INTRODUCTION
Eggs are fragile and impossible to clean inside and external and internal defects readily occur [1]. Defective eggs are typically classified as bloody eggs, cracked eggs, and eggs with dirty shells. Dirt on the eggshell can be removed by washing. However, cracked eggs that cannot be restored or foreign objects inside eggs that cannot be washed should be discarded immediately. A defective egg can pose a risk of cross-contamination during storage [2]. In the past, eggs were inspected by skilled workers to remove defective eggs in advance [3]. This inefficient and subjective method can result in distribution of abnormal eggs to consumers. Conventional egg visual inspection methods are not suitable for detecting abnormal eggs and cracks. Eggs are one of the most widely consumed agricultural products in the world [4]. Emerging technologies to replace conventional egg inspection methods are needed to ensure safe eggs are distributed to consumers.
New abnormal egg detection technologies have been developed to replace the conventional visual inspection method [5]. Advanced technologies can detect abnormal eggs rapidly and accurately. Egg detectors have been systematized into egg sorting systems and are essential in the global egg industry. The global egg sorting system market is dominated by Moba (Netherlands), Nabel (Japan), and Kyowa (Japan). The sorting systems can be equipped with crack, dirt, and abnormal egg detectors. All crack detectors are acoustically analyzed. Abnormal eggs are detected using spectroscopy, and dirt is detected using a camera [6–8]. Abnormal egg detection systems developed by each company have excellent performance and are being used all over the world. The detection technologies and processing speeds of each manufacturer’s egg detector system are summarized in Table 1.
Studies to develop abnormal egg detection technology using a modified pressure technology [9–12] and hyperspectral imaging technology [13–16] have also been conducted in addition to the currently commercialized technology. All techniques for detecting defective eggs are non-destructive and require a defective egg identification algorithm. Non-destructive testing methods are important because if the shell is destroyed to inspect the inside of an egg, the egg is no longer valuable [17]. In addition, the accuracy of the algorithm along with the performance of the technology can improve the detection accuracy of abnormal eggs [18]. Among the numerous statistical-based data analysis models and machine learning models, it is important to use the right model. Currently, studies on performance verification of various learning models are being conducted to develop an optimized egg detection algorithm [15,19,20].
This paper was aimed to provide basic concepts of the currently developed inspection technologies for internal and external defects of eggs and to investigate the performance of the developed models to identify eggs in each technique.
DETECTION TECHNOLOGIES FOR EXTERNAL DEFECTS OF EGGS
Machine vision systems (MVSs), advanced technology that integrates mechanical, electronic, optical, and software systems, have been widely employed in a variety of commercial industries to identify defects of commercial products, as well as to monitor and control continuous processing [5,21]. Currently, MVSs have been introduced to various processes for agricultural products such as grading and sorting of fresh produces, crop monitoring, and internal quality (e.g. soluble solid content, moisture content, internal defects) and safety inspection [22]. In addition, the most extensively used method for inspecting external defects of eggs such as dirt and cracks on eggshells is a MVS system that integrates a lighting system and software algorithms as an alternative to human inspection [1,23]. Since light can penetrate the eggshell and the transmitted light can highlight the singularities in the eggshell, the images of the singularities can be acquired by a MVS [24]. The cracked egg detection system using MVS consists of roller conveyor for egg transportation, industrial camera, light box and egg candle (light source) as indicated in Fig. 1 of the research done by Guanjun et al. [24]. The roller conveyor was employed to transfer and rotate eggs so that the images of entire surface of eggs could be acquired by the camera. The egg candle was used to irradiate light to the eggs, and the light box could prevent occurring light noise from the experimental environment. The defects on the eggshell finally could be determined through further imaging processing. Egg candling and lighting boxes were effective for the image processing to detect external defects of eggs by removing the non-egg background from the image. A MVS for detecting external defects of eggs can provide faster and more objective inspection of important characteristics of eggs with high accuracy [25]. The effectiveness of MVSs for inspecting external defects of eggs has been evaluated by a number of researchers [4].
