INTRODUCTION
Pig breeding for economic traits has undergone continuous improvement over time, with ongoing research in this field. Productive traits such as average daily gain (ADG), days to 105 kg (AGE), and backfat thickness (BF) have moderate to high heritability. ADG and AGE directly influence pig growth [1, 2]. BF is a trait linked to reproductive performance of Landrace and Yorkshire sows [3], making it crucial for enhancing and maintaining mothering ability of the dam.
According to the Korean Swine Performance Recording Standards (KSPRS) established by the Ministry of Agriculture, Food and Rural Affairs (MAFRA), performance testing is conducted within a weight range of 70–110 kg, with the endpoint set at 90 kg. Days to reach 90 kg and BF are adjusted to assess growth trait performance. However, the endpoint weight of 90 kg has remained unchanged since its establishment in 1984, reflecting the market weight of finishing pigs at that time. With current trend of market weights surpassing 110 kg, there is a growing consensus that the endpoint weight for performance testing should be increased. Consequently, there is a need to develop a new adjustment formula for performance testing, resulting in the creation of a 105 kg-based adjustment formula by the National Institute of Animal Science (NIAS).
Genome-wide association studies (GWAS) have been widely applied in various fields, including the identification of economic traits. Multiple candidate genes and significant markers have been reported for the same trait, with associations between multiple traits observed at the same locus. These results are inherent to quantitative traits, single-marker GWAS analyses might have limited power for detecting quantitative trait loci (QTLs) and mapping accuracy [4]. The cost of analyzing single nucleotide polymorphism (SNP) panels and the imbalance between individuals with genomic data and those without genomic data present additional limitations.
The weighted single-step (Wss)GWAS method has emerged as a powerful approach that leverages genomic estimated breeding values (GEBVs) derived from genotypes, phenotypes, and pedigree information to estimate the effects of SNPs [5]. This method effectively addresses the issue of unequal variances among SNPs, leading to more accurate estimation of SNP effects [6]. WssGWAS is more effective than GWAS in analyzing traits that are influenced by QTLs with significant effects or when there is insufficient phenotype and genotype data available. Recent studies have successfully used this approach to identified various economic traits in livestock species [7–9].
We investigate the genetic regions and candidate genes associated with productive traits (adjusted to 105 kg body weight) in Landrace pig using WssGWAS. Also, we conducted GO and KEGG enrichment analyses to gain deeper insights into the underlying biological processes and functional terms associated with the identified candidate genes for productive traits.
MATERIALS AND METHODS
This article does not require IRB/IACUC approval because there are no human and animal participants.
We obtained the total 37,099 productive records (9,818 males and 27,281 females) born from 2015 to 2021 at five Great-Grand-Parents (GGP) farms (Supplementary Table S1). We adjusted to evaluate for productive traits (AGE, ADG, BF, and eye muscle area [EMA] to 105 kg) with method outlined by the NIAS in Korea (https://www.nias.go.kr/images/promote/result/file/2021_2_5.pdf), and the equations used are as follows:
where α is the correction factor used to adjust AGE to 105 kg as follows:
ADG adjusted to 105 kg is calculated using the following equation:
BF adjusted to 105 kg is calculated using the following equation:
where β is the correction factor used to adjust BF to 105kg as follows:
EMA adjusted to 105 kg is calculated using the following equation:
where γ is the correction factor used to adjust EMA to 105kg as follows:
Illumina Porcine 60K V1 and V2 were used and V2 was selected as a reference panel for imputation. Prior to imputation, phasing was performed using Shapeit4 [10], a fast and accurate method for haplotype estimation using a positional Burrows-Wheeler transform (PBWT)-based approach to select informative conditioning haplotypes. Imputation was then conducted using Impute5 [11], assuming phased samples having no missing alleles. After imputation, quality control (QC) was performed by PLINK v1.09 [12] to exclude SNPs with low call rates (< 90%), low minor allele frequencies (< 0.01), or deviation from Hardy-Weinberg equilibrium (10−6). After QC, we used the number of animals and SNPs were 6,683 and 35,420, respectively.
