INTRODUCTION
The Hanwoo production system in Korea consists of three tiers, including the seed stock sector (bull selection sector), cow-calf operations, and feedlot sector [1]. The Korean government operates the bull selection program, which is called the National Genetic Evaluation Program, to select proven bulls to increase the genetic potential of the entire Hanwoo population [2]. The semen straws of selected bulls are distributed to the multiplier sector (reproduction of cows) to produce calves. The use of genetics to select bulls has gradually increased over the past 20–30 years and is remarkably successful [3]. However, no female genetic improvement program for carcass traits has been proposed. Korean farmers recognize the necessity of genetically improving the production and reproductive traits of cows to obtain higher profit margins. Compared with the bull selection breeding program, selecting females is more challenging because estimating the breeding value of a female is difficult due to a lack of information. Currently, the female estimated breeding value (EBV) can be determined for production and reproductive traits using the pedigree index (PI), bull (sire) pedigree, and best linear unbiased prediction method [4]. Pedigree data are traditionally applied to determine the EBV using phenotypes generated from half-sib, full-sib, and progeny testing [5]. However, pedigree information may be recorded incorrectly; for example, some individual identification tag numbers could be lost or incorrectly attached to livestock, leading to false pedigree information and inaccurate predictions [6]. Thus, determining an accurate EBV to select the female population during farm-level breeding operations is difficult. Farmers running small cow farms that produce calves wish to predict their cows’ EBV to obtain cows with better genetic potential; therefore, a more effective method with few errors is needed.
The disadvantages of the PI and pedigree-based best linear unbiased prediction (PBLUP) methods could be overcome by recent developments in genomic technology, which enable estimation of the genomic breeding value (GEBV) using DNA variants [7, 8]. In addition, the cost of these tests has been decreasing. Therefore, genomic information allows us to derive more accurate breeding values than previous methods [9].
The accuracy of the EBV can be affected by the genetic relatedness (genetic co-variance) between a large reference population and female candidates. The effective number of chromosome segments (Me) is used to estimate genetic relatedness between two populations [10]. Me is also defined as the variance of identity-by-descent among genetically related individuals [11]. The lower the Me value, the closer the genetic relationship between the reference and test population [12]. The accuracy of the GEBV increases if the genetic relatedness between the reference and test population is high.
This study aimed to compare the accuracy of three methods (PI, PBLUP, and the genomic best linear unbiased prediction [GBLUP]) for analyzing a reference population comprised of half-sib family data and a test population (481 cows), and for identifying factors affecting the accuracy of EBVs for replacement cows for selecting females.
MATERIALS AND METHODS
Using a reference population (n = 16,971) and female test population (n = 481), we compared the accuracy of EBVs of females estimated using different methods (Fig. 1). The reference population was comprised of 16,971 steers derived from half-sibs of 485 bulls genotyped with the Illumina bovine 50K chip (Illumina, San Diego, CA, USA). Fig. 2 describes the data collection process and shows the structure of the reference population. The phenotypes (carcass weight [CWT], eye muscle area [EMA], backfat thickness [BFT], and marbling score [MS]) for the reference population (n = 16,971) were collected from Korea Animal Products Quality Evaluation. Summary statistics of the reference phenotype are shown in Table 1. The mean and standard deviation of the CWT, EMA, BFT, and MS were 441.2 ± 50.88 kg, 95.81 ± 12.21 cm2, 14.26 ± 4.92 mm, and 5.95 ± 1.85, respectively. The data were normally distributed, as shown in Fig. 3. The pedigree information for the reference population (n = 16,971) consisted of three generations including 44,145 animals (Table 2). The female herd (n = 481) was one-quarter genetically related to validate their breeding value and there were 1,595 ancestors in the pedigree (Table 3). All the female herd sample cows were selected randomly from all over the country, and derived from 104 Korea proven (KPN) bulls, and those cows are not part of the reference population. Pedigree information for the test and validation animals was collected from the Korea Animal Improvement and Association ([KAIA] https://www.aiak.or.kr/).
