INTRODUCTION
The Kele pig is a precious Chinese indigenous pig breed with disease resistance and economic value found in Guizhou Province located in the Karst mountainous area. Porcine respiratory disease complex (PRDC) is the most important and economically significant disease in the Chinese pig industry, and the economic loss caused by this disease is also the most serious in pig farms. As far as the incidence of pig farms worldwide is concerned, PRDC is generally a mixed infection of multiple pathogens [1]. The dual infection of porcine reproductive and respiratory syndrome virus (PRRSV) and Haemophilus parasuis (HPS) is epidemic and severe in China [2], resulting in the excessive use of antimicrobials and antivirals and causing reduced pig survival, feed conversion, and pork quality. Previous studies that focused on single pathogen invasion [3,4] and host defense mechanisms [5,6] were mainly concerned with protein-coding genes. Most studies on PRRSV and HPS co-infection only focus on molecular biological detection [7,8], comparisons of clinical symptoms and pathological changes of target organs [9], and immune responses in vitro [10,11]. According to our previous clinical observation and research, the results preliminarily suggested that Kele pigs have some ability to resist PRRSV and HPS concurrent invasion [12]. More information is still needed to better understand the host immune responses to PRRSV and HPS co-infection in Kele pigs.
Non-coding RNAs, including microRNAs (miRNAs) and long non-coding RNAs (lncRNAs), have been gradually recognized as key regulatory factors involved in the pathogenesis of PRDC. Some miRNAs were reported in pigs infected with PRRSV [13], porcine circovirus type 2 (PCV2) [14], Mycoplasma hyopneumoniae [15], and HPS [16]. The above studies have shown that miRNAs play critical roles in pathogen invasion, gene expression regulation, cell growth, differentiation, and apoptosis, immune response pathways. An increasing number of lncRNAs in the studies of HPS [17], PCV2 [18], and PRRSV [19,20] were revealed to function as crucial regulators of an immune response, and dysregulation of lncRNAs may also lead to disease. Furthermore, based on the competitive endogenous RNA (ceRNA) hypothesis, lncRNAs harboring miRNA response elements (MREs) act as miRNA “sponges”, which can compete with miRNA target genes for shared miRNAs. These MRE-sharing elements form the post-transcriptional ceRNA network to regulate mRNA expression [21]. Therefore, an efficient way to infer the potential function of miRNAs and lncRNAs related to pathogen infection and the host immune response is by exploring their relationships with annotated mRNAs.
To elucidate the mechanisms of resistance to PRDC pathogen invasion in Kele pigs for disease resistance breeding, it is necessary to research the Kele pig immune response to these two pathogens and the potential effect of differentially expressed transcripts on the development of a protective immune response against PRRSV and HPS co-infection. In this study, with an established PRRSV and HPS infection model, transcriptome sequencing was performed to explore the differences in miRNA and lncRNA expression patterns and functions between PRRSV and HPS infection alone and co-infection, and particularly the changes after co-infection. The functions of the target genes of the differentially expressed miRNAs (DEmiRNAs) and differentially expressed lncRNAs (DElncRNAs) were analyzed and a ceRNA regulatory network was constructed in the PRRSV and HPS co-infection group. Furthermore, the selected miRNAs and lncRNAs were validated by real-time quantitative polymerase chain reaction (RT-qPCR) and dual-luciferase reporter assays. This revealed a novel posttranscriptional regulation perspective that could help us understand the crucial roles of non-coding RNAs for regulating gene expression in immune resistance to PRRSV and HPS co-infection.
MATERIALS AND METHODS
All animal procedures were conducted in accordance with the National Research Council Guide for the Care and Use of Laboratory Animals and approved by the Animal Ethics and Research Committee of Guizhou University (EAE-GZU-2020-P008).
The viruses and bacteria used in this study were the same as in a previously published study [12]. The titer of the PRRSV genotype 2 GZBJ12 strain was 105.675 Tissue Culture Infective Dose (TCID50)/mL. The concentration of the HPS serotype 4 GZ strain inoculum was 1.66 × 109 CFU/mL.
Thirteen 5-week-old Kele piglets were selected and divided into four groups (There were four piglets in the PRRSV–HPS group and three piglets in the HPS, PRRSV and control groups, respectively.). The piglets were inoculated with pathogens and managed for feeding as described in a previous study [12]. All animals were euthanized after 10 d post-infection. Pentobarbital sodium salt (Sigma, MO, USA) was used to relieve the pain of experimental animals. Lung tissues were collected, homogenized, and mixed with Trizol (TaKaRa, Kusatsu, Japan) for total RNA and stored at −80°C.
Total RNA was extracted from 13 frozen lung tissue samples using Trizol (Invitrogen, Carlsbad, CA, USA) according to the manufacturer’s instructions. The RNA was used for miRNA and RNA sequencing on an Illumina platform at Novogene Technology (Beijing, China). After quality validation using an Agilent 2100 Bioanalyzer (Agilent Technologies, Santa Clara, CA, USA) and gel electrophoresis, the small RNA library was constructed using the NEBNext® Multiplex Small RNA Library Prep set for Illumina® (New England Biolabs, New Ipswich, MA, USA), and its quality was assessed on an Agilent 2100 Bioanalyzer system (Agilent Technologies). Then, clustering of the index-coded samples was performed on a cBot Cluster Generation system using a TruSeq SR Cluster kit v3-cBot-HS (Illumina, San Diego, CA, USA) and the preparation libraries were subjected to deep sequencing. The detailed procedure of the library construction and quality control for RNA sequencing were carried out according to our related report [12].
The small RNA sequencing clean data were obtained by removing reads containing ploy-N, with 5’ adapter contaminants, without 3’ adapter or the insert tag, containing ploy A or T or G or C and low quality reads from raw data by using custom scripts. And then unique sequences with length in 18–26 nucleotide were mapped to the reference genome (Suscrofa 10.2.72) by Bowtie (-v 0 -k 1) [22] for further annotation analysis. Next, miRBase20.0 [23] was used as reference, the known miRNAs were analyzed by miRDeep2 (quantifier.pl -p -m -r -y -g 0 -T 10) [24]. After removing protein-coding genes, repeat sequences, rRNA, tRNA, snRNA, and snoRNA, small RNA tags by RepeatMasker (-species -nolow -no_is -norna -pa 8) and Rfam databases, the novel miRNAs were predicted using miREvo (-i -r -M -m -k -p 10 -g 50000) [25].
The raw RNA-sequencing data were filtered and quality control was carried out according to our previous study [12]. Novel potential lncRNA transcripts were identified following below steps: (1) Filter the transcripts with shorter than 200 base pair (bp); (2) Filter the transcripts with single exon; (3) Filter the known coding protein transcript; (4) Filter the transcripts with Fragments Per Kilobase of per Million fragments mapped (FPKM) < 0.5 and p < 0.05; (5) Coding-Non-Coding-Index (CNCI, score < 0) [26], Coding Potential Calculator (CPC, score < 0) [27], Pfam-scan (-E 0.001 --domE 0.001) [28], and phylogenetic codon substitution frequency (phyloCSF, --orf = ATGStop -frames = 3) [29] were used to distinguish mRNAs from lncRNAs, transcripts with coding potential predicted by any one of these four tools were filtered. Finally, those passing through all steps were filtered as the candidate set of lncRNAs.
