INTRODUCTION
The commercial value of dairy products strictly depends on milk quality. Milk adulteration is a frequent problem in many countries that, in case of allergy, can even cause life-threatening consequences for the consumers. The most frequent type of adulteration is represented by a partial or complete replacement of more valuable products with cheaper ones. Milk fraudulent adulteration is always pushed by an easy additional economic income and, sometimes, could be used to adjust milk defects such as a high bacterial load/somatic cell count or the presence of contaminants/pollutants. In the case of buffalo milk, this is often adulterated because of its high cost and low availability in certain periods of the year. This is due to the reproductive seasonality of the buffalo species.
Italy contributes for over 90% of the European production [1] and, over 95% of the total amount of the European buffalos’ milk is used for dairy products [1]. Most of the buffaloes are reared under intensive conditions for milk production that is almost entirely transformed in mozzarella cheese that obtained the European “Protected Designation of Origin” (PDO) in 1996 [2]. The steadily growing demand and the high market price of this product, combined with the low availability of raw milk for processing, made buffalo milk an exploitable target for adulteration [3].
For this reason, it is necessary to develop a rapid and reliable method to verify the quality and authenticity of milk and dairy products to protect both the intrinsic value of the product and the consumers from fraud. Furthermore, as previously mentioned, some cow milk proteins can induce allergies and other adverse effects and pose a high risk to susceptible consumers if their presence is not declared in food [4]. The official method to detect the presence of cow milk in ewe, goat, and buffalo milk is the isoelectric focusing of γ-caseins after plasminolysis [5] which is only a qualitative and time-consuming method that requires experienced and qualified personnel. Many analytical methods, such as capillary electrophoresis [6], synchronized fluorescence spectroscopy [7], mass spectrometry-based procedures [8,9], or other spectroscopy-based techniques [10] have been proposed over the years to improve speed and reliability. Among them, Fourier Transform (FT)- mid-infrared (MIR) is already used routinely to quickly determine the composition of milk due to its fast, easy use, and simple sample preparation [10]. Furthermore, has been recently demonstrated that this technique could be successfully applied to detect a range of potential adulterants in milk [11].
Some studies have already demonstrated the applicability of spectroscopy to detect or quantify milk from species other than those declared [10–15]. In the present study, we applied a new FT-MIR spectroscopy method combined with chemometrics analysis, such as the partial least square (PLS) regression, for the quantitative detection of cow’s milk as an adulterant in buffalo milk.
MATERIALS AND METHODS
A total of 14 raw bulk tank milk samples of 2 L each were collected: 7 milk samples from dairy cow (Bos taurus) herds of different breeds (Jersey, Holstein-Friesian, Italian Brown, Italian Simmental, Angler, and crossbreed) and 7 milk samples from different Mediterranean buffalo (Bubalus bubalis) herds. All farms were located in Calabria Region (Southern Italy) and all milk samples were collected from October 2017 to February 2020. After collection, the samples were kept refrigerated at 4°C and immediately delivered to the laboratory of the University of Catanzaro for the analysis by MilkoScan FT+ (Foss, Hillerød, Denmark) instrument.
The milk mixtures were prepared by randomly pairing one of the 7 buffalo milk with one of the 7 cow milk and then blending them according to the following 17 proportions: 0%, 1%, 2.5%, 5%, 10%, 20%, 25%, 30%, 50%, 70%, 75%, 80%, 90%, 95%, 97.5%, 99%, and 100% (vol/vol).
A total of 119 standards (17 mixtures × 7 different couples of buffalo-cow milk) were obtained. Before the analysis, standards (45 mL each) were warmed in a 40°C water bath for 20 minutes, slightly stirred for a few seconds and then analyzed by MilkoScan FT+ for the MIR spectra acquisition as the transmittance value at each of the 1060 points of wavelength from 5,011 to 925 cm−1. This instrument analyzes the chemical composition of milk according to the standards of the International Dairy Federation (FIL-IDF) by the FT technology over the Mid-infrared spectral range. Spectra were stored as absorbances (A) using the transformation A = Log (1/T), where T is the transmittance (Fig. 1). Two spectral acquisitions were carried out for each sample, and the results were averaged before data analysis.