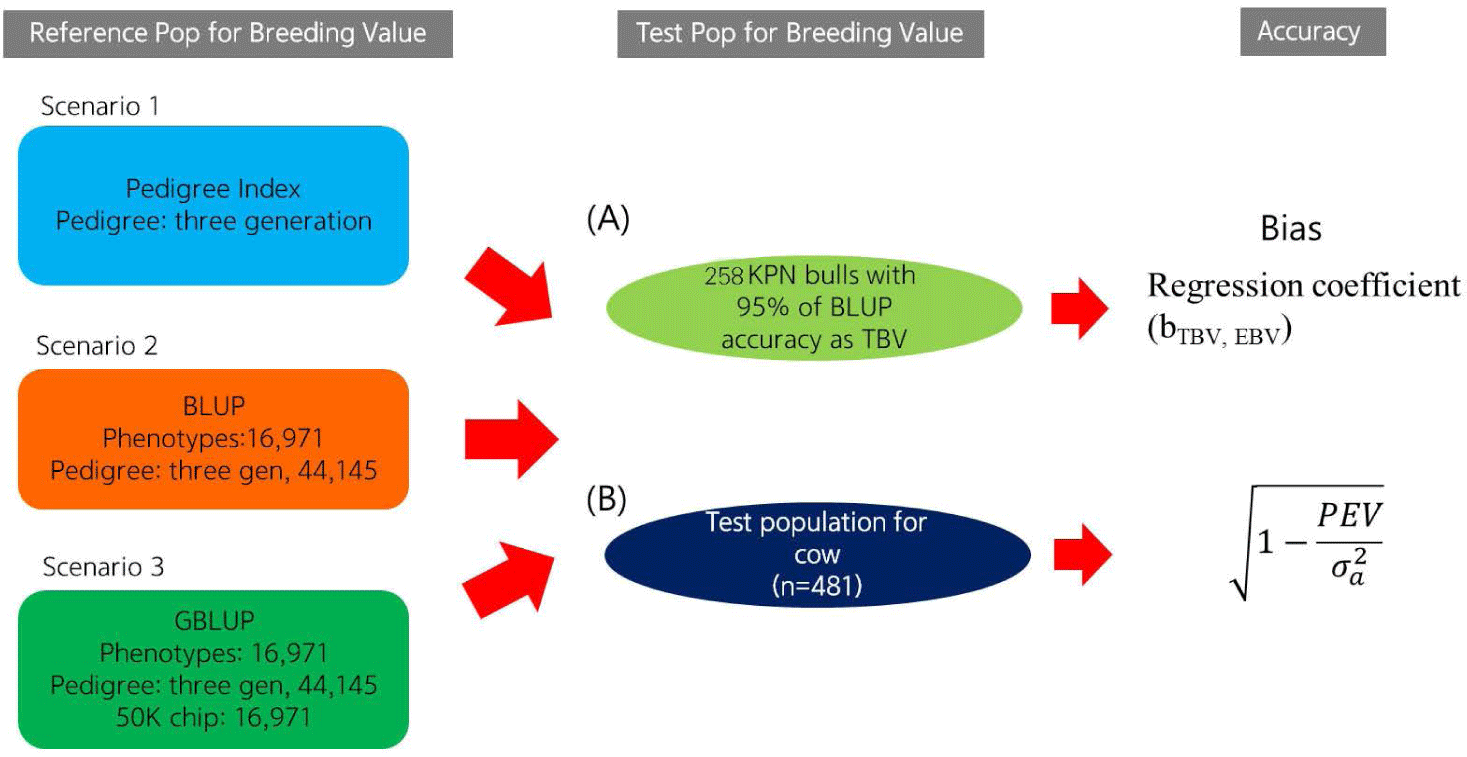
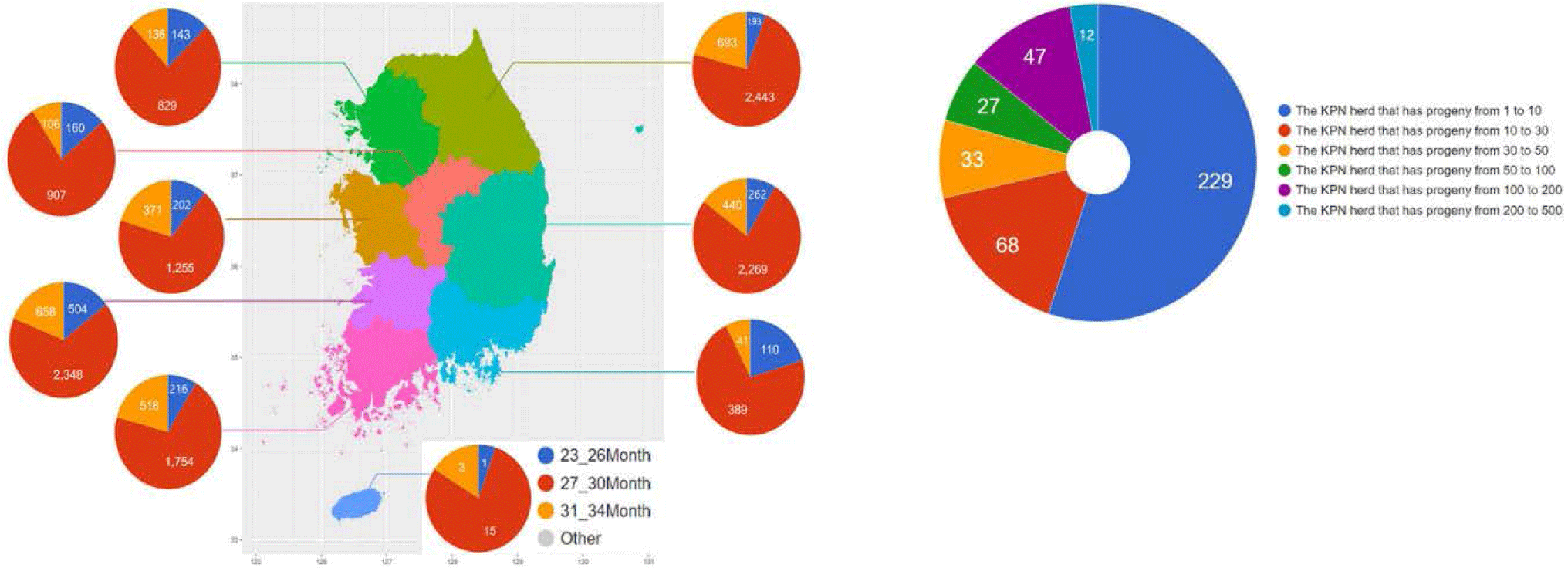
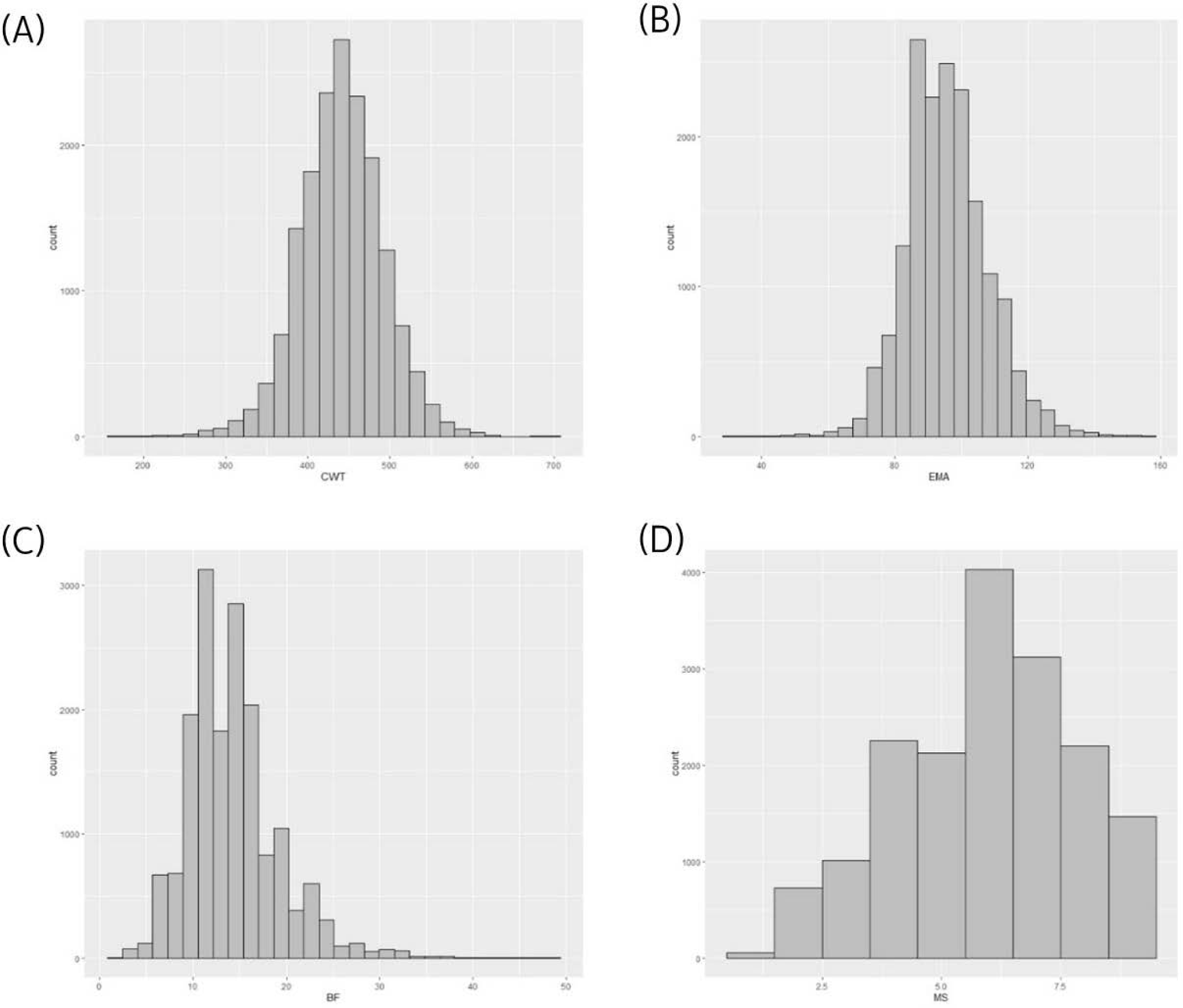
Traits | Mean | SD | Minmum | Maximum |
---|---|---|---|---|
CWT (kg) | 441.2 | 50.43 | 214.0 | 632.00 |
EMA (cm2) | 95.82 | 12.07 | 43.00 | 148.00 |
BFT (mm) | 14.2 | 4.77 | 1.00 | 35.00 |
MS | 5.95 | 1.85 | 1.00 | 9.00 |
Age | 30.26 | 1.91 | 21 | 40 |
The 481 cows were genotyped using the bovine 50K single nucleotide polymorphism (SNP) chip (Illumina). Quality control was performed while estimating the GEBVs of the 481 cows (test population) based on 16,971 commercial Hanwoo (reference population). Using Plink 1.9 software (http://pngu.mgh.harvard.edu/purcell/plink/), SNP markers with missing call rates > 0.1, minor allele frequency < 0.01, and a p-value of Hardy-Weinberg equilibrium < 0.0001 were removed [13]. A total of 40,635 SNP markers were used in this study.
