INTRODUCTION
Accurate animal identification applies to individual management and the entire process of livestock food production; hence, it is essential for establishing a traceability system for the food supply chain from farm to table [1,2]. Reliable animal identification methodologies monitor each stage of growth steps and production while minimizing trade losses and ensuring animal ownership. To implement such a tracking system, a robust identification methodology is required [3] because the failure of the tracking system can cause enormous damage. The damage is linked to cow health and food safety, which can put the health of customers at risk and cause serious economic problems [4].
To eliminate these potential hazards, ear notching, tattoos, tags, and branding are some of the traditional permanent methods used for animal identification. However, these can be easily duplicated, simplifying theft and fraud [5]. Radio frequency identification (RFID) tags have been developed as an alternative to traditional methods [6]. Through RFID, animals are registered in computer systems and can be identified by scanning the RFID tag. However, the tag is invasive and can be changed by manipulating it in the system, creating an avenue for fraud [7]. Recently, biometrics such as retinal vascular patterns (RVPs) [8], muzzle [9,10], and iris [11,12] have been proposed to resolve the problems of RFIDs. Methods that utilize these biometrics are reliable for identifying an entity because they are the most accurate and stable biometric modalities during the lifetime of an animal [3,13].
With the advent of deep neural networks (DNNs) [14], there have been several attempts to identify anatomical parts of an animal using deep learning technologies [10,15–17]. Among the deep learning technologies, the segmentation technique classifies objects within a given image in a pixel-wise manner. As segmentation of the iris from the image of an eye is essential for initiating iris identification, using an elaborate and accurate segmentation technique is key to successful iris recognition [16].
In this study, we discuss bovine iris segmentation using a novel framework. The framework develops multiple segmentation models by training on publicly available bovine iris datasets, BovineAAEyes80 [18] and comparing combinations of state-of-the-art deep learning techniques. Since iris datasets are rare and have limited formats, like other biometric datasets, we propose a framework that can be used to develop models using the smallest input datasets: region of interests (ROIs) labels and RGB images. This study contributes to the advancement of iris identification using DNNs and the development of a reliable DNN training framework that assists in identifying the most suitable combination of DNN models for biometric images.
MATERIALS AND METHODS
The proposed framework starts with data collection. The input data must contain pairs of image and annotation data (Fig. 1A). After collecting the data pairs, the data is prepared, which includes data splitting and augmentation selection. Data must be split into training, validation, and test datasets that are preferably mutually exclusive for each of the training, validation, and testing stages to be conducted with unseen data. The data must be split such that it is equally distributed in terms of quality since this step can affect the result of the trained model [19]. The augmentation selection step can be varied according to the traits of the dataset (Fig. 1B). After selecting the augmentation options, we developed 15 combinations of DNN models by utilizing three different encoder backbones, namely VGG16 [20], ResNet50 [21], and MobileNet [22]. Additionally, we employed five segmentation decoder DNNs, namely FCN8, FCN16, FCN32 [23], U-Net [24], and SegNet [25]. The encoder and decoder form an architecture known as an encoder-decoder network, which is widely used for tasks such as image segmentation. The encoder extracts useful features and compresses the input data, while the decoder reconstructs or segments the data based on the encoded representation. This architecture enables the network to learn and leverage hierarchical and contextual information, leading to more accurate segmentation results. These combinations allowed us to explore a range of model architectures and evaluate their performance. In total, we trained and evaluated 75 models (15 combinations × 5-fold cross-validation) to ensure the reliability of the training results (Table 1). The evaluation process included assessing various metrics such as accuracy, precision, recall, intersection over union (IoU), and dice coefficient [26]. Furthermore, the framework provided detailed information such as inference time on each model, along with graphical representations of the segmentation results (Fig. 1D).