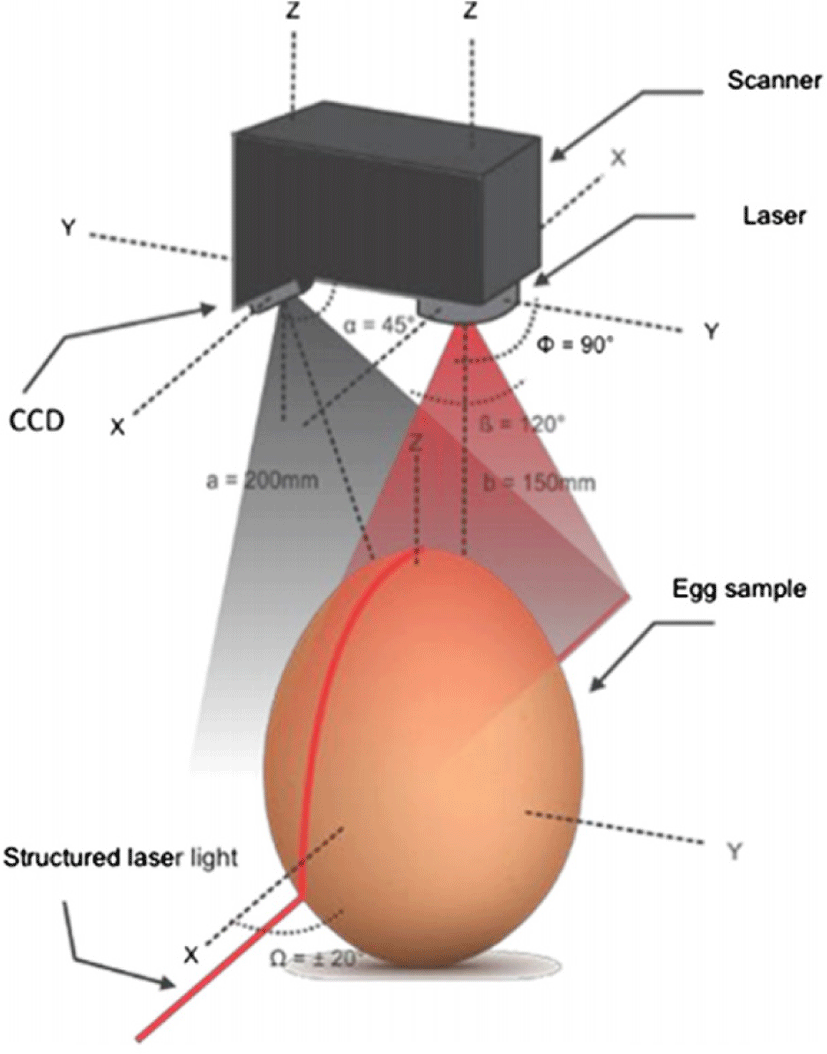
The eggshell dirt was detected by processing the acquired image from the eggs using a MVS including a charge-coupled device (CCD) camera under different illumination conditions [26]. A wooden cubic box was fabricated to remove light noise. In order to obtain clear images of eggs, halogen lamps were installed on the inside top of the box and yellow and white fluorescent tubular lamps were installed on the interior sides of the box. A total of eight lamps were installed inside the box. The image processing algorithm was developed using MATLAB 7.6 software. The canny edge detection method, one of the edge detection methods, was employed to detect the edges of the acquired egg images, and additional image processing functions such as the bwlabel function were applied to detect dirt on eggshells. The eggshell dirt was measured by counting the detected dirty pixels. The accuracy of the eggshell dirt algorithms was determined by comparison with a skillful egg inspector. The accuracy was 85.66% over all egg samples.
An egg grading system combined with machine vision and artificial intelligence technologies was designed and developed by Omid et al. [4]. Similar to the research done by Dehrouyeh et al. [26], a cubic box was fabricated to create uniform illuminance. A CCD camera was installed at the top of the box, and a halogen lamp was installed at the bottom of the box. An image processing algorithm including background removal, resizing, egg pixel counting, and crack pixel identification functions was developed to detect cracks in eggshells. In addition, a fuzzy inference system (FIS) for grading eggs was designed based on triangular, S-, and Z-shaped fuzzifiers, the Mamdani inference engine, and a defuzzifier (center of average defuzzification). The input variables for the FIS were cracks, blood, breakage, and size. The fuzzy interval determination and algorithm evaluation were conducted with human experts’ judgment. The algorithms for detecting size, cracks, and breakage of eggs showed an accuracy range from 93% to 98%, and a sensitivity range from 90.65% to 96.96%, respectively. The detection error from the algorithm for detecting sizes of eggs was caused by light noise and the adjacency of classification intervals. The overall accuracy of the egg grading fuzzy algorithm was 95.4%.
A negative Laplacian of Gaussian (LoG) operator was applied to a MVS to effectively detect invisible micro-cracks on eggs [24]. The detection of cracks on eggs using the MVS was complicated because of the noise factors (such as image noise and the area of freckles in eggshells). Image processing was performed to identify various cracks on eggs. The LoG operator used a Gaussian filter convolution, which can smooth the acquired images of eggs and remove small noises. The cracks in the acquired egg image were accentuated using the negative LoG operator. The sharpness of cracks and the backgrounds of egg images was changed depending on the scale of σ. When σ was 0.3, it was the best scale to indicate micro-cracks in the egg image. A hysteresis thresholding method was employed to obtain the binary egg image. In addition, the non-crack region in the binary egg image was eliminated using an improved local fitting image (LFI) index to dark spots. As a result, the accuracy of the developed algorithm for discriminating between cracked eggs and intact eggs was 91.3% as compared with human inspection. However, the accuracy was obtained only from egg samples without invisible micro-cracks because invisible micro-cracks could not be detected by human inspection.
A convolutional neural network (CNN) and artificial neural network (ANN), which can represent complex and complicated relations between input and output data, have been combined with MVS to rapidly detect external defects of eggs with superior accuracy [1,27,28].
An automatic egg sorting system using a CNN was proposed to detect internal and external defects of eggs [1]. The CNN model consisted of the modified VGG 16 structure (pre-trained CNNs) including the global average pooling layer to minimize overfitting with a reduction of the number of parameters, the dense layer with softmax classifier, batch normalization to retain all input variables of the layer, and the dropout layer for preventing overfitting. The rectified linear unit (ReLU) function was utilized as the activation function of the convolutional layers. The developed CNN model was trained, validated, and tested by using the acquired images from eggs with external and internal defects. The efficiency of the CNN model was estimated using a 5-fold cross-validation method. In this system, blood spots in eggs were detected as well as cracked eggs. The developed model was able to accurately detect all broken eggs and the blood spots in the eggs; however, there was a significant difference in appearance between broken and intact eggs. With this significant difference, it was possible to accurately detect broken eggs and blood spots in the eggs.