In this study, we compared the accuracy of breeding values estimated using three different methods (PI, PBLUP, and GBLUP) for female replacement and management.
The National Agricultural Cooperative Federation and National Institute of Animal Science conducted performance and progeny testing to select KPN. The EBV accuracy for these bulls was 75%–80%. Based on this information, the PIs and EBVs of the test cows were estimated using a three-generation pedigree (sire, grandsire, and grand-grandsire). The equation was as follow [14]s:
A mixed model was used for PBLUP, including traits and considering the effects of environmental factors. The model was constructed using the BLUPF90 program [15,16] and has the following equation [17]:
where y is a vector of the phenotype information; X contains the design matrix of the observations for fixed effects; b is the vector of the fixed effects, including farm, birth year, and month, slaughter year and month, and age; Z is the design matrix-matching phenotype value and random effect values; u is the vector of the random effects with a normal distribution; e is the vector of residual error effects with a normal distribution is random variance, and I is the identity matrix. . A is the numeric relationship matrix (NRM) constructed based on the pedigree information.
GBLUP uses the genomic relationship matrix (GRM) based on SNP markers. The GRM is expressed as [18].
Then,
where M is the matrix for genes of individuals, m is the total number of SNP markers, and pi is the frequency at the i-th position in the SNP.
The general linear mixed model equation for GBLUP is:
where Z is the incidence matrix for the animal effect (a), X is the incidence matrix for the fixed effect (b), is residual variance, and a . Therefore, GBLUP is a BLUP that replaces NRM with G [GRM]).
This study investigated the accuracy of the EBVs according to prediction error variance and the correlation between the true breeding value (TBV) and EBV. To investigate the accuracy of the EBVs derived using the three methods, we selected those of the top 258 KPN bulls (i.e., bulls with EBVs predicted by the BLUP method with 95% accuracy) as the TBVs. The Pearson’s correlation coefficients between TBV and the EBVs for the 258 KPN bulls were considered to reflect the actual accuracy.
The accuracy of the EBVs for cows was calculated based on the prediction error variance (SE2) and additive genetic variance of the trait estimated using the BLUPF90 program [15]. The equations for the theoretical and PI EBV accuracy are as follows: [14]
The genetic relatedness between the reference and test data is an important factor affecting genomic prediction accuracy that varies depending on the data set. Several methods are available to calculate the relatedness of the reference and test populations. The number of effective chromosome segments (Me) is one of the most useful methods for calculating genetic relatedness. To determine the genetic relatedness between the reference and test populations, which affects the accuracy of the cow breeding value (EBV), we calculated Me using genomic information [19]. Various approaches can be used to estimate Me within a population. In this study, the following equation was used to estimate Me [20].
The genomic relationship between individuals was calculated as the covariance matrix (Gij) [21]. In the Gij, the variance of the covariance of the entire reference population was calculated for each individual and converted into a reciprocal number. If the variance between individual i and j was close to 0, the genetic relationship was considered weak [20]. The Gij for the genomic relationship was estimated from Me. In addition, we investigated the difference in prediction accuracy based on Me.