We estimated the genetic parameters for AGE, ADG, BF, and EMA with average information restricted maximum likelihood (AIREML) method. We considered two approaches: pedigree-based best linear unbiased prediction (PBLUP) and single-step genomic best linear unbiased prediction (ssGBLUP). Each trait was estimated with a single-trait animal model, and the equation as follows:
where y is the vector of observations; b is the vector of fixed effects (herd-birth year-season, sex); a is the vector of additive genetic effects; e is the vector of residuals; and X and Z are the incidence matrices for b, a, and e. Heritability was estimated as , where and were additive genetic and residual variances, respectively.
Furthermore, GEBVs calculated using ssGBLUP approach, and marker effects were derived from these GEBVs. In contrast to the conventional BLUP approach, ssGBLUP substituted the inverse of the pedigree relationship matrix (A−1) with the inverse of the combined matrix H−1, which incorporated both the pedigree and genomic relationship matrices [13]. The H−1 can be represented as follows:
where is the inverse of numerator relationship matrix for pigs with genotyped, and G refers to the genomic relationship matrix [14]. G is presented below:
where Z is a matrix of gene content adjusted for allele frequencies (0, 1 or 2 for AA, Aa and aa, respectively), D is a diagonal matrix of weights for SNP variances (initially D = I), M is the number of SNPs, and pi is the minor allele frequency of ith SNP. Estimates of SNP effects and weights for WssGWAS were obtained according to following steps [5]:
-
First step (t = 1): D = I, , where [5]:
-
Calculate GEBVs;
-
Convert GEBVs to SNP effects , where ag was the GEBV of animal that was also genotyped;
-
Calculate the weight for each SNP: , where i was the ith SNP;
-
Normalize SNP weights to keep the total genetic variance constant:
-
t = t + 1 and loop to step 2.
The procedure was iteratively performed for a total of three cycles, taking into account the achieved accuracies of GEBV [15,16]. During each iteration, the weights of SNPs were updated (steps 4 and 5), and utilized to construct G matrices (step 6), update GEBV (step 2), and estimate SNP effects (step 3). Subsequently, the proportion of genetic variance explained by each consecutive set of SNPs, referred to as ith SNP windows, was calculated [16]. In a previous study, values for αi were determined based on linkage disequilibrium (LD) decay distance analysis of the population, considering the distance where r2 drops below 0.2 [17]. In this study, LD decay distance was not calculated separately, and to facilitate comparison with the previous study’s findings [17], the same value of 0.8 Mb was adopted. SNPs were positioned within a 0.8 Mb region, and the percentage of genetic variance explained by each 0.8 Mb window was determined as follows:
where αi is the genetic value of the ith SNP window that consisted of a region of consecutive SNPs located within 0.8 Mb, was the total additive genetic variance, Zj was the vector of gene content of the jth SNP for all individuals, and ûj was the effect of the jth SNP within the ith window. To visualize the distribution of these SNP windows, Manhattan plots were generated using the R software and CMplot package [18,19]. The procedures described above were implemented iteratively using the software suite of BLUPF90 programs [20].
We conducted to identify specific genomic regions associated with productive traits by examining QTL using genomic windows that accounted for more than 1.0% of the total genetic variance.
These genomic windows, previously employed in similar studies [17], represent regions of the genome that contribute significantly to the genetic variation underlying productive traits.
Our focus on these candidate QTL regions aimed to uncover genetic markers or regions that play a pivotal role in influencing growth-related characteristics. Notably, we observed a significant deviation from the expected average genetic variance explained by the 0.8 Mb window, which accounted for 0.0495% of the genetic variance on average (dividing 100% by the number of 2022 genomic regions). The 1% threshold exceeded the anticipated average genetic variance explained by the 0.8 Mb window by more than 20-times. To identify genes within the identified QTL regions, particularly within the significant windows, we utilized the ensemble Sus scrofa 11.1 database (https://www.ensembl.org/biomart). Furthermore, to gain deeper insights into the biological processes associated with these regions, we performed gene ontology (GO) and Kyoto Encyclopedia of Genes and Genomes (KEGG) analyses using the Database for Annotation, Visualization, and Integrated Discovery (DAVID v6.8, https://david.ncifcrf.gov/). GO terms and KEGG pathways showing significant enrichment were determined based on a p-value threshold of < 0.05. Through these analyses, we gained valuable knowledge regarding crucial molecular pathways and biological functions associated with the observed genetic variations.