Turkoglu [27] developed a defective white egg detection system using a real-time MVS without a light source. The combined CNN and Bidirectional Long-Short-Term-Memory (BiLSTM) model was coupled with the detection system to identify dirt, cracks, and blood in eggshells. The developed model could segment each egg image from the acquired image containing 10 eggs and extract 1,000 features from the fc 1,000 layer for the segmented egg image using a pretrained DenNet201 model. The extracted features were fed into the component layers of the model (BiLSTM, ReLU, dropout, fully-connected, Softmax, and classification layer in order) to classify the labels (dirt, cracks, and blood) of input egg images. The developed defective egg detection model showed excellent performance with an accuracy of 99.17%.
A MVS combined with a CNN model to detect cracked eggs was also developed by Botta et al. [28]. The background and the edges of eggs from acquired egg images were removed through image processing. In addition, cracks and non-cracks on the egg images were separated and extracted using a filtering process. The developed CNN model based on the LeNEt-5 architecture included the ReLU function as the activation function, and the Softmax function was applied in the last layer of the model. Instead of examining cracks in the entire image, the image patches detected as features of cracks were extracted and utilized in the CNN model. After training, validating, and testing micro-crack and non-crack image patches, an accuracy of 95.38% was achieved in the developed model. The use of image patches was efficacious to improve memory efficiency and detection accuracy.
A line laser diode Adafruit with a center wavelength of 650 nm and an ANN were employed in a MVS to detect defects in eggshells [29]. The line laser diode used in this system projected structured light onto the surface of the egg, and an industrial RGB camera was used to acquire the image of the projected egg as shown in Fig. 1 in the paper [29]. The original image of the egg was used in conventional MVS, but the scanned laser light image of the egg surface was used in the system with a line laser camera. The diode with a wide numerical aperture of 120 ° could project light onto the large surface of the egg. The curvature of structured laser light on the egg surface was different depending on the presence of external defects in eggs. The acquired images from laser light scanning of eggshells were analyzed with the Levenberg-Marquardt ANN backpropagation algorithm. A well-smoothed curve was presented in the acquired line scanning image from the eggshells without defects. On the other hand, the scanned images obtained from eggshells with defects showed morphologically non-uniform curves. Detection accuracy of 97.5% and mean square error (MSE) of 2.6 × 10−6 were achieved in thisMVS.
The acoustic response system (or acoustic signal analysis) has been extensively studied to detect cracks in eggshells [30]. This method measures and analyzes the acoustic response or vibration signal generated by slight mechanical impact at several locations on eggshells, which can distinguish between cracked and normal eggs [31]. As shown in Fig. 1 of the research done by Wang et al. [32], the acoustic response system mainly consists of mechanical impact balls or rods, microphones for collecting impact sound, and a digital signal processing (DSP) unit. The excitation ball attracted to the annular magnet can vibrate up and down due to the attraction force of the magnet. The vibrating ball hits the egg several times, and the acoustic signal generated by repeated slight impacts can be collected using a microphone. The acoustic signal is analyzed by three important signal process steps (1. Extraction and conversion of acoustic features, 2. Selection of the most relevant feature from acoustic features, 3. Recognition of selected feature) to discriminate cracked eggs [32]. Although the acoustic response system cannot accurately detect cracked eggs, this system is widely applied in the poultry industry because it requires less data to be processed compared to the MVS [33]. The performance of the online acoustic response system for the detection of cracked eggs can vary depending on the acoustic signal analysis algorithms [34].
Sun et al. [35] evaluated the performance of an online acoustic response system for detecting cracked eggs. The analog acoustic signal generated from impacting eggs with a light metallic rod was collected by a microphone and an electric circuit was used to amplify and filter the collected signal. DSP was then employed to convert the signal into a digital signal. The converted signal was processed using Code Composer Studio (CCS) installed on the computer. The frequency range of the signal was from 1,200 to 6,800 Hz. The performance of the online system was enhanced by adjusting operation parameters such as the impact point and strength, the speed of the conveyor, and the position between impact point and crack direction. The optimal signal was obtained when the equatorial part of the egg was impacted by the rod. In addition, the speed of the conveyor and impact strength for achieving optimal signal without damage were 2.09 N and 28 cm/s, respectively. When the crack direction was perpendicular to the equator of the eggs, it was difficult to detect a cracked egg. A significant signal difference between normal and cracked eggs was observed at 2,900 Hz. The developed online system could indicate the different frequency signals of normal and cracked eggs by analyzing the frequency signal range collected through continuous impaction (over 40 times). As a result, the identification rate of normal and cracked eggs was 100% and 96.11%, respectively.
Kertesz et al. [33] artificially created a thin micro-crack on the pointy tips of eggs and measured the acoustic signal generated by impacting in the vertical and horizontal directions along the equatorial part of the egg. The acoustic signal was processed by a fast Fourier transform (FFT) and principal components analysis (PCA). Regardless of the impact direction, the detectable frequency signal range was below 3,000 Hz. The signal patterns obtained from normal and cracked eggs were significantly different in the frequency range between around 500 and 3,000 Hz. The accuracy of discrimination between normal and cracked eggs was 97.9% and a false positive rate of 0.87% was achieved. However, this system was not fully developed for application to the poultry industry.