The miRNA and lncRNA expression levels were estimated by Transcripts Per Million reads (TPM) and FPKM, respectively. Differential expression analysis between challenged and normal groups was performed using DESeq2 [30]. MiRNAs with a log2|fold change| > 1 and p < 0.05 between the two groups (PRRSV–HPS vs. Control, PRRSV vs. Control, and HPS vs. Control) were identified as differentially expressed. DElncRNAs were identified between the two groups (PRRSV–HPS vs. Control, PRRSV vs. Control, and HPS vs. Control) by log2|fold change| > 2 and q < 0.05 according to the methods of our related transcriptome sequencing study, and differentially expressed genes (DEGs) were used in this study from our published data [12].
Predicting the target genes of the DEmiRNAs was performed by miRanda [31] with parameters: -sc 140 -en 10 -scale 4 -strict; and RNAhybrid [32] with parameters: -e 10 -p 0.05 -m 50,000; and the common targets were the final results. Target gene prediction of DElncRNAs was performed according to two roles: (1) Cis-role of the target gene prediction: a cis-role is the lncRNA acting on neighboring target genes. We searched coding genes 100,000 bp upstream and downstream of the lncRNA. (2) Trans-role of the target gene prediction: a trans-role is the lncRNA identifying non-neighboring genes by the expression level of correlation with Pearson correlation coefficient (PCC) |R| > 0.95 and p < 0.05. The expressed correlation between lncRNAs and coding genes was calculated by “cor.test” in R.
The Gene Ontology (GO) and Kyoto Encyclopedia of Genes and Genomes (KEGG) enrichment analyses were performed using KOBAS software [33] with the hypergeometric test and p < 0.05 considered significantly enriched. Furthermore, Cytoscape3.7.2 [34] ClueGO plug-in was used to build the GO-target gene networks.
Clearly establishing the potential regulatory relationship between lncRNA-miRNA-mRNA gene pairs was based on the sequence complementarity and PCC (R > 0.5 or R < −0.5, and p < 0.05) between the shared miRNA and target mRNA or target lncRNA, as well as the co-expression relationship between the lncRNA and mRNA. The regulatory network of the ceRNAs was analyzed, and the network was constructed by Cytoscape3.7.2.
The RT-qPCR validation was performed with the total RNA from lungs used in the transcriptome sequencing analysis with a PrimeScriptTM RT reagent kit (Perfect Real Time) (Takara). cDNA was used for RT-qPCR with TB Green® Premix Ex Taq™ II (Tli RNaseH Plus, Takara), following the instruction manual. Reactions were performed on an Eppendorf realplex Sequence Detection system (Eppendorf, Hamburg, Germany). Triplicate wells of reactions (25 µL) contained 12.5 µL of TB Green Premix Ex Taq II (2×), 2 µL of 50 ng/µL cDNA, 1 µL of 10 µM of each primer, and 8.5 µL of ddH2O. The RT-qPCR conditions were 95°C for 30 s, followed by 40 cycles at 95°C for 10 s, 60°C for 30 s, and 72°C for 20 s, followed by a melt curve. Ten DEmiRNAs and ten DElncRNAs were selected randomly from the three infected groups after being filtered and identified by sequencing. U6 [16] and GAPDH [20] were chosen as the internal reference genes for miRNAs and lncRNAs, and the 2−∆∆Ct method was used to calculate the fold change for gene expression. All primers of the selected genes were synthesized by Sangon Biotech (Shanghai, China) and are shown in Supplementary Table S1.
To verify the effects of ssc-miR-671-5p on target genes, the full length sequence of DTX4 and AEBP1 3’-UTRs were cloned into pmirGLO vector (Promega, Madison, WI, USA) downstream of the firefly luciferase gene, respectively. The Renilla luciferase gene was expressed as a reference reporter in the pmirGLO vector. Luciferase activity was measured using a Dual-Luciferase Reporter Assay System (Promega) according to the manufacturer’s instructions. Briefly, PmirGLO-DTX4-3’-UTR wild type (wt), PmirGLO-DTX4-3’-UTR mutated type (mt), PmirGLO-AEBP1-3’-UTR wt, or PmirGLO-AEBP1-3’-UTR mt was transfected into HEK293 cells along with ssc-miR-671-5p mimics or negative control miRNA mimics (mimics NC) (Genecrete, Wuhan, China) in 24-well plates using Lipofectamine 2000 (Invitrogen) according to the manufacturer’s instructions. The Dual-Luciferase Reporter Assay kit (Beyotime, Shanghai, China) and GloMax 96 Microplate Luminometer (Biosino, Beijing, China) were used to detect firefly and Renilla luciferase activities. Luciferase activity was normalized to Renilla luciferase activity at 48 hours after transfection.
Three replicates of RT-qPCR and dual luciferase reporter assays were performed for each sample. The results of dual-luciferase reporter assays were presented as means ± SD, p values were calculated using Student’s t test between two groups, and values of p < 0.05 were considered to indicate a statistically significant difference.
RESULTS
To reveal the expression difference of non-coding RNAs in lungs from PRRSV- and HPS-infected pigs, 13 small RNA libraries and 13 cDNA libraries were constructed and sequenced. For small RNA sequencing, the number of raw reads ranged from 0.786 G to 1.113 G (Supplementary Table S2). Q20 was above 99.19 % and Q30 was above 97.34 %, which indicated high accuracy of the sequencing data. Clean reads accounted for 98.85%–99.32% of the raw reads. The length of the clean reads was mainly from 20 nt to 24 nt, which is in accordance with the general length range of miRNAs. Of the 360 annotated mature miRNAs and 135 novel miRNAs, 326 miRNAs were transcribed in all sequenced individuals.
The lncRNA sequencing showed that 12,029 annotated lncRNAs and 17,338 novel lncRNAs were identified in all sequenced individuals. These identified lncRNAs were characterized as shorter in exon length, with fewer exons, shorter open reading frames (ORFs), and lower expression levels than protein-coding genes (Supplementary Figs. S1A, S1B, S1C, and S1D). Among the lncRNAs, 51.1% were intronic lncRNAs, 34.4% were lncRNAs, and 14.5% accounted for antisense lncRNAs (Supplementary Fig. S2).
DEmiRNAs and DElncRNAs were identified to understand the non-coding RNA changes in different groups. Compared with controls, 13 significant DEmiRNAs (10 upregulated miRNAs and 3 downregulated miRNAs) and 159 significant DElncRNA (66 upregulated lncRNAs and 93 downregulated lncRNAs) were identified in the HPS group (Figs. 1A and 1D). There were 20 significant DEmiRNAs (11 upregulated miRNAs and 9 downregulated miRNAs) and 122 significant DElncRNAs (79 upregulated lncRNAs and 43 downregulated lncRNAs) in the PRRSV group (Figs. 1B and 1E), and 36 significant DEmiRNAs (20 upregulated miRNAs and 10 downregulated miRNAs) and 225 significant DElncRNAs (144 upregulated lncRNAs and 81 downregulated lncRNAs) in the PRRSV–HPS group (Figs. 1C and 1F).