RESULTS AND DISCUSSION
The estimates of the heritabilities for AGE, ADG, BF, and EMA were 0.49, 0.49, 0.56, and 0.23, respectively (Table 1). Results showed that the heritability of ssGBLUP was higher than that of PBLUP, which only used pedigree information. The ssGBLUP method, which incorporates both pedigree and genetic information, theoretically provides more accurate estimates of genetic parameters [7].
In most cases, major economic traits of livestock are quantitative traits except for some traits. These quantitative traits are characterized by a complex genetic structure. Exploration of candidate genes for such traits has always been an important goal of animal breeding programs. In this study, the genetic variance explained by a 0.8 Mb window for each growth trait was estimated using WssGWAS (Fig. 1). Specifically, we explained 2.05%, 3.23%, 9.27%, and 9.96% of the total genetic variation for AGE, ADG, BF, and EMA, respectively, with the most significant window explaining approximately 2.05%–2.34% of the total genetic variation (Table 2). Furthermore, within the identified window regions of this study, we presented the SNP markers, their corresponding chromosome (Chr), positions, and the associated genetic variance values explained by each marker (Supplementary Tables S2, S3, S4 and S5).
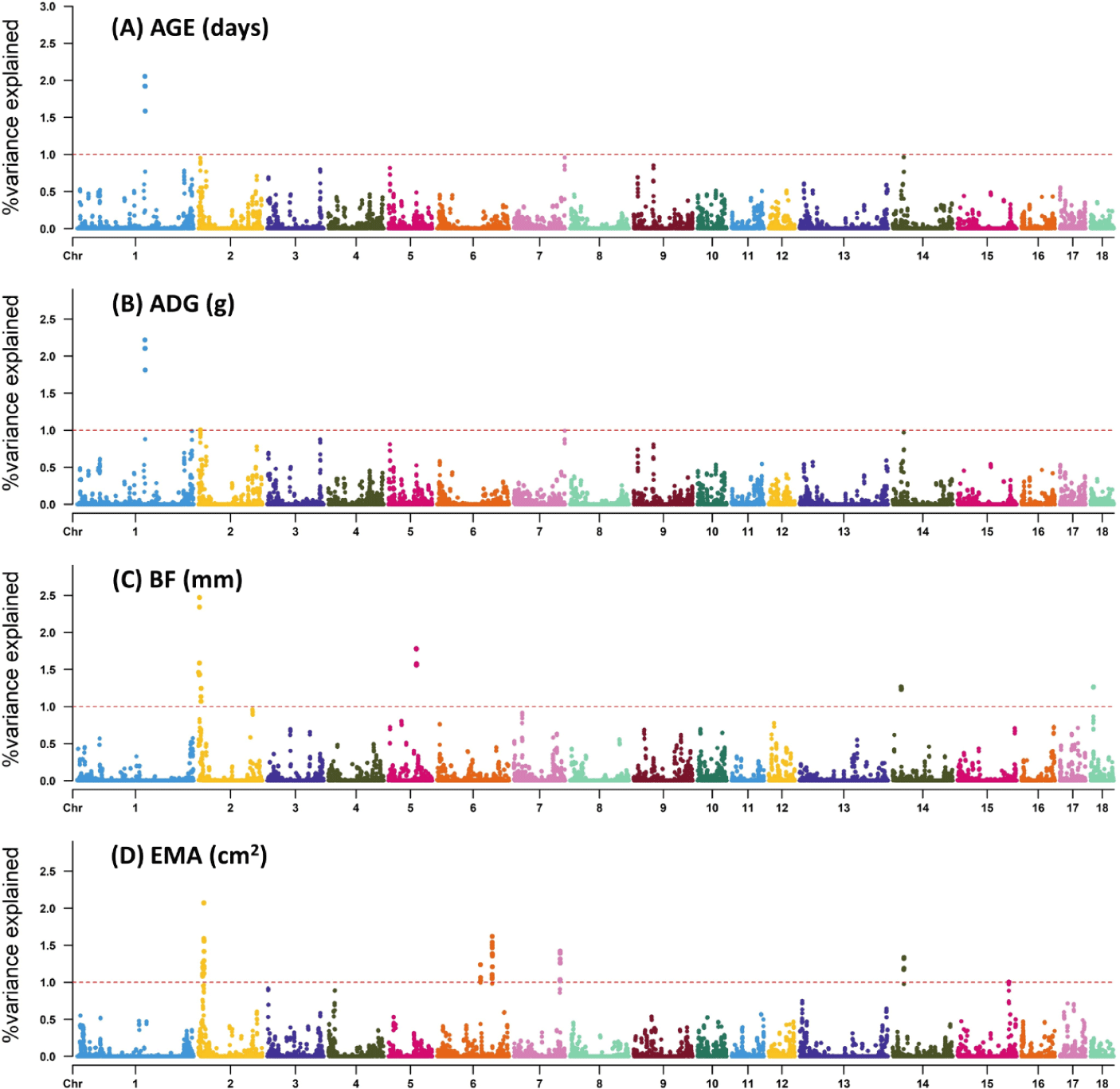
Previous GWAS studies have reported significance regions on Sus scrofa chromosome (SSC) 1, 3, 6, 8, and 13 for ADG and on SSC 1, 3, 6, 8, and 10 for AGE, explaining a total of 8.09% and 4.08% of the genetic variance, respectively [21]. Moreover, candidate QTL regions on SSC 4 and 14 for AGE, on SSC 4 and 2 for ADG, and on SSC 2, 3, and 10 for BF explain a total of 6.48%, 5.96%, and 6.76% of genetic variance, respectively [4]. The utilization of the WssGWAS, which incorporates SNP windows for genetic variance estimation, offers improved capabilities in identifying previously unknown QTLs compared to conventional GWAS methods. This approach mitigates the risk of overestimating the number of detected QTLs and false positives resulting from LD [22,23]. Furthermore, the iterative weighting of SNPs enhances the detection of QTLs