Another approach to detect cracks in eggshells is a MVS coupled with a modified pressure method. Large cracks in eggshells can be easily detected by human inspection, candling, and MVS; however, micro-cracks, also known as hairline cracks, cannot be observed by the aforementioned methods. When the air pressure in the chamber containing eggs is suddenly dropped, the micro-cracks in the eggshells are instantaneously expanded due to the pressure difference between the air pressure in the chamber and the internal pressure of the eggs [9]. The instantaneously expanded micro-cracks in the egg can then be captured using a MVS. Even though this method requires supplementary devices (i.e. a vacuum pump and a well-sealed chamber equipped with a light source) and a long processing time, the detection accuracy is much higher than other detection methods, and can replace human inspection in egg processing facilities [10].
Lawrence et al. [9] developed a modified pressure imaging system to detect micro-cracks in eggshells. The system comprised a transparent vacuum chamber with a light source, a monochrome camera to capture cracks in eggs, and a vacuum pump. The egg images were acquired at atmospheric pressure and modified pressure (254 mmHg). Intact eggshells could withstand repeated vacuum pressure of 254 mmHg and micro-cracks in eggshells stably expanded below a vacuum pressure of 137.16 mmHg. Transmitted light through the egg could improve the performance of the MVS to detect micro-cracks. The acquired images of eggs at atmospheric pressure and modified pressure were compared to detect micro-cracks by using an image processing algorithm. The developed system achieved a detection accuracy of 98.8% in identifying all cracked eggs except for the eggs with cracks at the pointy tips.
As a continuation of the research done by Jones et al. [10], Yoon et al. [36] improved a modified pressure imaging system capable of examining 20 eggs. The system consisted of custom made vacuum chamber and pump, automatic rotating device, LED light source, and monochrome camera (Fig. 1 in the paper [36]). The custom made vacuum pump was able to instantaneously decrease the pressure inside the chamber, finally creating the pressure difference between the internal pressure of egg and the pressure inside chamber. A thick and transparent cubic chamber was designed and fabricated to prevent the deflection of the lid of the chamber and image distortion when a sudden vacuum condition was generated. A rotating device was installed in the chamber to acquire entire surface images of eggs by rotating the eggs about 90° to 120°. LEDs were installed under the rotating device. The egg images were acquired at two different pressure conditions (atmospheric pressure and modified pressure) in the chamber. The camera could capture the expanded egg surface in the short moment of pressure difference. The pressure inside the chamber then was returned to atmospheric pressure as the intake air by the vacuum pump returned to the chamber. This system was automatically controlled by combining hardware and software architectures. After acquiring images of eggs at different pressure conditions, the acquired images were analyzed using an imaging processing algorithm including a background mask and median filter function as indicated in the Fig. 1 in the paper [36]. To determine the effect of modified pressure on the change in the eggshell quality, preliminary tests were performed using eggs with poor shell quality. When modified pressure was applied in the chamber, the cracking rate of eggs was 0.03% and the modified pressure had little effect on the quality of the eggshell. In this system, the detection accuracy for micro-cracks in eggs was 99.6% and the false rejection rate was 0.2%.
Similar to the research done by Yoon et al. [36], the effect of modified pressure on the detection of cracked eggs was evaluated by Priyadumkol et al. [12]. The system was operated at a negative pressure of 254 mmHg and the rotation degree of the eggs was 120°. Egg surface segmentation, eggshell crack segmentation, and crack and dust identification functions were fed into the developed image processing algorithm. The accuracy of the developed algorithm for the detection of cracked eggs was 94% and the false negative rate was 1.67%.
DETECTION TECHNOLOGIES FOR INTERNAL DEFECTS OF EGGS
Typical cameras such as CCD and monochrome cameras can only inspect external defects of eggs. However, inspection of internal defects of eggs by a MVS equipped with an intensive light source that can penetrate the eggs is technically feasible [1]. When the intensive light penetrates intact and internally defective eggs, the color of the intact egg images is usually yellow, while bloody spots in the defective egg images are displayed in red color [26]. The difference between colors in the acquired images can be determined using a simple image processing algorithm.
Arivazhagan et al. [3] developed a MVS to detect blood in eggs. Similar to earlier studies [37,38], a wooden cubic chamber was fabricated to block light noise from the environment, and a halogen lamp and digital camera were installed in the chamber. The egg images were acquired using light transmitted through the eggs. A software algorithm including color correction, image filtering, and bloodstain area extraction functions was developed to detect blood in the eggs. The bloodstain area in the egg was represented by pixels with black color, and the other area in the egg image was indicated as pixels with white color. Finally, the bloodstain area was estimated by the size of the black pixels. The simple MVS with a light source could be used to detect blood in eggs.
The freshness of eggs was evaluated using a MVS coupled with an ANN [39]. The eggs samples were stored for 30 days, and the quality indices of the egg such as the area of the air cell, area index, Haugh unit (HU), and the density and pH values for the yolk and albumen were measured at 25°C and relative humidity (RH) of 20%. The measurement interval was two days. After capturing the egg images and measuring the weight of the eggs, pH, density, and HU values of the eggs were determined using destructive measurements. The egg and background areas from the acquired image were separated using an image processing algorithm, and the feature space (egg weight and area index) was extracted. The features were fed into a training neural network consisting of five training algorithms such as Levenberg–Marquardt and then evaluated. During storage, the pH value of the egg significantly increased with a decrease in the air cell area and area index. The weight of the egg was quite stable. The area index was substantially related to the pH and HU of the albumin with correlation coefficients of 0.77 and 0.86, respectively. The ANN algorithm developed with the area index and weight of eggs as inputs could accurately predict the pH and HU of the albumin and showed detection accuracies of 86.6% and 93.3%, respectively.