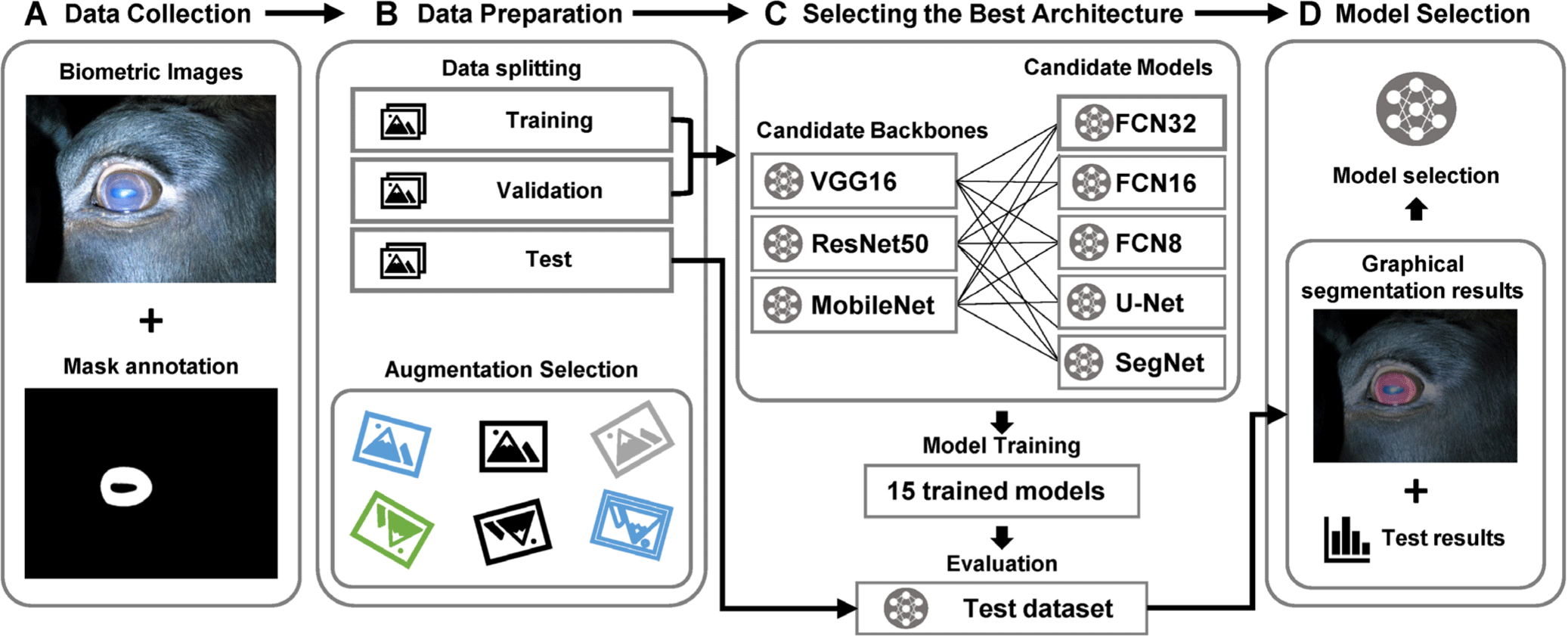
Dataset | Fold | Images | Eye ID |
---|---|---|---|
Train | Fold 1 | 12 | 3, 7 |
Fold 2 | 13 | 4 | |
Fold 3 | 13 | 5, 6 | |
Fold 4 | 12 | 8, 9 | |
Fold 5 | 22 | 10, 11 | |
Test | - | 8 | 1, 2 |
Total | 80 | 11 eyes |
With reference to previous studies, we compared five candidates, FCN32, FCN16, FCN8, U-Net, and SegNet, to find the most reliable architecture for anatomical segmentation (Fig. 1C). All configurations were set to be equal for a fair comparison, minimizing variants between model training processes. After several attempts, the training hyperparameters were experimentally determined: training for 100 epochs with 128 steps per epoch, a learning rate of 0.001 optimized using an Adam optimizer, and a batch size of 4. These trained models automatically generated anatomical ROIs from input test images. After training and evaluation with statistical performance measures, such as the dice coefficient and accuracy [27], the statistical result is returned in the csv format and analyzed within the framework system.
