In addition, a coding gene in a cis-acting relationship could be affected not only by one lncRNA but also by several lncRNAs. Conversely, one lncRNA may affect multiple genes in a cis-acting relationship [37]. In the present study, XLOC_003255, XLOC_008338, XLOC_037548, and other lncRNAs had this potential (Figs. 6A, 6B and 6C). The principle that lncRNA regulates a gene as a trans-acting factor and various transcriptional regulation methods of lncRNA are complex and diverse. Consequently, a more complete reference, including the results of this study, should be established to accurately determine how lncRNA plays these roles, and in-depth research based on structural analysis should be performed.
CONCLUSION
In this study, lncRNA (novel lncRNA and known lncRNA) and mRNA expressed during the swine estrous cycle were discovered in three reproductive tissues of pigs, and their expression patterns were similar. Network analysis was performed to analyze the function of lncRNA according to its expression pattern, and cis-acting genes were searched to understand the interaction between lncRNA and mRNA. The main lncRNA and cis-acting gene significantly expressed according to the estrous cycle in a tissue-specific or tissue-integrated manner were found, and their functions were presented. The expression of these lncRNA and cis-acitng gene could be highly related to hormonal activities secreted by each tissue. In the ovary, XLOC_021792 likely regulated FSH secretion during the follicular phase; XLOC_01711, ENSSSCG00000050977, XLOC_000342, and ENSSSCG00000050380 possibly regulated P4 secretion during the luteal phase. ENSSSCG00000045111 controlled the oviductal morphogenesis for pregnancy preparation during the luteal phase, and XLOC_008338 modulated the uterine morphogenesis in the estrus phase. XLOC_004128 and ENSSSCG00000040267 likely inhibited P4 secretion and activated E2 secretion in the ovary during the luteal phase. They were also related to the secretion of PGF2α from the endometrium during the proestrus period. XLOC_016616 was also presented to be a similar lncRNA. These results revealed the possible lncRNAs and their functions that have not been identified in porcine reproductive tissues according to the estrus cycle. Furthermore, this study could help elucidate the activities and interactions of reproductive tissues that were difficult to understand only when mRNA was used as a reference. This study also provided a basis for further research on the specific mechanism by which lncRNA regulates mRNA according to the structure and location of a given lncRNA sequence.