RESULTS AND DISCUSSION
The accuracy of the EBV is ordinarily determined by two methods. Empirical accuracy is reflected in the Pearson’s correlation between TBV and EBV [22] for the three different methods, and theoretical accuracy is calculated from the prediction error variance and additive genetic variance for individual test cows [23-25]. TBV could not be determined in this study due to a lack of information on the genes controlling the phenotype. Therefore, we regarded the EBVs of the top 258 KPN bulls as TBVs to compare the accuracy of breeding values among the three different methods (PI, PBLUP, and GBLUP). The EBVs were determined for the top 258 KPN bulls and the reference population (16,971 cattle) using the three methods. Fig. 4 shows that the accuracies of GEBV were 0.78, 0.54, 0.62, and 0.66 for CWT, EMA, BFT, and MS, respectively. The PBLUP accuracies were 0.63 and 0.56 for CWT and BFT, and 0.39 and 0.56 for EMA and MS, respectively. The PI showed accuracies of 0.35, 0.27, 0.22, and 0.3 for CWT, EMA, BF, and MS, respectively. The accuracy of the GBLUP method was about 12% higher than that of the PBLUP method using pedigree information (Fig. 4). Many studies have shown that the GBLUP method is superior to PBLUP, and Naserkheil et al. [26] reported that GBLUP (single- and multiple-trait GBLUP) was approximately 19% and 36% more accurate than conventional BLUP models (single- and multiple-trait GBLUP) for carcass traits in Hanwoo. Moreover, GBLUP has performed well in many studies, and the data of the present study showed similar trends [27,28].
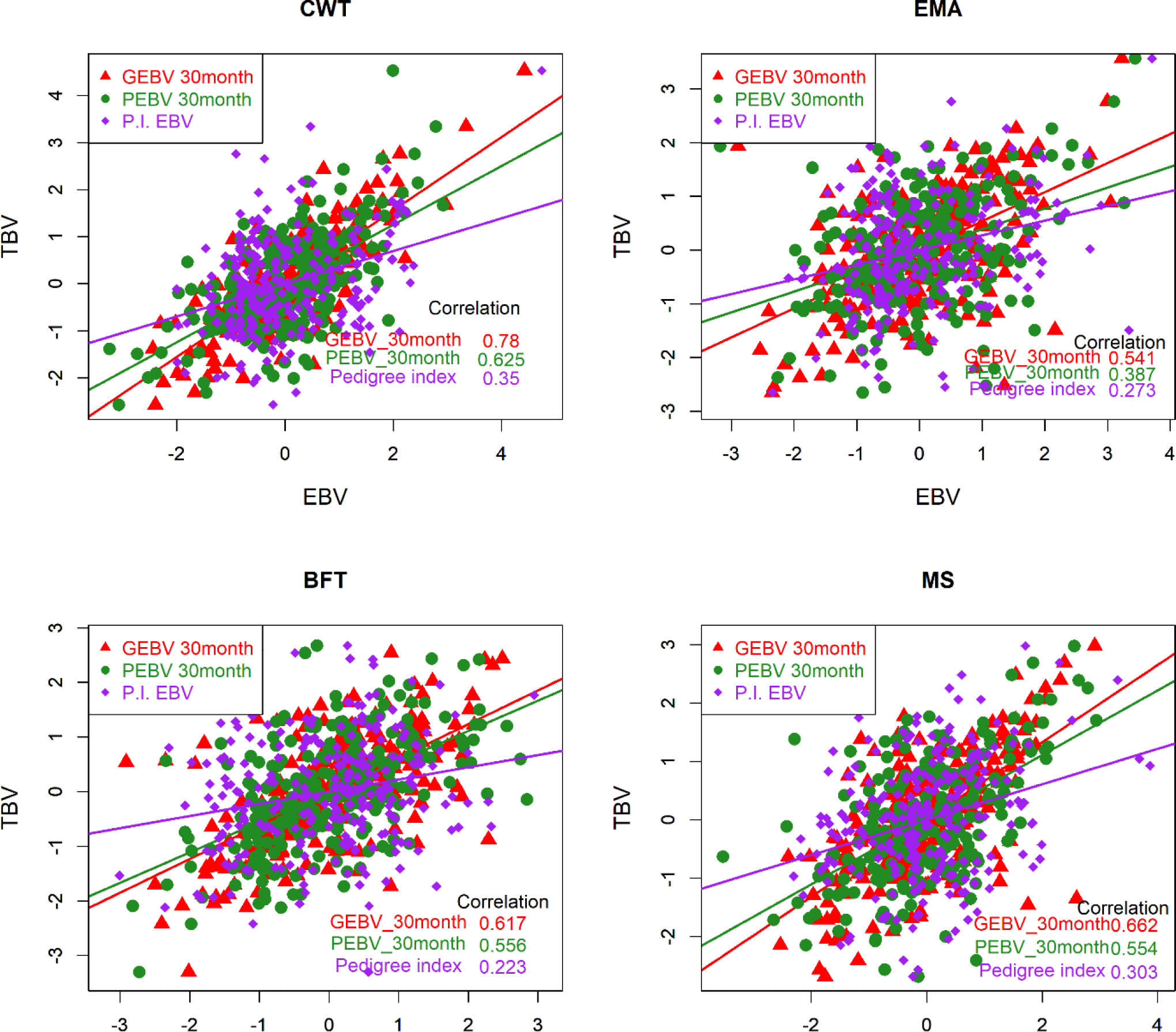