Model training was conducted on Anaconda 4.10.1 running on 64bit Ubuntu Linux 20.04.3 LTS and Python v3.8.8. TensorFlow-GPU v2.7.0 and CUDA 11.4 were used to accelerate the DNNs framework’s training process on a 24 GB RTX 3090 graphics card, and Keras v2.7.0 was used as a Python deep learning application programming interface (API). In the BovineAAEyes80 dataset, brightness ±10 and rotation ± 40° augmentation are applied to cover variations that could arise in from the capturing environment, such as non-cooperative behavior of bovines and changes in lighting conditions [18].
The classification performance of the trained model was evaluated using the following metrics: accuracy (1), recall (2), precision (3), IoU (4), and dice coefficient (5) [27]. Compared to the reference annotation, each pixel is classified into one of four outcomes: true positive (TP), true negative (TN), false positive (FP), and false negative (FN); these classifications are according to the metric criteria of a previous study [28].
RESULTS AND DISCUSSION
The learning curves of model training, as shown in Fig. 2, provide important insights into the performance and stability of different models during the training process. In the training curve of VGG16, as seen in Figs. 2A and 2B, all of the FCN series are observed to be unstable during the training process. Additionally, FCN32 is found to have the highest loss and the lowest accuracy, indicating that it is not the best model for this particular task. On the other hand, SegNet and U-Net demonstrate a comparatively stable decrease in loss and increase in accuracy during most of the training process. In the training curves of ResNet50, as depicted in Figs. 2C and 2D, and MobileNet, as seen in Figs. 2E and 2F, decent accuracies and losses with little fluctuation, compared with VGG16, are observed. In contrast to FCN32, which has the poorest performance among the models, the other models show promising results.
Table 2 shows the test results of the models trained with an unseen test dataset. In Table 2, U-Net with a MobileNet backbone has the best dice coefficient (98.35 ± 0.54%), accuracy (99.50 ± 0.16%), and precision (99.57 ± 0.16%). U-Net with a VGG16 backbone shows the best IoU score (96.81 ± 2.01%), which is slightly (0.01%) better than that of U-Net with a MobileNet backbone.
Table 3 presents the inference times of different decoder and encoder models for a given task. While MobileNet is generally observed to perform the fastest across most of the decoder models, the performance of different decoder and encoder model combinations can be influenced by a range of factors beyond the choice of encoder architecture alone. For instance, when paired with FCN8 and FCN16, MobileNet has processing times that are slower than those of VGG16 and ResNet50. Specifically, when paired with FCN8, the mean processing times are 133.3 ± 1.0 ms for VGG16, 180.1 ± 1.6 ms for ResNet50, and 156.8 ± 7.1 ms for MobileNet. When paired with FCN16, the mean processing times are 131.6 ± 0.6 ms for VGG16, 182.5 ± 1.3 ms for ResNet50, and 136.7 ± 1.5 ms for MobileNet. Likewise, while MobileNet performs well when paired with FCN32, it is outperformed by VGG16 when paired with FCN8 and FCN16. However, when MobileNet is paired with SegNet and U-Net, it shows the fastest inference speed recording 122.8 ± 3.2 ms and 116.3 ± 2.5 ms respectively.
These findings suggest that the performance of different decoder and encoder model combinations can be influenced by a range of factors beyond the general performance of encoder architecture alone. The characteristics and complexity of the dataset, as well as the specifics of the task at hand, can all impact the performance of the model. Therefore, it is important to carefully consider the selection of both the decoder and encoder architectures when developing deep learning models for image segmentation tasks. Overall, Table 3 provides useful information on the performance of different decoder and encoder model combinations for the given task, with certain models performing significantly faster or slower than others. The information on processing times can be used to select the optimal model combination based on the trade-off between processing speed not only segmentation accuracy.