Harnsoongneon and Jaroensuk [38] proposed a simple egg grade and freshness assessment method using machine vision and a load cell sensor. The weight of the egg was measured using the load cell, and the volume of the egg was calculated from the length and width determined by the edge detection of the acquired images. The weight, length, width, and volume of the eggs were measured over a period of 30 days. The density of the eggs was calculated on the basis of the weight and volume of the eggs, and the density of the eggs consistently decreased during storage. The decrease in the density of eggs due to the evaporation of moisture through the tremendous pores on the eggshell surface could be used as egg grading and freshness assessment factors.
A MVS combined with a dielectric measurement method and an ANN was designed and developed to predict egg quality [40]. The dielectric properties of the egg were measured using the parallel method in a frequency range between 40 kHz and 20 MHz. The related voltage was calculated based on the measured dielectric properties. Egg samples were stored at 20°C and RH of 35% for 24 days. HU, yolk index, and yolk/albumen ratio were used as quality indices of the eggs. The volume of the eggs was determined by the water displacement method, and the vertical and horizontal length values of the eggs were obtained by processing the acquired images. The dielectric properties and quality indices of the eggs were measured at 1, 4, 7, 11, 18, and 24 days of storage time. The ANN model was developed using a feedforward neural network, backpropagation, and Levenberg-Marquardt algorithms. The measured voltage and dimensional characteristics of the eggs were utilized as the input of the developed ANN model to predict egg quality indices. The properties and quality indices of eggs decreased with an increase in storage time. The developed ANN model was able to accurately predict quality indices with R2 and MSE values of 0.99 and 0.064, respectively.
Spectroscopy technology can measure the energy of transmitted wavelength by irradiating the subjective material with optic light [41]. Spectroscopy can be classified according to the wavelength region used: ultraviolet (UV, 100 to 400 nm), visible light (Vis, 400 to 780 nm), near-Infrared (NIR, 780 to 2,500 nm), and mid-Infrared (MIR, 2,500 to 4,000 nm). Spectroscopy can provide a novel alternative to conventional destructive methods to measure food quality because of its non-destructive and rapid manner [42]. Among different types of spectroscopy, NIR spectroscopy has been widely employed in the poultry industry to measure the quality of eggs [43,44]. In recent years, visible NIR (Vis-NIR) spectroscopy has also been utilized to detect the internal defects of eggs [45]. The system for detecting internal defects in eggs using spectroscopy is simply composed of a light source that illuminates the egg, a white chamber that blocks external light, and a spectroradiometer sensor to acquire only the transmitted light through egg sample as indicated in Fig. 1 of the research done by Abdel-Nour et al. [45]. To prevent the eggs from being heated by the light source, the light source should be turned on for a short time period required for spectral measurements. The Vis-NIR spectral data of the egg is obtained by a spectrodiometer that can measure transmittance wavelengths. However, spectral data are an overlapping signal, and it is complicated to identify important signal information. Therefore, in order to extract the practical data from spectral data, it is necessary to exploit optimal data processing based on a multivariate statistical analysis [46].
A freshness assessment of eggs was carried out using NIR spectroscopy and ANN combined with a multivariate statistical technique [47]. The reflectance mode of NIR spectroscopy was used to obtain NIR spectra of eggs, and the spectra were recorded using a NIR spectrophotometer connected with a fiber-optic probe. The spectra were measured at the equatorial part of the egg and the wavelength range of the spectra was between 1,000 cm−1 and 4,000 cm−1. Standard normal transformation was applied as a preprocessing method of the spectral data to correct the baseline offset of the acquired spectra owing to the scattering effects. Effective features were extracted from the original spectral data using two types of extracting methods (independent component analysis [ICA] and PCA) that could remove overlapping information and reduce the data size. An ANN combined with a genetic algorithm (GA) was developed to predict the freshness of the eggs. The eggs were stored at 25°C and RH of 70% for 2 weeks, and the NIR spectrum and HU of the eggs were measured daily. The extracted features from the preprocessed NIR data were used as the input of the GA-ANN model and then the HU of the eggs was predicted as the output of the model. More than half of the extracted spectra features and the measured HU data were used as a calibration set to develop the GA-ANN model and the remaining data were used as a validation set to evaluate the performance of the model in terms of the root mean square error of prediction (RMSEP) and correlation coefficient (R). The identification rates of eggs in the model were 92.4% and 91.4% in the calibration and validation sets, respectively. When the ICA method was applied to the GA-ANN model, better performance than the GA-ANN model combined with PCA was obtained.
A quantitative model based on Vis-NIR spectra of a single variety (reference variety) of eggs was applied to another variety (comparison variety) of eggs [17]. The model was developed by changing the order of reference variety. White Leghorn egg and Bantam eggs were used in this study and the two varieties of eggs were stored for three weeks at 30°C and RH of 60%. Albumen pH (freshness factor) and the spectral data of both egg varieties were measured every two days during the storage. The spectral data were acquired in a Vis-NIR wavelength range from 340 nm to 1,030 nm. The outliers of the spectrum were eliminated using the Mahalanobis distance combined with PCA. Savitzky-Golay (SG), multiplicative scattering correction (MSC), SNV, and 1st and 2nd SG derivatives were used for preprocessing spectral data prior to the development of the model. Partial least square regression (PLS-R), which can extract important information from the acquired spectral data, was employed to develop the model for predicting the pH of albumen. In addition, calibration updating and transfer methods such as global updating, direct standardization (DS), and slope/bias correction (SBC) algorithms were utilized to apply the developed model based on one variety of eggs to another variety of eggs. The pH values of both egg varieties increased during the storage time. The spectral data of both egg varieties were significantly different in the wavelength range between 600 nm and 650 nm. The developed model with the MSC and SBC methods for both egg varieties showed good performance to predict the pH of the albumin on the basis of processed spectral data in terms of the correlation coefficient of prediction (rp) and RMSEP. Furthermore, when the model was developed with the Bantam egg variety as the reference variety, better prediction results than the model with the White Leghorn egg variety were achieved due to the brown color, chemical properties, and complex structure of the Bantam egg.