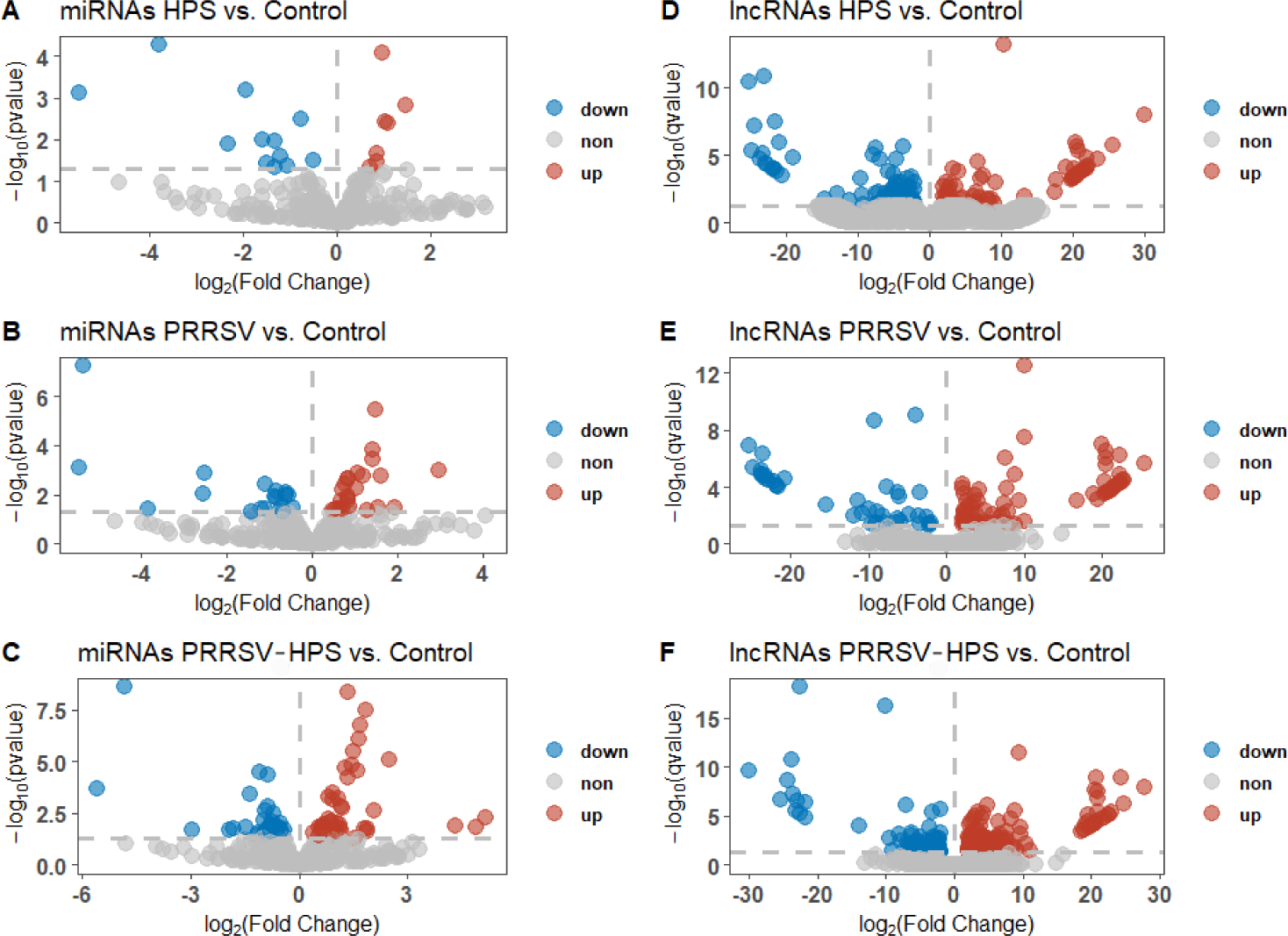
As shown in the Venn diagrams of the differentially expressed miRNAs and lncRNAs, there were 15 shared DEmiRNAs in the three infected groups, and 17 unique DEmiRNAs, including two novel miRNAs (novel_209, novel_494), in the PRRSV–HPS group (Fig. 2A), 92 shared DElncRNAs in the three infected groups, and 146 unique DElncRNAs in the PRRSV–HPS group (Fig. 2B). This showed that unique miRNAs and lncRNAs were regulated in the PRRSV–HPS group, which may indicate a potential function with PRRSV and HPS dual infection.
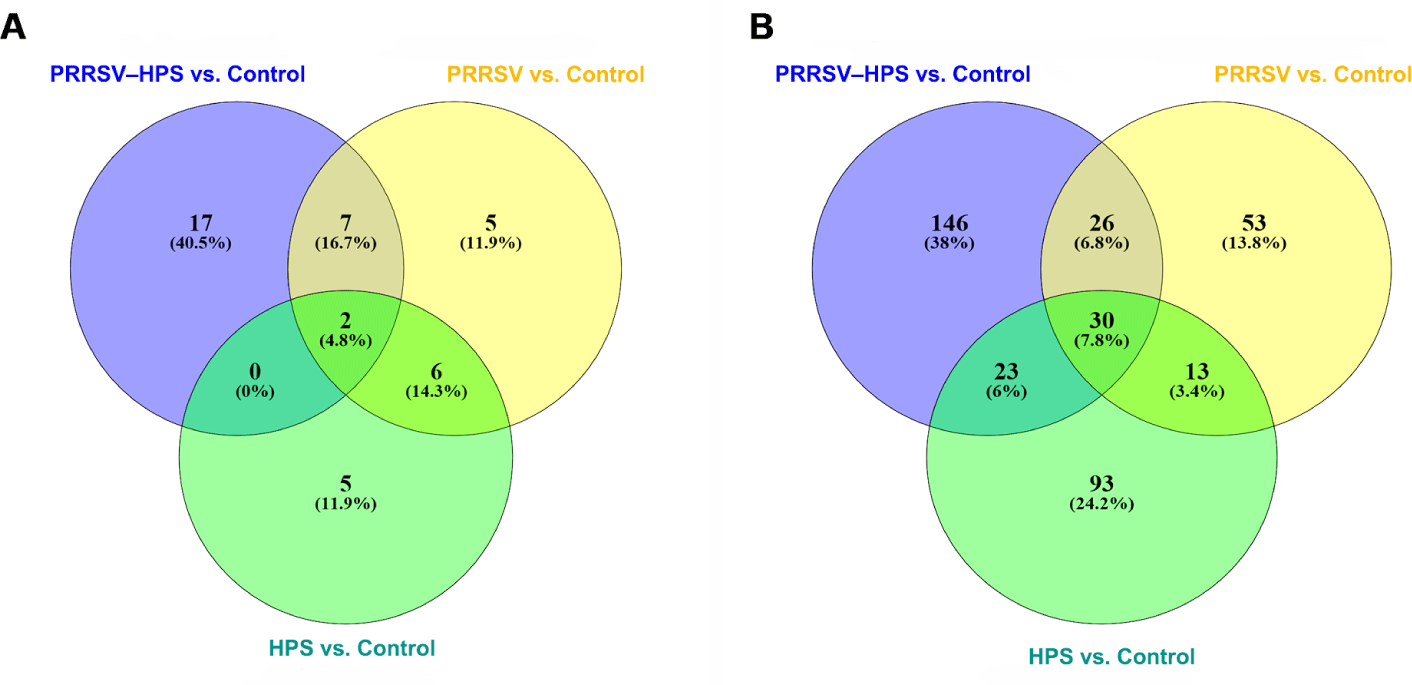
Non-coding RNAs play a role by affecting gene expression and by regulating biological processes and signaling pathways. Therefore, the primary method to explore DEmiRNAs and DElncRNAs is to predict their target genes and functions. The target genes of miRNAs were identified by miRanda and RNAhybrid, with 16, 58, and 115 target DEGs of DEmiRNAs in the HPS (SupplementaryTable S3A), PRRSV (Supplementary Table S3B), and PRRSV–HPS groups (Supplementary Table S3C), respectively. Meanwhile, potential cis-regulated and trans-regulated target genes of DElncRNAs were explored. In total, 30 cis-regulated and 90 trans-regulated DEGs were found in the HPS group (Supplementary Table S4A), 27 cis-regulated and 37 trans-regulated DEGs were found in the PRRSV group (Supplementary Table S4B), and 71 cis-regulated and 135 trans-regulated DEGs were found in the PRRSV–HPS group (Supplementary Table S4C). The Circos plots of DEmiRNAs, DElncRNAs, and differentially expressed target genes in the HPS (Supplementary Fig. S3A), PRRSV (Supplementary Fig. S3B), and PRRSV–HPS (Fig. 3A) groups were visualized, which showed a more complicated non-coding RNA and target gene regulation pattern in the PRRSV–HPS group.