Based on the results of our study, the U-Net model with a MobileNet backbone can be considered the most appropriate model for the given dataset. However, it is important to note that there are significant variations in the size of a segmentation unit of pixels between different backbones, which is influenced by the extracted feature map size of each encoder architecture. Therefore, when selecting a model, both numerical scores and pixel segmentation size should be taken into account, as the optimal DNN model can vary depending on the application domain.
In the context of iris segmentation, where the fine segmentation of the edge of the iris boundaries is a targeted objective, the model with the second-best score, U-Net with a VGG16 backbone, was chosen as the best model due to its superior dense boundary segmentation. The decision to select this model was based on the median values of the dice coefficient observed in the results of the 5-fold cross-validation.
Overall, while the U-Net model with a MobileNet backbone is the most suitable for the given dataset, the U-Net model with a VGG16 backbone was deemed the optimal model for iris segmentation due to its superior boundary segmentation. The selection of the best model for a given task requires careful consideration of both numerical scores and pixel segmentation size, as well as the specific objectives of the application domain (Fig. 3).
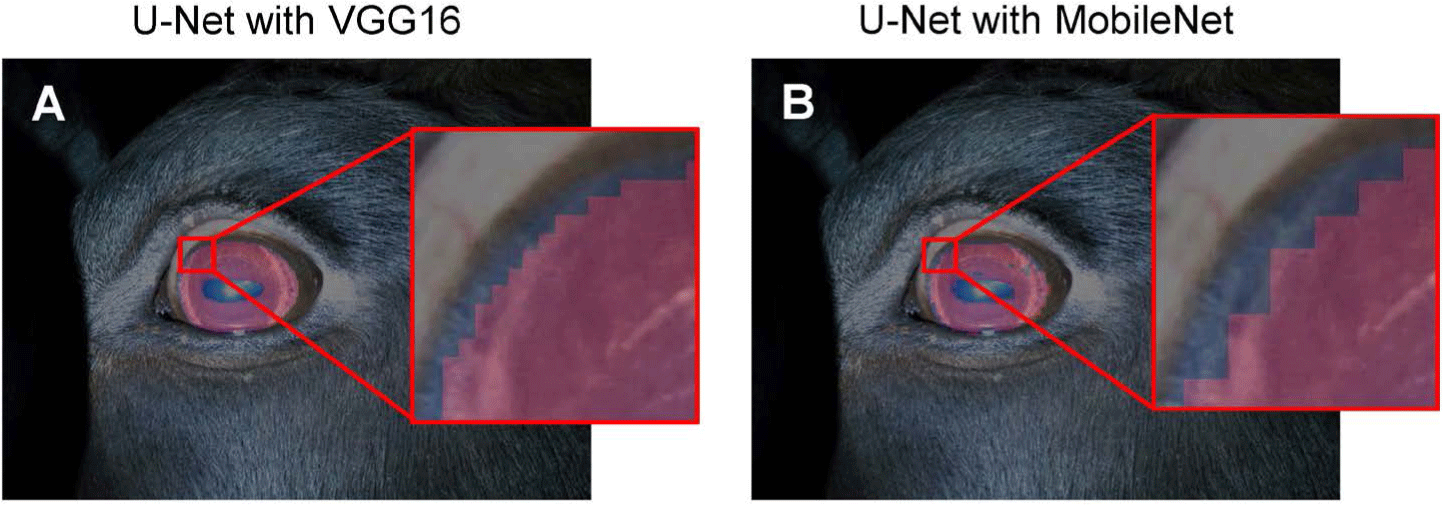
In Fig. 4, common corruptions in iris images are described. Minor corruptions, which distort the iris image, can be caused by many factors, such as dust in the spots, stains over the lens, the animal’s eyelash or fur in the eye (Fig. 4A), and unwanted light spots [16]. These minor corruptions were not reflected in the segmentation result (Fig. 4C). However, this issue must be resolved to eliminate false information within the iris image. Major corruption is generally caused by relatively large parts of the animal’s body, such as the occlusion of eyelashes and eyelids (Fig. 4B). As mentioned in other studies, these major corruptions can impede accurate identification [12, 18, 29]. However, the best selected model accurately segmented the corrupted image by excluding the occlusion (Fig. 4D). This could have not been calculated correctly in the result because the annotation labels used in the model training did not provide much pixel-wise accurate segmentation ground truth. This is remarkable compared with other studies using image processing techniques because it segmented the exact iris area without preprocessing or postprocessing with the model’s knowledge, even though image corruption was not annotated in the given labels.