with larger effects [16]. In this study, a total of 10 iterations were conducted, and the genomic accuracy for each trait was presented (Supplementary Table S6). As the number of iterations increased, there was a corresponding increase in genetic accuracy, consistent with previous study [5]. The highest increase was observed at the 3rd iteration, followed by a gradual decrease. Unlike the study that reported a decrease in weights at certain iterations [5], our study showed an increase in accuracy up to 0.02 to 0.04 over 10 iterations, as compared to the first iteration where all SNP weights were set to I. While the optimal number of iterations for each trait was not conclusively determined in our study, we chose to use the results from the 3rd iteration, which exhibited the highest genetic accuracy, for the GWAS analysis.
We have successfully identified three significant regions (SSC 1, 7, and 14) that are associated with AGE. These regions explain 1.03%–2.03% of the total genetic variance for AGE. Additionally, we conducted gene annotation and identified five genes with potential as candidate genes. Similarly, ADG is discovered five relevant QTL regions (SSC 1, 2, 7, and 14) that account for 1.01%–2.14% of the total genetic variance. Within these regions, we have annotated seven genes. Notably, although three QTL regions associated with AGE are also found to be associated with ADG, the proportions of genetic variance explained differ between the two traits.
When considering complex quantitative traits, it is important to acknowledge that linear gene effects may not consistently align with average trait values. Instead, a nonlinear assumption is often more appropriate [21], as gene contributions can exhibit nonlinearity and pleiotropic effects between traits may manifest [4]. Pleiotropic QTLs are prevalent in the porcine genome, as exemplified by the presence of QTLs associated with vertebral number, body length, and nipple number on SSC 7 [24]. Considering the overlap in the identified genomic regions and the substantial genetic correlation observed between ADG and AGE, it is reasonable to infer that the genes associated with these traits are shared.