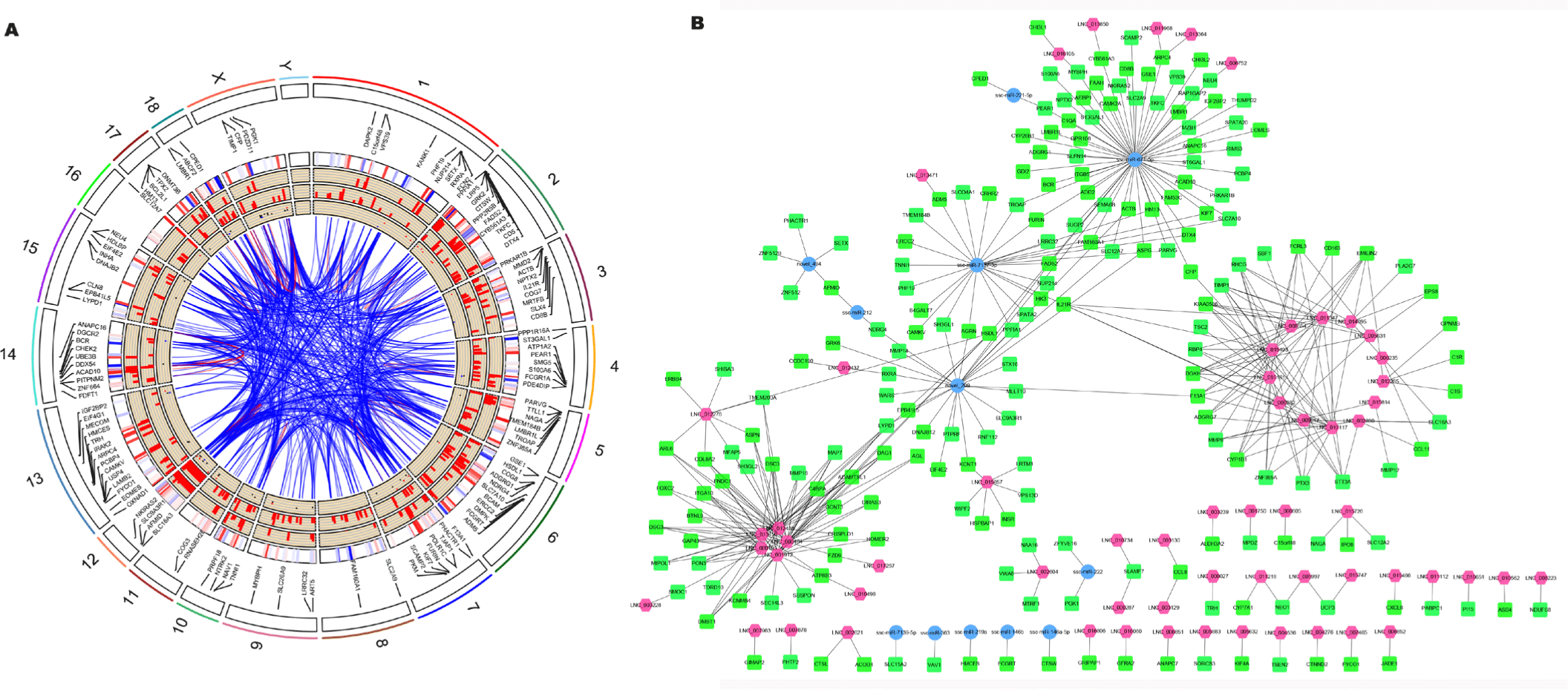
Furthermore, compared with the HPS and PRRSV single-infection groups, 206 target DEGs were predicted by the unique 146 DElncRNAs and 17 DEmiRNAs in the PRRSV–HPS group (Fig. 3B), and some DEGs related to pathogen infection and the immune system were regulated. For example, matrix metalloproteinases (such as MMP8; log2 (fold change) = 22.825, q = 5.33e-06) and their inhibitors (such as TIMP1; log2 (fold change) = 3.981, q = 0.0017), associated with pulmonary fibrosis, were regulated by LNC_002890, LNC_005631, LNC_008554, LNC_009017, LNC_010161, LNC_011347, LNC_015493, LNC_015814, and LNC_017117; CD163 molecule (CD163; log2 (fold change) = 2.154, q = 0.0017), the membrane protein receptor of PRRSV, was regulated by LNC_008554 and LNC_017117; T lymphocyte surface glycoprotein beta chain (CD8B; log2 (fold change) = 2.178, q = 0.044), calcium/calmodulin-dependent protein kinase II alpha (CAMK2A; log2 (fold change) = -4.913, q = 0.039), and complement c1q A chain (C1QA; log2 (fold change) = 8.760, q = 0.00012) were regulated by ssc-miR-671-5p; and interleukin 21 receptor (IL21R; log2 (fold change) = 2.260, q = 0.033) was regulated by LNC_000282, LNC_015493, LNC_017117, and ssc-miR-7137-3p. The results indicated that the regulated non-coding RNAs might potentially regulate the expression of genes to influence pathogen invasion and the host immune response against PRRSV and HPS infection.
Differentially expressed lncRNAs and miRNAs are involved in the immune response pathways in the concurrent infection group
Next, we investigated whether the target DEGs of DElncRNAs and DEmiRNAs from PRRSV–HPS group influenced the immune response. The possible enrichment pathways and biological processes of the target DEGs were predicted and analyzed. The GO enrichments demonstrated that more immune-related biological processes were present in the PRRSV–HPS group compared with the HPS and PRRSV single-infection groups. The top 20 enrichments are shown in Supplementary Table S5. Eight immune biological processes were significantly enriched in the unique DElncRNAs and DEmiRNAs of the PRRSV–HPS group, including complement activation (GO: 0006956), interaction with host (GO: 0051701), and B cell-mediated immunity (GO: 0019724) (Fig. 4). These immune processes were activated by two special miRNAs (novel_209 and ssc-miR-671-5p) and 16 lncRNAs (including LNC_012285, LNC_014095, and LNC_015466), which regulated 13 DEGs, including C1S (log2 (fold change) = 2.071, q = 0.00054), CD46 (log2 (fold change) = 2.071, q = 0.00054), C1QA (log2 (fold change) = 8.760, q = 0.00012), CXCL8 (log2 (fold change) = 2.833, q = 0.0013), and CD163 (log2 (fold change) = 2.154, q = 0.0017).