Simple and low-cost portable spectrometers have been also employed to predict the storage time and freshness of eggs [19,48]. An egg storage time prediction model was developed using NIR spectral data of eggs obtained from a portable spectrometer [48]. The portable spectrometer connected to a smartphone could easily collect the spectral data of eggs in a wavelength range between 740 nm and 1,070 nm. The spectral data of eggs were measured every 24 h for 21 days at the blunt end of the eggs stored at about 23°C. The changes in spectral data were evident between 0–7 days and 14–21days. Six pre-processing techniques (SG, Beer-Lambert law, standard normal variate [SNV], Multiplicative scatter correction [MSC], and first and second spectral derivative [FSD, SSD]) and two calibration algorithms (partial least squares [PLS] and ANN) were deployed to develop the prediction model. The informative wavelength was selected for the prediction model using the best combination of preprocessing technique and calibration algorithm. R2 and root mean square error of cross-validation (RMSECV) values were used as evaluation indicators for the model. The 660 collected spectral raw data were divided into nine fold of calibration (70%)/ validation (20%), and one fold of test (10%) sets using 10-fold cross validation to evaluate the developed prediction model. The optimal combination of prediction model was SG and ANN, which obtained a R2 of above 0.8. The optimization-process for the parameters of the SG and ANN combination was performed using training and validation sets. The optimized model was examined using test sets, and the obtained R2 and RMSEC values were 0.873 and 1.97 days, respectively. Although the R2 value was lower than 0.9, the RMSECV value was in a reasonable range for predicting egg storage time.
A portable and low-cost NIR spectrometer was used to make real time prediction of egg freshness depending on the HU [19]. Two egg sample groups were stored for different storage periods (maximum 21 days) at 20°C and 30°C and the range of RH was between 50% and 65%. At the end of the storage period, the spectral data of eggs in the wavelength range between 900 nm and 1,700 nm were measured at the top (R3), middle (R2), and bottom (R1) parts of the eggs. After measuring the HU value of the eggs, the freshness grade of the eggs was calculated according to the HU value and the grade was divided into three classes (AA, A, and B). SNV was used to correct the light scattering effects of raw spectra of eggs, and the random noise and changes in the baseline of the spectra were corrected using the 1st and 2nd SG derivative preprocessing methods. PCA was performed using two spectral groups (full spectra and selected spectra) in order to analyze the differences in the spectral data depending on the freshness grades. PLS-R and support vector machine regression (SVM-R) methods were adopted to develop the HU prediction model using reflectance values of the NIR spectra as input variables. PLS discriminant analysis (PLS-DA) and SVM classification (SVM-C) were deployed to classify eggs depending upon the freshness. PLS-DA and SVM-C methods were tested using two classifications: (1) AA, A, and B grade eggs and (2) fresh (AA and A) and stale (B) eggs. Among the performance indicators of the regression model, the most important indicators affecting the accuracy of the prediction model were the relative error (RMSEP / average of the prediction data set) and the ratio of standard deviation (RPD, defined as the standard deviation of the prediction data set / RMSEP). The SVM-R model using the average spectral values measured at three positions (mean R1 + R2 + R3) was the most effective for predicting the HU of eggs. In this case, the wavelength range was between 1,300 nm and 1,690 nm, and the relative error and RPD were 7.32% and 2.56, respectively. The PLS-R model using average spectral values measured at R1 and R2 also provided good HU prediction. The accuracies of the PLS-DA model for classifying fresh and old eggs were 85% and 87%, respectively.
A simple Vis/NIR spectroscopy system can also be used to detect bloody defects inside eggs [20,49]. A Vis/NIR spectroscopic system consisting of a handheld spectrometer, optical fiber, and a halogen lamp was applied to detect blood spots inside brown eggs [20]. Bloody eggs were fabricated by injecting a certain amount of blood into an artificially created hole slightly below the center of the upper part. The injected blood volumes were 0.01, 0.04, 0.07, and 0.1 mL. The angle of two light sources for irradiating eggs was set to 30°, and the distance between the egg and the light source was 100 mm to prevent damage to the eggs by heat. The spectral data of the eggs were collected from 471 nm to 1,154 nm. Smoothing, MSC, SNV, and regularization methods were employed for preprocessing the spectral data, and the PLS-DA model was developed to detect bloody spots inside eggs. The performance of the model was expressed as R2 and the standard error of the prediction set (SEP). Although the optimal data preprocessing method differed depending on the blood volume in the eggs, the MSC model was the most suitable for detecting bloody spots inside eggs regardless of the blood volumes. The MSC model accuracy increased from 86.5% to 97.9% with an increase in blood volumes.