We investigated theoretical breeding value accuracy for individual test cows using prediction error variance. The breeding value of individual test cows was also estimated using the PI, PBLUP, and GBLUP methods. The reference population consisted of 416 KPN families in different age classes, and the number of progenies of each KPN half-sib family varied from 5 to 378 (Fig. 2). The accuracies of the GBLUP method were 0.634, 0.659, 0.619, and 0.627 for CWT, EMA, BF, and MS, respectively. The PBLUP accuracies were 0.434, 0.445, 0.435, and 0.431 for CWT, EMA, BF, and MS, and those for PI were 0.276, 0.294, 0.293, and 0.293, respectively (Fig. 5). The accuracy of the GEBV method was highest for all traits. The accuracies of the PBLUP EBV and PI EBV were about 0.2 and 0.35, which were lower than that of the GEBV. A difference of about 0.15 was detected between the accuracy of the PBLUP EBV and PI EBV methods.
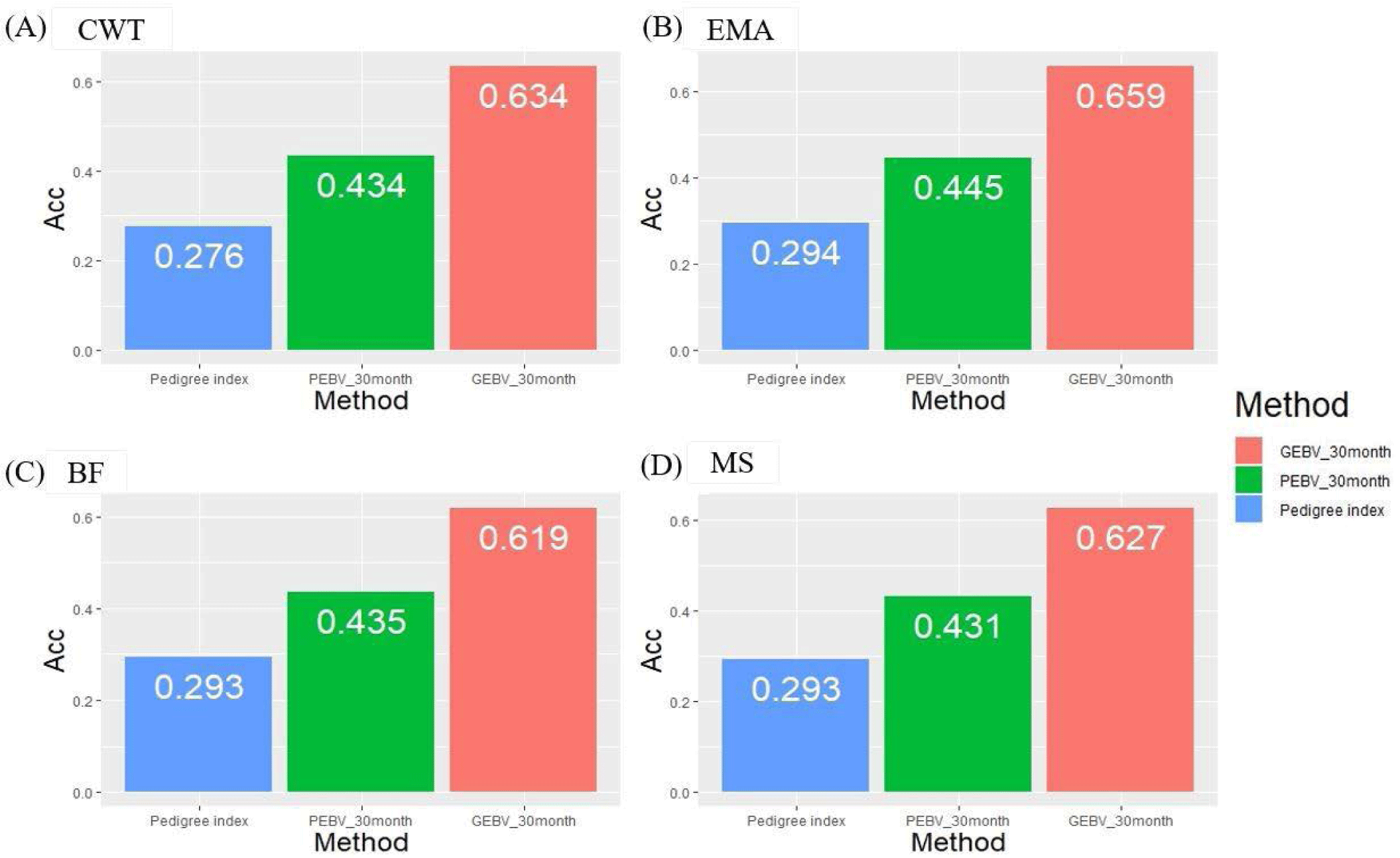
The finding that GBLUP outperformed PBLUP and PI could be explained by several factors, such as genetic covariance (genetic relatedness) between the reference and test populations [29]. In this study, a heatmap plot of the additive genetic relationships between the reference and test populations (cow) showed that, with the GRM, there was a stronger additive genetic relationship compared with pedigree-based NRM (Fig. 6). Clark et al. [29] reported that the accuracy of genomic selection depends on the strength of the relationships between the reference and test populations. If unrelated animals were estimated using the PBLUP method, accuracy would be close to zero. In