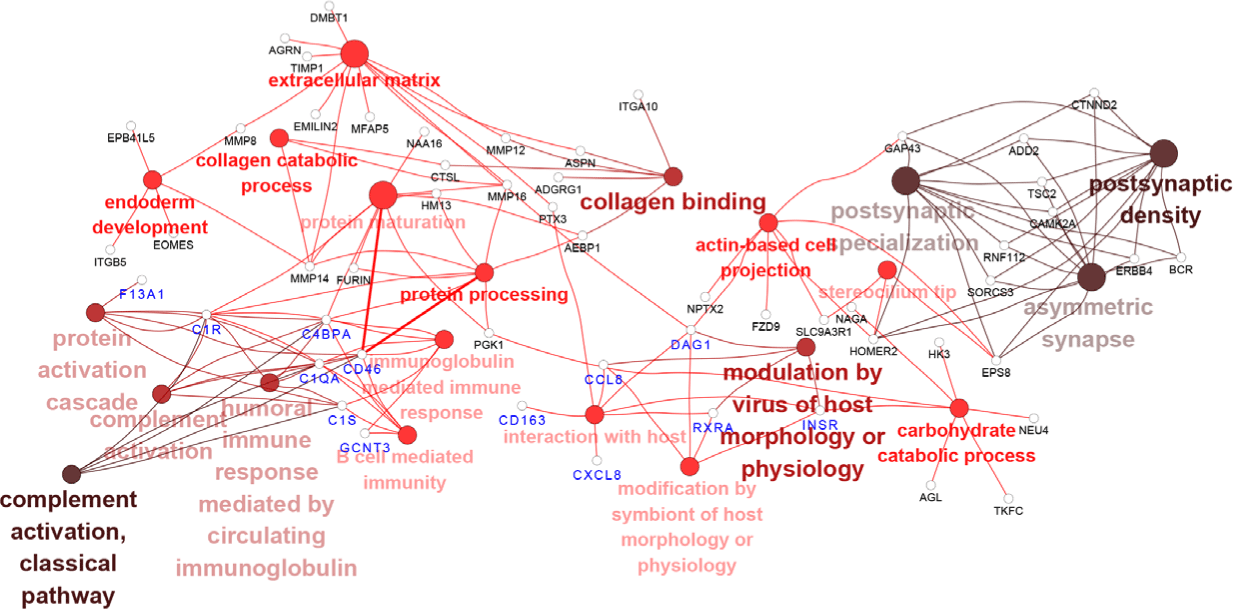
KEGG pathway enrichment showed that important immune pathways were regulated by the target genes of regulated non-coding RNAs in the three infection groups (Supplementary Table S6). For example, in the networks of the miRNA/lncRNA-KEGG pathway, the HPS group was enriched in adherens junction (ssc-miR-21-3p–PTPRF [log2 (fold change) = 3.121, q = 2.88e-08]), and viral protein interaction with cytokine and cytokine receptor and Toll-like receptor signaling pathway (LNC_001726–IL6 [log2 (fold change) = 4.929, q = 0.0045]; LNC_015081, LNC_015082–CXCL10 [log2 (fold change) = 2.57, q = 0.0011], CXCL11 [log2 (fold change) = 3.104, q = 0.040]) (Fig. 5A). Cell adhesion molecules (LNC_001035–CD274 [log2 (fold change) = 5.544, q = 0.0091] and LNC_014466–SLA-5 [log2 (fold change) = −20.992, q = 0.00017]), antigen processing and presentation (LNC_002022–CTSL [log2 (fold change) = 3.882, q = 0.00023]), and salmonella infection (ssc-miR-4331-3p–CCL3L1 [log2 (fold change) = 2.114, q = 1.46e-06]) were significantly enriched in the PRRSV group (Fig. 5B). The KEGG pathways of the HPS and PRRSV single-infection groups indicated that the HPS or PRRSV infection influenced the immune system through regulated miRNAs and lncRNAs.
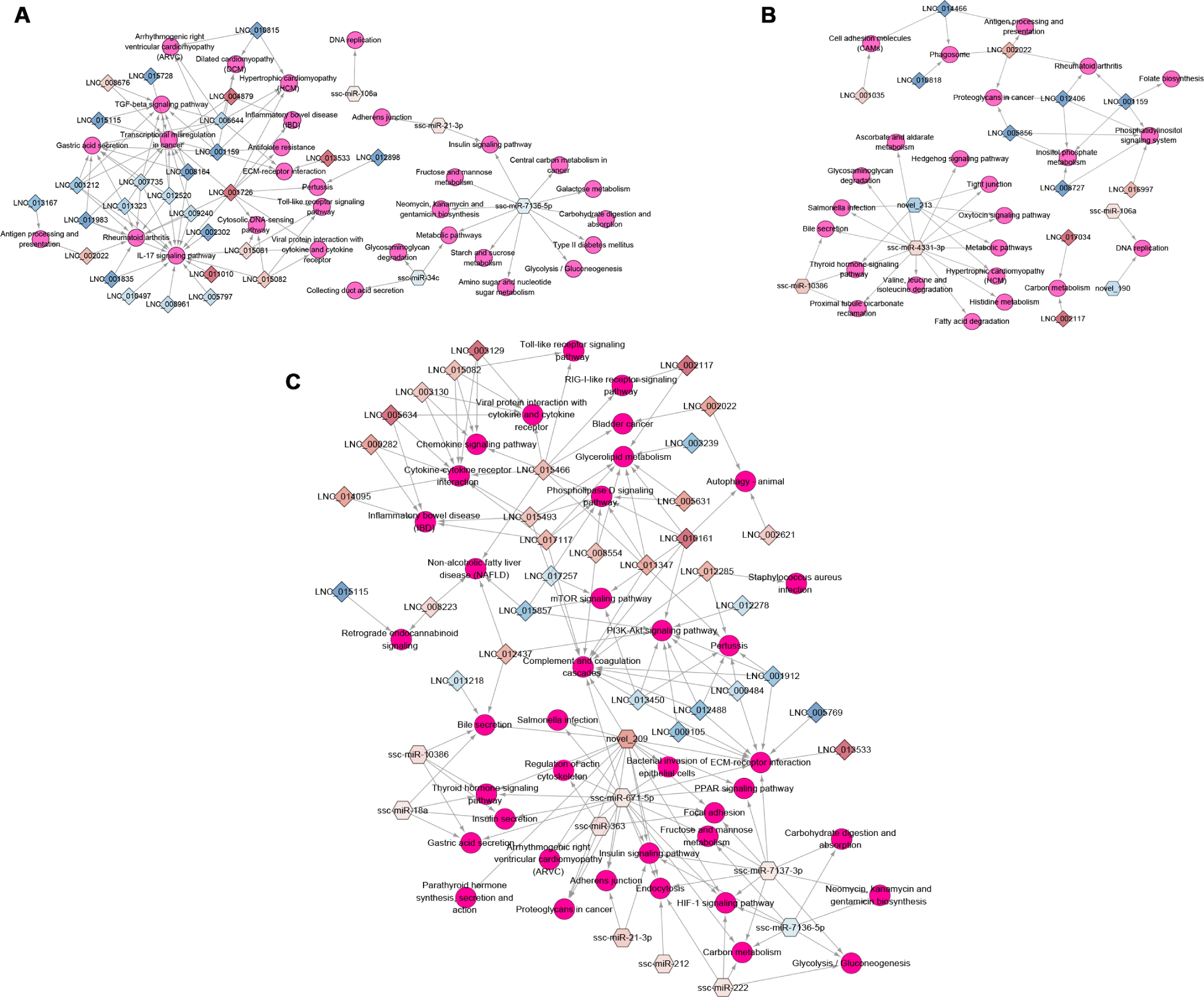
Compared with the HPS and PRRSV single-infection groups, more DEmiRNAs and DElncRNAs regulated immune-related KEGG pathways in the PRRSV–HPS group (Fig. 5C), such as ECM–receptor interaction, bacterial invasion of epithelial cells, and complement and coagulation cascades, which were enriched by novel_209, ssc-miR-671-5p, ssc-miR-7137-3p, LNC_015466, and LNC_017117. Detailed descriptions of the immune-related pathways in the PRRSV–HPS group by targets of unique DElncRNAs and DEmiRNAs are listed in Table 1, suggesting that they may affect complement and coagulation cascades and the HIF-1 signaling pathway to activate the host immune defense mechanisms. Across these results are regulated non-coding RNAs that influence or take part in the immune biological processes and signaling pathways to defend against PRRSV and HPS invasion, and more immune response pathways were enriched in the PRRSV–HPS group compared with the single-infection groups.