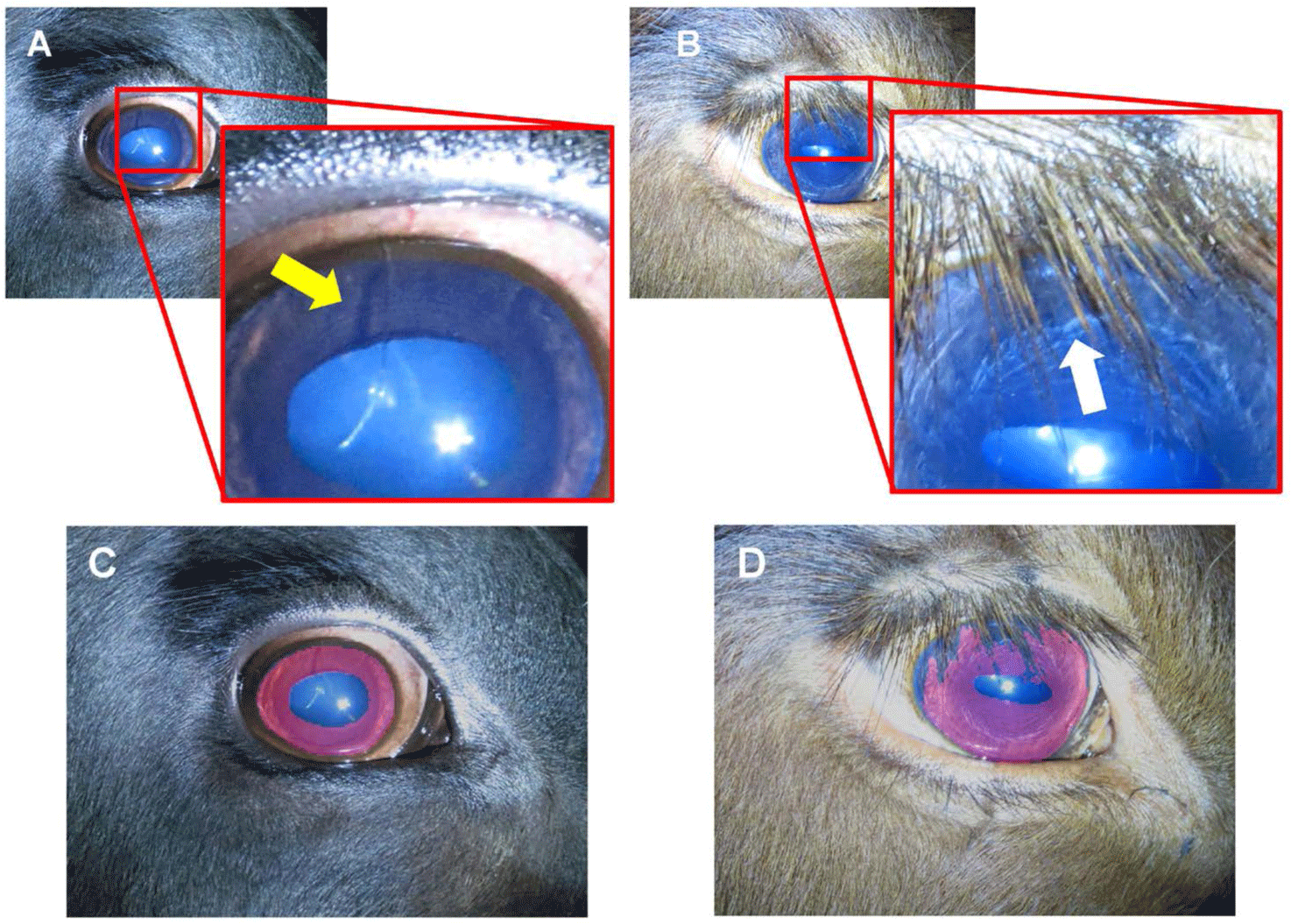
The field of deep learning is rapidly evolving, with new and improved models being developed all the time [26,30,31]. Therefore, it is possible that even better-performing segmentation encoder and feature map decoder models may become available in the future. The current study used a limited set of models, which may not represent the best possible models for bovine iris segmentation. However, the proposed deep learning framework provides a foundation for future research to incorporate and evaluate additional models. This could lead to further improvements in the accuracy and efficiency of the segmentation process.