Hyperspectral imaging (HSI) technology integrates conventional imaging and spectroscopy techniques to simultaneously acquire spatial and spectral data for each pixel of a subject in multiple spectral bands [50]. A hyperspectral image consists of two spatial dimensions (X, Y) and one spectral dimension (λ). The shapes of spectral curves of the objects obtained from HSI can be significantly affected by the chemical composition or intrinsic physical properties of objects [51]. Therefore, the hyperspectral image of an object can provide chemical information that cannot be obtained with a typical MVS [52]. HSI is one of the emerging non-destructive and rapid technologies for the inspection of agricultural products [53]. Choosing an appropriate wavelength from the full spectra of an object can reduce the configuration and cost of the HSI system, finally increasing the efficiency of the system [54].
HSI techniques are useful to detect stale eggs by predicting the freshness of eggs [13–15]. Hyperspectral imaging was used to examine internal quality (freshness, air bubbles, and scattered yolks) of eggs [13]. Hyperspectral images of eggs were acquired using a pushbroom HSI system consisting of a CCD camera, an imaging spectrometer, and an illuminator. The acquired spectral image of an egg consisted of 440 images extracted in the spectral range from 380 nm to 1,010 nm. In order to extract spectral information (i.e. image characteristics, the selection of an effective wavelength, prediction, and classification), image calibration was carried out using percent transmission to the acquired spectral image. The region of interest (ROI) was selected from the calibrated image, and the successive projection algorithm (SPA) was deployed to extract the appropriate spectral features and wavelength for the egg freshness assessment. The mean ROI was utilized as an average transmission value. PCA was conducted to reduce the dimensionality of the calibrated image and to extract the optimal characteristic images. In addition, the gray level co-occurrence matrix (GLCM) was employed to extract the optimal texture variables from the characteristic pictures that were used to detect the internal bubbles. Imaging processing was developed to detect the scattered yolk in the eggs. The scattered yolk was determined according to changes in its morphological characteristics in the processed image. The calibration model was developed with PLS-R and SVM-R to evaluate egg freshness using a full spectral range. In addition, SVM-C was used to distinguish the eggs with internal bubbles and scattered yolks. The performance of the egg freshness assessment model was expressed as RPD, root mean square error of calibration (RMSEC), RMSEP, and the coefficient of determination of calibration (R2c) and prediction (R2p). The SVM-C model was evaluated by the total accuracy, defined as the ratio of correctly classified number of eggs over the total number of eggs within the calibration and prediction sets. The egg samples were divided into three sets to detect freshness, bubbles, and scattered yolks. Egg set 1 for freshness was stored at 20°C and RH of 50% for 0, 7, 14, 21, 28, 35, and 42 days and HU was measured after hyperspectral image acquisition. Egg sets 2 and 3 were artificially damaged by simulated transportation vibration. In all three sample sets, 60% and 40% of the eggs were used for calibration and prediction models, respectively. The R2p and RMSEP values obtained from the PLS-R prediction model were 0.78 and 5.30%, respectively. The values from the SVR model using PCA were 0.85 and 4.33%, respectively. This low accuracy was caused by the influence of the eggshell pores on the transmission spectral properties. The selected optimal spectral wavelengths for SPA were 620, 632, 654, 671, 680, 684, 697, 707, 712, 724, 762, 780, and 796 nm. The R2p, RMSEP, and RPD obtained from the selected optimal wavelengths based SPA-SVR model were 0.87, 4.01%, and 2.80, respectively. The freshness of eggs depending on the HU was predicted by applying the SPA-SVR model. The detection accuracies of the SVC model for internal bubbles and scattered yolks in eggs were 90.0% and 96.3%, respectively.
Egg freshness was evaluated using the NIR-HSI system with comparison to HU measurement [14]. The NIR-HSI system consisted of a CCD camera, an imaging spectrometer, and a tungsten halogen lamp. Eggs were stored at room temperature for 21 days, and the spectra data and HU of eggs were measured approximately every four days. Data preprocessing and the selection of the ROI of hyperspectral images of eggs were performed in a manner similar to the aforementioned research. The egg freshness assessment model was developed with PLS-R using the spectral data in the range between 900 nm and 1,700 nm. The ROI was cropped at the center of each hyperspectral egg image and the average spectra of the ROI were applied to model calibration. Different types of data preprocessing methods such as SG smoothing, SG derivative, MSC, and SNV were evaluated to determine the optimal conditions for the calibration model and tested through cross-validation. The SNV preprocessing method was able to achieve the best results for the prediction of egg freshness.
The optimal conditions of the egg freshness assessment model were investigated by using different types of hyperspectral images of eggs [15]. The reflection, scattering, transmission, and mixed hyperspectral images of eggs were acquired using a dual hyperspectral camera, and the incident angle of the light source to irradiate eggs was increased from 0° (for scattering hyperspectral image) to 60° (for mixed hyperspectral image) with an increment of 10°. The egg freshness assessment model was developed by applying a variety of data preprocessing methods, data extracting methods, and classification models, and the optimal combination of conditions for the model was investigated. Brown eggs were used in the experiment, and hyperspectral images and HU data of the eggs were collected weekly for four weeks. The developed model with a MSC-SPA-DAC combination method and scattering hyperspectral image showed the highest accuracy of 96.25% for egg freshness assessment. The incident angle of the light source had a significant impact on the accuracy of the model. Among four types of hyperspectral images, the scattering hyperspectral image was the most suitable to accurately predict the freshness of eggs.