Next, we investigated the regulatory network between differentially expressed non-coding RNAs and target genes based on the “ceRNA hypothesis.” The lncRNA–miRNA–mRNA networks with the sequence complementarity and high PCC of expression levels between miRNAs and their targets (lncRNA or mRNA) were constructed for the HPS, PRRSV, and PRRSV–HPS groups (Supplementary Fig. S4), which revealed the more complicated lncRNA–miRNA–mRNA network in the PRRSV–HPS group.
Furthermore, based on the sequence complementarity and negative correlation between DEmiRNAs and their targets, as well as the co-expression relationship between lncRNA and mRNA, the ceRNA network was constructed and analyzed in the PRRSV-HPS group (Fig. 6). The result showed that ssc-miR-671-5p (log2 [fold change] = 1.183, p = 0.0018) was the hub miRNA of the regulatory network, which regulated four downregulated lncRNAs (LNC_001016, LNC_002194, LNC_003637, and LNC_009394) and five downregulated mRNAs, including DTX4 (log2 [fold change] = −26.266, q = 6.09e-08), CAMK2A (log2 [fold change] = −4.913, q = 0.039), FAM160A1 (log2 [fold change] = −25.821, q = 1.05e-07), SLC2A9 (log2 [fold change] = −6.597, q = 0.027), and AEBP1 (log2 [fold change] = −27.228, q = 1.76e-08). The novel_207 (log2 [fold change] = −2.936, p = 0.018) was the only miRNA with downregulated expression that regulated ADM5 (log2 [fold change] = 2.370, q = 0.000026). In addition, the effect of ssc-miR-106a, ssc-miR-20b, ssc-miR-7139-5p, and ssc-miR-2411 on the expression of ten lncRNAs (such as LNC_009394, LNC_003226, and LNC_003637) formed a closed regulatory network.
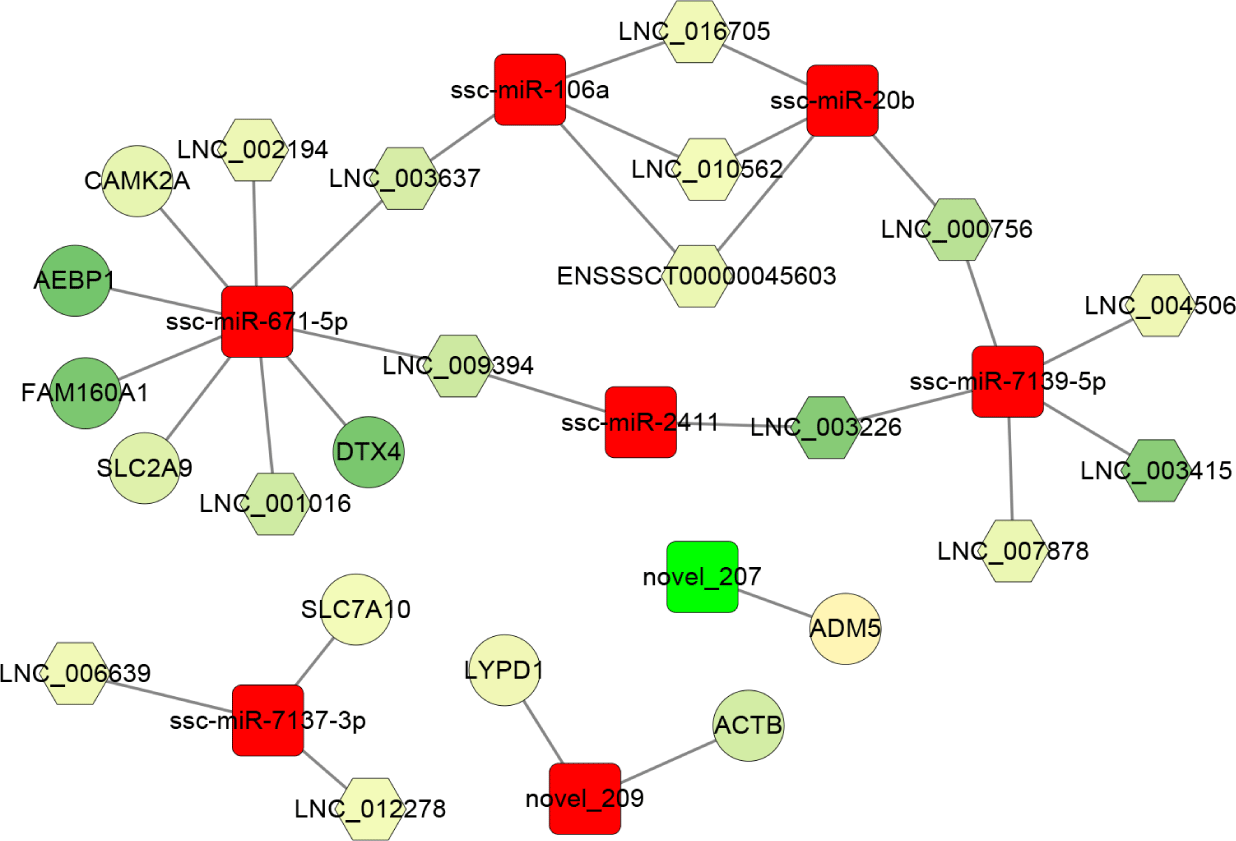
To validate the expression pattern of DEmiRNAs and DElncRNAs identified from RNA-seq, ten DEmiRNAs and ten DElncRNAs were selected for RT-qPCR assays with the U6 and GAPDH genes as the internal controls. Among the selected DEmiRNAs and DElncRNAs, seven DEmiRNAs (including ssc_miR-21-3p, ssc_miR-371-5p, and novel_209) and eight lncRNAs (including LNC_015980, LNC_002561, and LNC_004879) showed a consistent upregulated trend in the results of both RT-qPCR and RNA-seq. Three DEmiRNAs (ssc_miR-202-5p, novel_611, and novel_207) and two DElncRNAs (LNC_009367 and LNC_002788) were downregulated in both RT-qPCR and RNA-seq (Fig. 7A). Although the extent of the fold change varied between RT-qPCR and RNA-seq, the RT-qPCR assay confirmed the results of RNA-seq, and the methods displayed a strong correlation (miRNAs: R2 = 0.853, lncRNAs: R2 = 0.936) (Figs. 7B and 7C), indicating the high reliability of RNA-seq data.