In addition, the present study focused on bovine iris segmentation using a limited dataset. Future research could expand the framework to include other animal species and biometric features. This would increase the framework’s versatility and applicability to various animal biometric applications.
Overall, while the proposed framework has limitations, it serves as a starting point for future research to incorporate additional models and further optimize the segmentation process. As the field of deep learning continues to advance, it holds great promise for improving animal identification and traceability systems.
The deep learning framework proposed in this study for bovine iris segmentation has potential applications in animal identification and traceability systems, which are crucial for ensuring food safety, quality, and individual management system. The framework could be used in various animal biometric applications, such as identifying individual animals in large herds, monitoring animal health, and tracking animal movements.
In addition, the proposed framework could have implications for improving the efficiency and accuracy of livestock management practices. By enabling reliable and rapid animal identification, the framework could help reduce labor costs and improve animal welfare. Furthermore, the framework’s use of deep learning technology could lead to new insights into animal biometrics and behavior, which could inform the development of more effective management strategies. Moreover, the proposed framework’s reliance on deep learning technology could also exacerbate existing biases and inequalities in animal identification and traceability systems. Careful consideration must be given to how the framework’s use of biometric data might disproportionately affect certain animal populations or communities. Nevertheless, despite these concerns, it is deemed necessary to conduct in-depth further research as this technology can still contribute to the national animal population management system, livestock distribution industry, and livestock quality assessment.
In summary, the proposed deep learning framework for bovine iris segmentation has the potential to improve animal identification and traceability systems, and to enhance the efficiency and accuracy of livestock management practices. However, its use must be guided by ethical principles and considerations to prevent potential harms and biases.
CONCLUSION
With the proposed framework, iris segmentation for identifying animal biometrics was performed utilizing the information in the trained DNNs along with robust comparisons to determine the best model for the given dataset. The model selected as the best combination of an encoder and decoder, U-Net with a VGG16 backbone, demonstrated an accuracy and dice coefficient of 99.50% and 98.35%, respectively, on an unseen test dataset.
This study contributes to the initial step of iris identification to improve animal tracking systems; it suggests a framework for training DNNs for pixel-wise segmentation using a minimum use of annotation labels. For the reliable comparison of various combinations of DNN models to select the most suitable DNN model combination, this approach uses multiple metrics commonly used in the evaluation of segmentation, including visual references; hence, it is unbiased and has consistent model selection. The framework has the potential to improve the accessibility of DNNs for operators with limited knowledge of DNNs, accelerate inter-study comparisons, and reduce the variations in current manual model selection methods. Following this study, the authors plan to improve the framework’s model selection, image segmentation, machine learning, animal biometrics, and multi-resolution imaging. The goal of future research is to develop techniques and skills that can be applied to animal tracking, image recognition, and artificial intelligence applications in domestic animal field.