In addition, bloody eggs were detected using the HSI system [16]. Hyperspectral images of intact brown eggs and dried brown eggshell fragments were acquired from a spectrum range between 450 nm and 1,000 nm. The entire area of the egg was used as the ROI from a hyperspectral image of an intact egg, while the ROI of shell fragments was extracted manually. Raw spectral data were corrected and normalized to minimize the influence of transmitted light intensity caused by eggshell thickness. k-nearest neighbor (KNN) and SVM models were compared to develop the bloody egg detection model. The KKN model achieved less than 90% accuracy in all variables, but SVM achieved better accuracy than KNN in all variables, and input variable 3 achieved 100% accuracy.
CONCLUSION
The present review demonstrated the application of a variety of technologies for detecting internal and external defects of eggs. Egg quality inspection has been conducted using simple image imaging techniques and spectroscopic analysis methods analyzing complex spectral data. All detection technologies for defects of eggs required a discrimination software algorithm. CNN models, which contributed greatly to the success of object recognition, have been widely applied to various studies on detecting the exterior of eggs using MVSs. In addition, the SVM model, one of the machine learning models used for pattern recognition and data classification, has been applied to the internal inspection of eggs. As a result, the majority of the detection techniques for defects of eggs achieved greater than 95% accuracy. The currently developed detection technologies for defects of eggs are summarized in Table 2. However, most of the studies were conducted on a laboratory scale, and the processing speed required from the commercial poultry industry was not achieved. Therefore, it is necessary to measure the number of inspections per unit time as a performance indicator of the technology in future studies.
Detection method | Target (Measurement index) | Sample egg | Prediction algorithm | Accuracy | References |
---|---|---|---|---|---|
Machine vision | Dirty egg | 350 white eggs | Image processing algorithm developed in the study | 85.7% | [26] |
Cracked egg | 400 white eggs | FLI model | 94.5% | [4] | |
Cracked egg | 150 eggs | Negative LoG -LFI model | 91.3% | [24] | |
Broken egg | 67 white eggs | Image pre-processing and CNN model | 100.0% | [1] | |
Bloody egg / cracked egg / dirty egg | 400 white eggs | SMI-CNN-BiLSTM model | 99.2% | [27] | |
Cracked egg | 130 white eggs | Image pre-processing and CNN model | 95.4% | [28] | |
Bloody egg | 200 white eggs | Image processing algorithm developed in the study | - | [3] | |
Stale egg (HU and albumen pH) | 210 eggs | Image processing - LM algorithm | 93.3% | [39] | |
Machine vision + line laser | Cracked egg | 200 brown eggs | Image pre-processing and ANN model | 97.5% | [29] |
Machine vision + density measurement | Stale egg (storage time) | 87 brown eggs | Calibration model developed in the study | 99% or more | [38] |
Machine vision + dielectric measurement | Stale egg (HU) | 287 white eggs | ANN model | 99% or more | [40] |
Acoustic response | Cracked egg | 203 brown eggs | FFT-DSP-calibration model developed in the study | 98.0% | [35] |
Cracked egg | 693 brown eggs | PCA-FFT-QDA model | 99.6% | [33] | |
Negative pressure | Cracked egg | 160 white eggs | Image processing algorithm developed in the study | 98.7% | [9] |
Cracked egg | 201 white eggs | Image processing algorithm developed in the study | 94.5% | [36] | |
Vis-NIR spectroscopy | Stale egg (albumen pH) | 96 white eggs and 96 brown eggs | MSC-SBC model (brown egg as a reference variety) | 90.8% | [17] |
Bloody egg | 200 brown eggs | MSC-PLSDA model | 97.9% (0.1 mL) | [20] | |
Bloody egg | 194 brown eggs | MSC-1st derivative-BLR model | 96.9% | [49] | |
NIR spectroscopy | Stale egg (HU) | 185 white eggs | SNV-1st SG derivative-iPLS-SVMR model | 88.0% | [19] |
Stale egg (freshness grade) | 185 white eggs | SNV-1st SG derivative-PLSDA model | 87.0% | ||
Stale egg | 176 eggs | ICA-GA-ANN model | 91.4% | [47] | |
Stale egg (storage time) | 66 brown eggs | SG-ANN model | 87.3% | [48] | |
Hyper-spectral imaging | Stale egg (HU) | 100 white eggs | SPA-SVMR model | 84.0% | [13] |
Bubble in egg | 80 white eggs | PCA-GLCM-SVMC model | 90% (90°) | ||
Scattered yolk in egg | 80 white eggs | image-processing algorithm-SVMC model | 96.3% | ||
Stale egg (HU) | 33 white eggs | SNV-PLSR | 85.0% | [14] | |
Stale egg (HU) | 150 brown eggs | 0° scattering MSC-SPA model | 100.0% | [15] | |
Bloody egg | 34 brown eggs | Normalization-SPA-SVM model | 96.4% (Input 4) | [16] |
FLI, fuzzy logic inference; Log, laplacian of gaussian; LFI, local fitting image; CNN, convolutional neural network; SMI, sequential multiple image; BiLSTM, bidirectional long-short-term-memory; HU, haugh unit; LM, levenberg-marquardt; ANN, artificial neural network; FFT, fast Fourier transform; DSP, digital signal processing; PCA, principal component analysis; QDA, quadratic discriminant analysis; MSC, multiplicative scatter correction; SBC, slope/bias correction; PLSDA, partial least square discriminant analysis; BLR, binary logistic regression; SNV, standard normal variate; SG, Savitzky Golay; iPLS, interval partial least square; SVMR, support vector machine regression; ICA, independent component analysis; GA, genetic algorithm; SPA, successive projection algorithm; GLCM, gray level co-occurrence matrix; SVMC, support vector machine classification; PLSR, partial least square regression; SVMC, support vector.