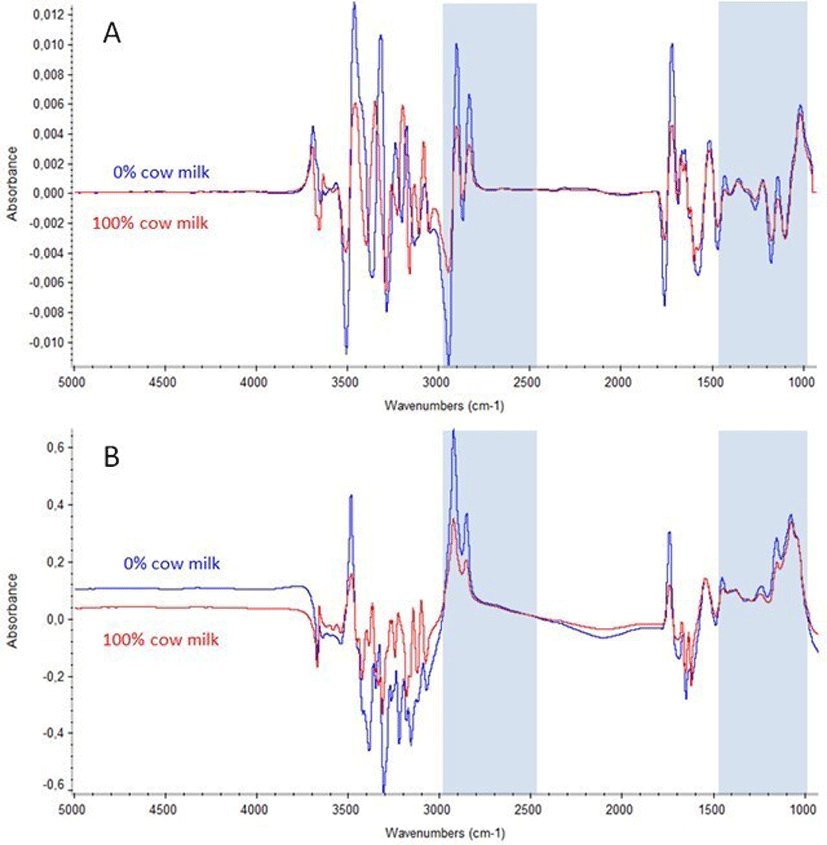
All spectral data were elaborated by PLS regression using the TQ AnalystTM software ver. 8.0 (Thermo Fisher Scientific, Madison, WI, USA) in order to quantify the % (vol/vol) of bovine milk in buffalo milk. 85 spectra belonging from 5 out of 7 mixtures pairs (randomly chosen) were used as calibration standards. The remaining 34 spectra were used as independent validation standards. In this way, spectra were distributed in two sets (70% for training and 30% for validation) evaluated by PLS in order to develop a multivariate calibration model. The number of latent variables in the model was tested according to the method proposed by the software. After leave-one-out cross validation, the number of the principal components (factors) that minimized the root mean square error of cross-validation (RMSECV) of the model was nine. After selecting the number of latent variables [16], the PLS model was used to recognize the concentration of cow’s milk in buffalo’s milk.
The accuracy and precision of the calibration model are expressed as correlation coefficient (R2) of the calculated vs. real values, as the root mean square error of calibration (RMSEC) and the root mean square error of prediction (RMSEP). The choice of the best model followed this rule: the higher R2 and the lower RMSEC and RMSEP, the better the models were considered [17]. Furthermore, a cross-validation using “leave-one-out” procedure was used to evaluate the overfitting of the calibration and the RMSECV was calculated.
As suggested by Muik et al. [18], to improve the performance of the prevision, the following corrections were applied on original spectra: first derivative; smoothing by Norris derivative filter with 5 both as segment length and as gap between segments. Data were normalized by using the mean centering technique.