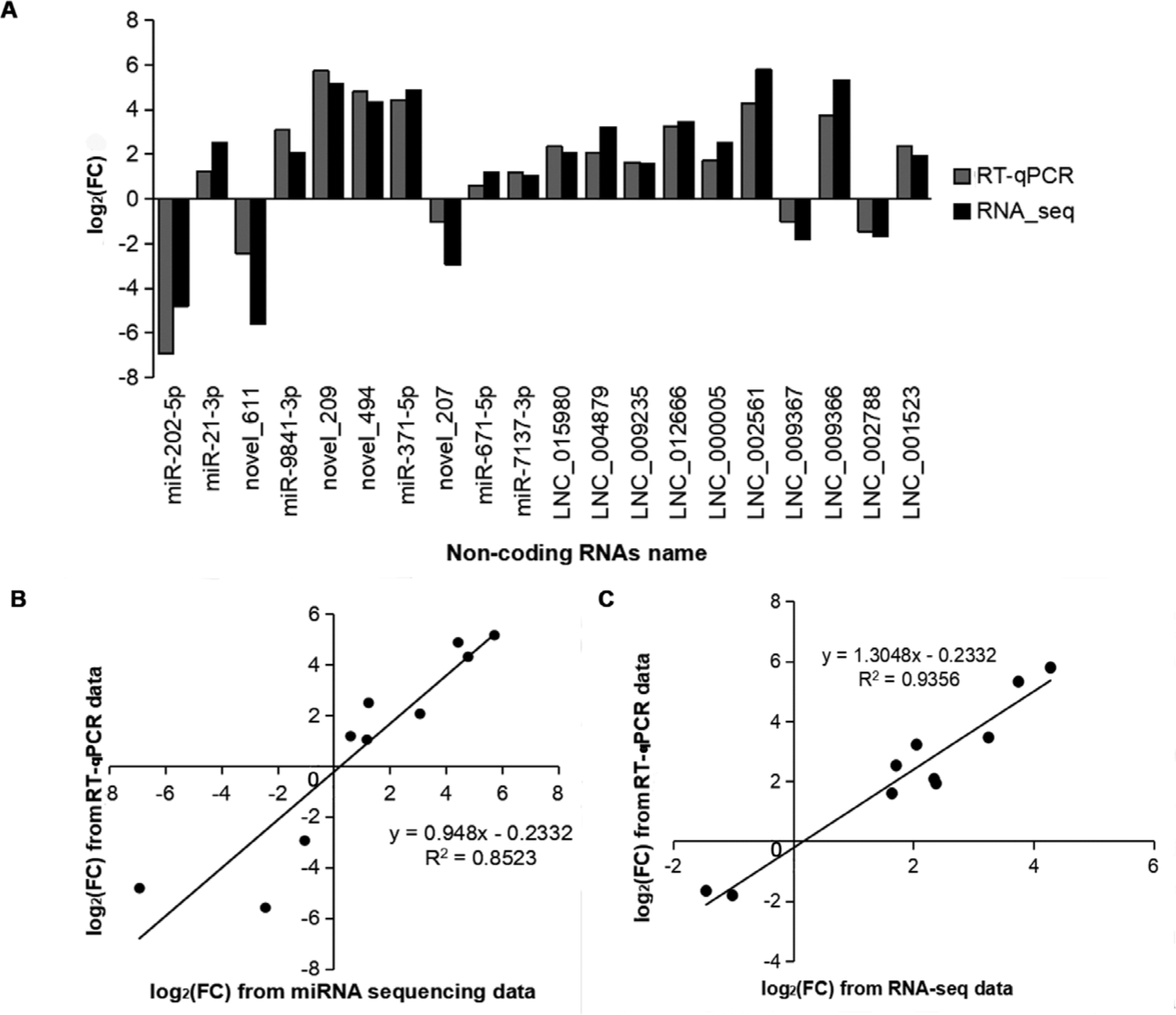
To validate the predicted ssc-miR-671-5p target genes (DTX4 and AEBP1) (Fig. 8A), luciferase reporter assays were performed in HEK293 cells. Ssc-miR-671-5p mimics cotransfection with pmirGLO- DTX4 3’-UTR wt resulted in a significant decrease in luciferase activity, whereas the opposite effect was observed when mimics NC cotransfection with pmirGLO- DTX4 3’-UTR wt (Fig. 8B). Moreover, mutations in the predicted DTX4 3’-UTR binding sites abolished this effect. The suppression function of ssc-miR-671-5p on AEBP1 gene was consistent with DTX4 gene (Fig. 8C). Therefore, DTX4 and AEBP1 are target genes of ssc-miR-671-5p.
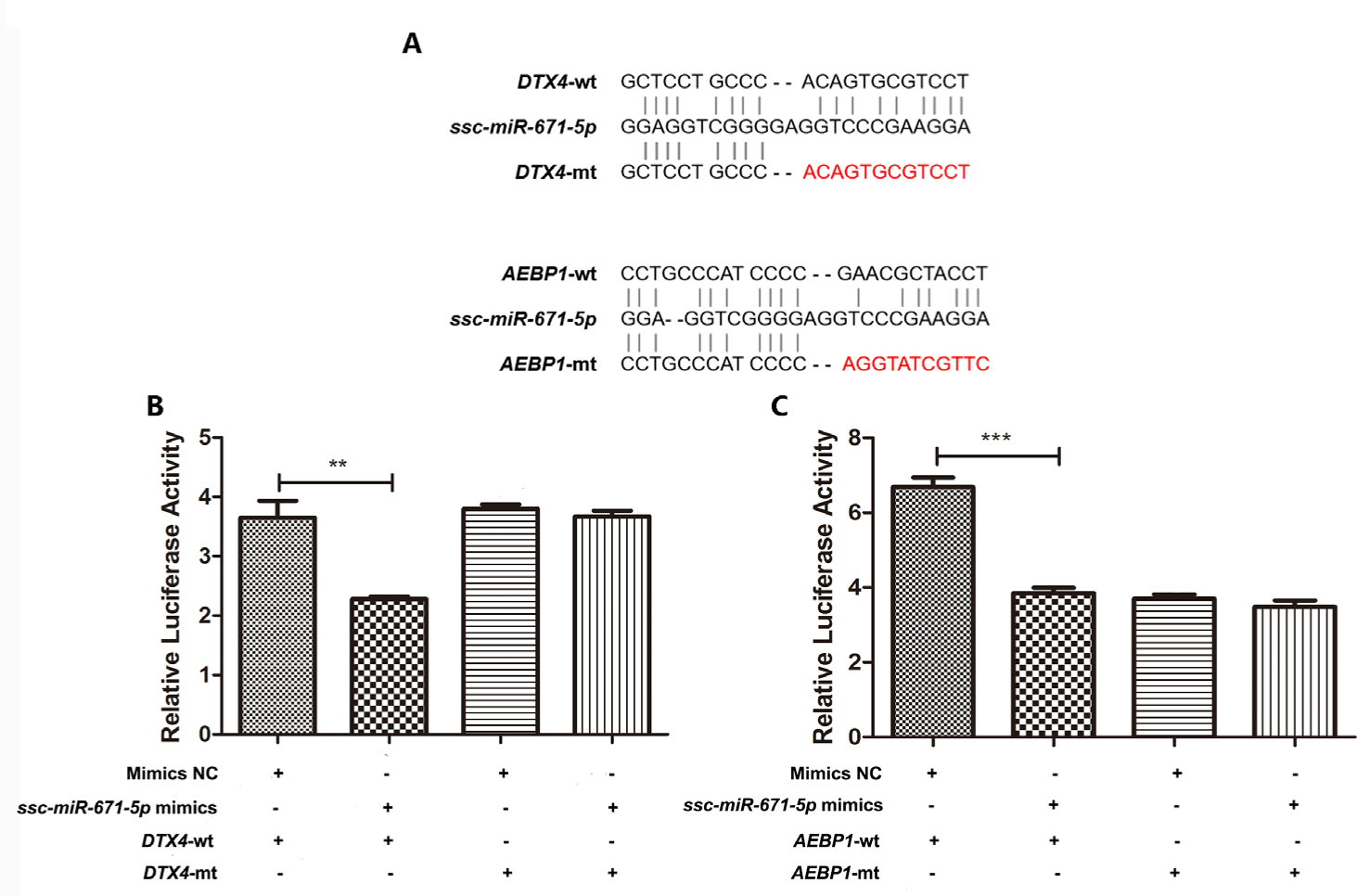
DISCUSSION
As a local pig breed in China, the Kele pig is famous for its resistance to diseases and for its high-quality pork, making it excellent breeding material. PRDC is a severe ailment in pig breeding in China. Our previous study reported that Kele piglets challenged with PRRSV and HPS showed changes in clinical symptoms, body temperature, histopathology, and transcriptome levels in the lung, inferring that reactive oxygen species (ROS) production and pulmonary fibrosis were affected in Kele piglets in response to PRRSV and HPS co-infection [12]. This study aimed to further explore the effects of PRRSV and HPS infection on the miRNA and lncRNA levels in Kele piglets, which improved our understanding of the immune response to these pathogens in the host. Based on the comparative analysis, 122 DElncRNAs and 20 DEmiRNAs were found in the PRRSV group. The number of DElncRNAs was greater than that reported by a previously published study, but the number of DEmiRNAs was less [20]. We inferred that the different pig species and pathogens are key factors influencing the amount of non-coding RNA identified in studies, which suggest that experiments with different breeds infected with the same pathogens could be taken in further studies to compare the immune responses of different breeds. We also found that more DElncRNAs and DEmiRNAs were identified in the co-infection group than these in the single infection groups, implying that multi-pathogens co-infection tends to stimulate more changes in non-coding RNAs, which may lead to more complex post-transcriptional immune regulation. Moreover, compared with protein-coding genes, lncRNAs in lung tissue had the same characteristics in different tissues and cells [20,35].