Within the identified genomic regions, we observed the presence of RELCH in close proximity to MC4R on SSC 1. RELCH has been previously recognized as one of the seven potential candidate genes associated with pig fatness traits [25] and has demonstrated an association with pig fat depth [26]. Functionally, RELCH is involved in regulating intracellular cholesterol distribution, specifically from recycling endosomes to the trans-Golgi network. GO analysis further revealed enrichment in biological processes related to neuroactive ligand-receptor interaction [27]. These findings provide valuable insights into the potential regulatory mechanisms underlying fatness traits in pigs and highlight the role of RELCH in cholesterol metabolism and neuroactive signaling pathways.
RNF152 emerges as a promising candidate gene associated with pig fatness and body composition traits [25,26], specifically BF in Duroc pigs as revealed by ssGWAS analysis [28]. This gene acts as a negative regulator of the mammalian target of rapamycin (mTOR) signaling pathway [29,30], a key pathway governing cellular metabolism, survival, and proliferation through the regulation of anabolic processes such as protein, lipid, and nucleotide synthesis. The pivotal role of the mTOR pathway in cellular function has been extensively documented [31–34]. Our study highlights the potential pleiotropic effects within the SSC 1 region, which exhibited remarkable significance for both AGE and ADG traits. These findings provide valuable insights into the genetic architecture underlying productive traits and the interplay of key molecular pathways in pigs.
CDH20 has been identified as a candidate gene for pig fatness traits and days to reach 100 kg in previous studies [25, 35]. CDH20 encodes a type 2 classical cadherin, which is a calcium-dependent cell-cell adhesion glycoprotein and a potential candidate for tumor suppression [36]. Additionally, CDH20 is involved in the cell adhesion pathway. This study is the first to report its association with porcine growth and fatness traits [37].
TMEM132C has been identified as a potential candidate gene for growth and fatness-related traits in Bamaxiang pigs using a customized 1.4 million SNP array [38]. It has also been implicated as one of the candidate genes for average backfat at 100 kg [39].
NDUFV1 is located in the SSC 2 region and plays a critical role in energy metabolism [40]. Previous investigations have consistently demonstrated a significant downregulation of NDUFV1 expression in placental tissues, particularly when compared to the control group representing normal pregnancies. Notably, NDUFV1 plays a crucial role in facilitating energy production within the mitochondrial matrix and membrane, thereby influencing essential metabolic processes [41].
BF had the highest explained genetic variance and identified the highest number of candidate genes. Specifically, six relevant regions located on SSC 2, 5, 14, and 18 were identified, explaining 1.27%–2.34% of the total genetic variance, and 21 genes were annotated. EMA had lower heritability other traits such as AGE, ADG, and BF, but it was moderate heritability. Moreover, the significant genetic regions identified for EMA did not coincide with those found for BF, although SSC 2 and 14 exhibited similar levels of variance explained. Similar to BF, the region with the highest significant genetic variance explained was SSC 2 with 2.07% for EMA, while SSC 6, SSC 7, SC14, and SSC 15 were also identified as regions associated with EMA.
ANO1, also known as TMEM16A, is a Ca2+-activated chloride channel that plays a vital role in various physiological functions [42]. This channel is critical for maintaining the STT of urinary tract muscles in female mice and women. Sex differences in this context are likely influenced by ANO1 expression in SMCs of the urethra, and this gene is also involved in smooth muscle contraction [43,44].
PSMD13, also referred to as S11, Rpn9, p40.5, or HSPC027, is a 376 amino acid protein belonging to the proteasome subunit S11 family. It is located in the SSC 2 region and has been identified as being associated with loin depth in previous studies [45]. COX8H is a candidate gene situated in the SSC 2 region. It has been reported to explain 3.51% and 5.87% of the total genetic variation for BF and lean percent, respectively, in Yorkshire pigs [46]. Additionally, it has been identified as one of the highly expressed genes in intramuscular adipose tissues of Erhualian pigs [47]. MAP3K11 belongs to the serine/threonine kinase family and plays a crucial role in the FGFR signaling pathway, which regulates cartilage and bone formation [48]. Furthermore, a previous study has suggested a potential association between MAP3K11 and body weight in sheep [49].