RESULTS AND DISCUSSION
The qualitative composition of the bulk tank milk of the two different species was firstly observed in this study (Table 1). The buffalo milk average protein, fat, and lactose content were respectively 8.41 g/100 g, 4.64 g/100 g and 4.83 g/100 g. The same parameters for cow milk were 3.83 g/ 100 g, 3.50 g/100 g and 4.76 g/100 g. These data show the higher contents of protein and fat in buffalo milk than in cow’s milk and are in agreement with those reported in previous studies [6,19].
Milk components | Buffalo milk (N=7) | Cow milk (N=7) |
---|---|---|
Fat (g/100 g) | 8.41 ± 0.29 | 3.83 ± 0.31 |
Protein (g/100 g) | 4.64 ± 0.11 | 3.50 ± 0.29 |
Lactose (g/100 g) | 4.83 ± 0.16 | 4.76 ± 0.13 |
Two buffalo and cow milk representative MIR spectra, before and after spectral treatment, are reported in Fig. 1. The absorbance regions that best correlated with the percentage of cow’s milk in buffalo milk were suggested by the TQ analystTM and were those in the ranges 2,989.12–2,495.44 and 1,481.06–987.38 cm−1 (Fig. 1). As expected, the spectral regions corresponding to water interference [20] did not fall within the regions considered appropriate for a good calibration. More precisely, these spectral regions fall between 1,680 to 1,600 cm−1 and 3,650 to 3,000 cm−1 (O-H stretching) [20]. Among spectral ranges having no useful information there is also the area between 1,765 to 1,730 cm−1 associated with C=O stretching modes (triglyceride) and the area between 1,700 to 1,500 cm−1 containing the peak typical of amide I (C-O) and amide II (N–H) [21]. Among other biomolecules populating the spectrum can be annotated lipids, whose absorption region is between 1,477 to 1,400 cm−1 (C-H stretching) and caseins (phosphate group) that are absorbing at 1,100 cm−1 [22].
The peaks at 1,200–900 and 1,045 cm−1 are associated with lactose, and those at 3,000–2,800 cm−1 and 1,400–800 cm−1 are related to carbohydrates, monosaccharides and polysaccharide groups (C-O-C) [15]. All the spectra were pre-processed for the outliers detection [23] through the Chauvenet test and no outliers were found among the 119 spectra [23].
The performance of PLS calibration models for both original and pre-treated spectra is shown in Table 2. Both calibrations showed good results, but the spectral pre-processing allowed to significantly improve the quality of the prediction as indicated by the lower RMSEP values. Generally, a good model should have lower RMSECV, RMSEP and higher R2 but small differences between RMSEP and RMSECV [8].
Spectral treatment | R2 cal | RMSEC | R2 val | RMSEP | RMSECV | N° of factors |
---|---|---|---|---|---|---|
Normal | 0.99857 | 2.08 | 0.99770 | 6.06 | 2.63 | 10 |
First derivative, Norris filter | 0.99861 | 2.04 | 0.99803 | 2.84 | 2.44 | 9 |
Both the high value of the correlation coefficient and the low RMSEP value indicated the success of the PLS regression on the pre-processed spectra and, for these reasons this calibration model may be preferred to the one elaborated on the raw spectra. Fig. 2 shows calibration results for the detection of bovine milk percentage in buffalo milk using the calibration model on the pre-treated spectra. It can be observed that data points in the plot of the calculated vs. actual values (Fig. 2A) drew a line with a 45 degrees angle with respect to both axes. No bias, intended as a systematic difference between expected and true values, was detected. For this reason, the data points in the % difference plot (Fig. 2B) were distributed randomly above and below the zero line within a concentration ranging from −7% to +7% of the actual value. The differences between calculated and actual values of the validation standards were similar to those observed for the calibration standards, this feature indicates that there was no overfitting for the considered calibration.