As an important regulatory factor of post-transcriptional regulation, miRNAs play an important role in many biological processes. There were eight DEmiRNAs shared by the three infected groups, among which ssc-miR-21-5p, ssc-miR-20b, ssc-miR-106a, ssc-miR-7-5p, and ssc-miR-21-3p were upregulated. The expression of ssc-miR-202-5p, novel_611, and novel_190 was downregulated. High expression of the shared DEmiRNA miR-21-5p can inhibit H2O2 (PTEN/AKT)-induced apoptosis of alveolar epithelial cells, thus improving acute lung injury induced by hyperoxia in rats [36], and it is also related to the epithelial–mesenchymal transition (EMT) in humans [37]. These studies showed that ssc-miR-21-5p was highly expressed in the lungs of pigs infected with pathogens, and the highest expression level in the co-infection group (log2 [fold change] = 1.7005) may be related to pulmonary fibrosis after acute lung injury. MiR-20b can induce polarization of M2 alveolar macrophages in mice. Macrophages present in alveoli and the mesenchyme are activated to form M2 alveolar macrophages, participating in the process of pulmonary fibrosis after lung tissue is continuously stimulated by antigen [38]. Ssc-miR-20b also had the highest expression in the co-infection group, indicating that both pathogens can lead to pulmonary fibrosis formation after continuous infection, and PRRSV and HPS co-infection promoted the process. Both miR-106a and miR-7-5p were found to be associated with human lung cancer [39,40]. Ssc-miR-21-3p, shown to promote PRRSV replication in MARC-145 cells in a previous study [41], was upregulated post-infection in the three treatment groups compared with the control. Ssc-miR-202-5p was downregulated about 5-fold in the PRRSV and PRRSV–HPS groups compared with the control group, and is related to EMT [42], apoptosis [43] and innate immunity [44]. In the PRRSV–HPS group, among 21 unique DEmiRNAs, novel_209, novel_494, ssc_miR-371-5p, and novel_207 were differentially expressed more than 2-fold compared with the single-infection groups. The overexpression of ssc_miR-371-5p may inhibit proliferation and promote apoptosis of mesangial cells by directly targeting HIF-1α in humans [45], suggesting that upregulation of ssc-miR-371-5p after infection might also induce apoptosis.
Increasingly more evidence shows that miRNAs can regulate expression of their targets through lncRNAs, thus affecting gene function [20,35,46,47]. Although many lncRNAs have been found, the potential role of lncRNAs in the pig respiratory system is far from understood. A large number of target genes of DEmiRNAs and DElncRNAs participating in host immune regulation were found in this research. In the PRRSV–HPS group, the CD163 molecule, having long been confirmed to be the receptor protein of PRRSV [48], was regulated by two DElncRNAs (LNC_008554 and LNC_017117), so we speculated that these two DElncRNAs may participate in the invasion process of PRRSV. MMPs and TIMPs were regulated by nine DElncRNAs and are related to pulmonary fibrosis [49], indicating that these nine DElncRNAs may be involved in the regulation of pulmonary fibrosis. Some studies have shown that C1QA [50], CD8B [51], and IL21R [52] are related to the classical complement pathway and immune response of the host. In our study, C1QA and CD8B were regulated by ssc-miR-671-5p, IL21R were regulated by three DElncRNAs (LNC_000282, LNC_015493, LNC_017117) and one DEmiRNA (ssc-miR-7137-3p), which showed that these DEmiRNAs and DElncRNAs may regulate the expression of genes.
We also found that there were many differentially expressed non-coding RNAs regulating immune-related biological processes and immune-related pathways. Particularly in the co-infection group, two special DEmiRNAs and 16 DElncRNAs were involved in regulating eight immune-related biological processes, such as complement activation, interaction with host, and B cell-mediated immunity. Compared with PRRSV or HPS infection alone, KEGG pathways in the PRRSV–HPS group were more complex and were mainly involved in the regulation of complement and coagulation cascades and the HIF-1 signaling pathway. Many lncRNAs and miRNAs reported to be related to the porcine respiratory system were also involved in host immune response [20], consistent with our results. Therefore, we suggested that these specific differentially expressed non-coding RNAs play critical roles in the innate immune and adaptive immune processes.
In the ceRNA network, we observed that ssc-miR-671-5p interacted with AEBP1, DTX4, SLC2A, FAM160A1, CAMK2A, and four DElncRNAs in the PRRSV–HPS group. Moreover, the results of the dual-luciferase reporter assays showed that the relative luciferase activities were significantly lower in cells transfected with the ssc-miR-671-5p mimics than in cells transfected with mimics NC in DTX4-wt and AEBP1-wt groups. This validated the role of ssc-miR-671-5p in regulating the expression of the DTX4 and AEBP1 genes. It was reported that silencing AEBP1 markedly suppressed the proliferation, migration, invasion, metastasis, and EMT of guanine cytosine (GC) cells [53,54]. However, overexpression of ssc-miR-671-5p led to the downregulation of AEBP1 expression in the present study, which suggests that ssc-miR-671-5p inhibits pulmonary fibrosis by regulating the expression of the AEBP1 gene. The published research showed that NLRP4-DTX4 mediated TBK1 degradation to promote virus infection, while the DTX4 gene knock-down eliminated TBK1 ubiquitination and degradation, and enhanced TBK1 and transcription factor IRF3 phosphorylation to achieve innate antiviral effects [55]. It is speculated that the expression of DTX4 is downregulated under the influence of ssc-miR-671-5p after persistent co-infection, and the body produces an antiviral response. Therefore, ssc-miR-671-5p may be a key promoter of the host’s inhibition of lung diseases and its antiviral mechanism, which deserves further study. Remarkably, novel_207 was the only downregulated miRNA in the ceRNA network, targeting the ADM5 gene. ADM5 may induce cell migration and invasion [56]. Therefore, the downregulation of novel_207 may involve cell migration and invasion by interacting with ADM5 after infection with PRRSV and HPS.
CONCLUSION
Differentially expressed lncRNAs and miRNAs have been identified between PRRSV and HPS single-infection and co-infection groups compared with the control group, the complex relationships between DEmiRNAs, DElncRNAs, and their target genes were analyzed in three infected groups. The ceRNA regulatory networks were constructed for the different groups. Two miRNAs (ssc-miR-671-5p and novel_207) were found that play important roles after PRRSV and HPS concurrent infection. Ssc-miR-671-5p is a regulator for DTX4 and AEBP1 genes, which was verified by dual-luciferase reporter assays. This explained the potential relationship between the two pathogenic infections of Chinese local pigs at the transcriptome level.