AKAP3, located in the SSC5 region, is a member of the AKAP family. It interacts with the regulatory subunit of PKA [50]. While it has been predominantly studied in sperm and cancer, previous research has shown the expression of AKAP3 in the longissimus dorsi muscle of pigs [51]. The expression of AKAP3 in skeletal muscle and its binding to PKA’s regulatory subunit have the potential to affect glycogen content in the muscle, thereby impacting meat quality after post-mortem modifications [51]. FGF6 is a key regulator of skeletal muscle development that influences muscle fiber diameter and intramuscular fat content [52,53]. Additionally, FGF6 has been employed in gene delivery systems for skeletal muscle repair [54].
ZYX is located in the SSC18 region and is closely associated with multiple QTLs related to tissue and texture characteristics [55]. ZYX is a protein present in focal adhesions depending on active fibers and interacts with the actin-crosslinking protein alpha-actinin. ZYX is involved in cellular organization, signal transduction, cellular response to mechanical stress, and cell adhesion [56–59]. Structurally, ZYX consists of an N-terminal domain that interacts with proteins involved in signal transduction and a C-terminal LIM domain that plays a crucial role in regulating cell proliferation, differentiation, and protein-protein and/or protein-DNA interactions [60].
MED9, located in the SSC 2 region, is an essential gene for the maintenance of white adipose tissues and adipogenesis in Piscirickettsia salmonis [61]. MED9 also interacts with PPARs, which are important for inflammatory processes [62]. Polymorphism in the SERPING1 gene has been found to be significantly associated with tenderness and pH24 in both dominant and co-dominant models. Furthermore, this gene can influence the postmortem pH of muscle by regulating glycolysis [63].
Enrichment analyses uncovered significant associations between multiple terms and productive traits. Specifically, we observed enrichment in three biological processes, four cellular components, three molecular functions, and six KEGG pathways (Table 3). Notably, the most significant GO term was GO:0004190, which pertains to chromatin. Furthermore, the GO:0005509 category, encompassing calcium ion binding, exhibited enrichment for nine candidate genes, constituting the majority of the candidates.
The process of actin filament bundle assembly (GO:0051017) involves the construction of actin filament bundles with varying degrees of tightness and orientation. It represents a vital aspect of cellular structure and function. Notably, the selective sweep gene AIF1L emerged as a significant molecule, playing an essential role in cell survival and contributing to proinflammatory activities of immune cells, including monocytes/macrophages and activated T lymphocytes [64,65].
Chloride transmembrane transport (GO:1902476) refers to the movement of chloride across a membrane. Previous studies have implicated ANO9 as a gene associated with marbling depth in both purebred and crossbred pigs. The genetic region containing this gene accounts for 3.34% of the total genetic variance for loin depth [45]. Additionally, the CLCN1 gene participates in the transmission of nerve impulses, a crucial cellular communication process involved in the interaction between adipocytes and myogenic cells [66]. The interplay between these cell types is significant for various aspects of growth and development, including the regulation of myogenesis rate and extent, muscle growth, adipogenesis, lipogenesis/lipolysis, and energy substrate utilization [67].
Calcium ion binding (GO:0005509) denotes the process of binding to a calcium ion (Ca2+). Prior research has identified EHD1 as a candidate gene that likely possesses functional relevance to meat quality in Beijing black pigs [68]. Additionally, a GWAS study revealed a significant association between EHD1 and the meat-to-fat ratio (MFR) [69]. Furthermore, using EHD1 knockout mice, researchers demonstrated the regulatory role of EHD1 in cholesterol homeostasis and lipid droplet storage [70].
In conclusion, this study offers novel insights into the genetic basis of productive traits in pigs. The identified biological processes, pathways, and candidate genes serve as valuable resources for future investigations for genetic improvement with these traits. Significant SNPs can be used as markers for QTL investigation and genomic selection (GS) for productive traits in Landrace pig.