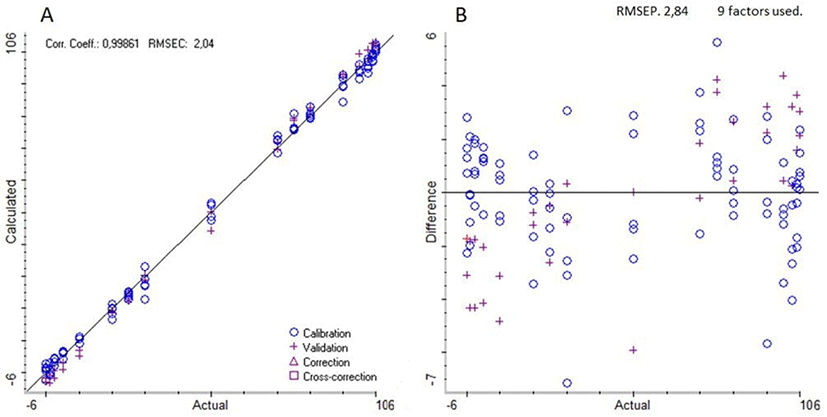
In a similar work detecting the adulteration of cow, sheep and goat milk by Fourier Transform Infrared spectroscopy (FTIR) in combination with multivariate statistical methods, Nicolaou et al. [24] obtained calibration models characterized by prediction error higher than those observed in the present study and ranging from 3.95% to 8.03%. However, recent research that used FTIR method with multivariate statistical analysis was successful in the differentiation between buffalo milk and buffalo milk added with cow milk [15].
In our research, the applied PLS regression models were able to detect as little as 3% cow in buffalo milk, reaching a good accuracy (Table 2). Although the adulteration of buffalo milk with cow’s milk is unlikely to occurs at concentrations below 3% due to limited economic convenience, from a scientific and technical points of view is relevant to have such a sensitive and cheap method for the detection of this type of fraud.
This is of major importance, especially considering that the presence of small quantities of cow’s milk in buffalo milk may be life threatening in the case of consumers with allergies to cow milk proteins. Cow’s milk has about 30 potentially allergenic proteins and, the foods that contain it must necessarily declare it on the label [25]. Other recent studies applied spectroscopy associated with multivariate statistics to verify the authenticity of buffalo milk. Silva et al. [26], analysed lyophilized milk samples with attenuated reflectance (ATR)-FTIR in association with PCA and artificial neural network. The authors could detect differences between different milk species only accounting for 40% of adulteration and could not assess the content of cow’s milk added to buffalo milk, but only its presence. Also, Gonçalves et al. [16] developed a similar method for the quantification of buffalo’s milk adulterants (e.g. cow’s milk) using MIR spectroscopy coupled to PLS and multiple linear regression (MLR). The obtained results were similar to the ones here presented, with R2 of 0.9938 and RMSEP of 3.484% for the adulteration. Durakli Velioglu et al. [27], similarly to Gonçalves et al. [16], when researching the authenticity of buffalo’s milk by fluorescence spectroscopy associated with principal component analysis (PCA) and PLS, found models with an R2 of 0.98. Instead, RMSEC, RMSECV, and RMSEP values were determined as 2%, 7% and 4%, respectively, indicating slightly higher prediction errors than the values in our findings.
In the study of Lapcharoensuk et al. [28], a near-infrared (NIR) spectroscopy model was used to quantitatively detect buffalo milk adulteration with cow milk, but on pasteurized milk samples. The authors yielded a R2 of 0.998 and RMSEP of 2.121. Although the RMSEP obtained in all these works are very similar to that obtained in our study, most of the above-mentioned authors only used adulterations ranging from 10%–90% (v/v) while we used adulterations ranging from 1%–99% (v/v). Moreover, we enrolled cow milk samples coming from 7 different breeds while the other studies used only 2 different breeds.
CONCLUSION
In this study, we demonstrated that MIR Spectroscopy in combination with PLS regression analysis provides an accurate, simple and rapid method for quantitative assessment of the adulteration of buffalo milk with cow’s milk. For this reason, we believe that the proposed calibration can be a useful tool for expanding the possibilities of combating fraud in laboratories equipped for routine milk analysis using dedicated FTIR instruments at no additional cost.