contrast, the GEBV method showed high accuracy for animals that had no pedigree relationship with animals in the reference data set. The breeding values estimated using the GBLUP method were more accurate for closely related animals (~0.5) compared to those of the PBLUP and PI. Similar results were reported by Hayes et al. [30] and Habier et al. [31,32] for populations with a close relationship. Therefore, genetic relatedness is a major factor in the higher accuracy of the GBLUP compared to the PBLUP and PI seen in this study. A drawback of applying the PBLUP and PI methods to a half-sibling dataset (without individual phenotypes or progeny information) is that the accuracy of the EBV does not increase over 50% because of Mendelian sampling error [33]. This drawback cannot be solved at birth of the animal using the pedigree information [33]. Analysis of genetic gain depends on the accuracy and time taken to estimate the Mendelian sampling term. The GBLUP method is one way to control Mendelian sampling error because it provides useful information on alleles that originated from the parents. And both NRM and GRM consider an inbreeding coefficient, but GRM has more accurate inbreeding coefficient information (Fig. 7). Therefore, the GBLUP method produced more accurate breeding values than the PBLUP method in this study. The pedigree information included man-made error (affecting 10% of test animals), which caused the NRM to diverge from the actual genetic covariance. A simulation study by Nwogwugwu et al. [6] showed that a 20% pedigree information error rate led to a 5%–10% decrease in prediction accuracy.
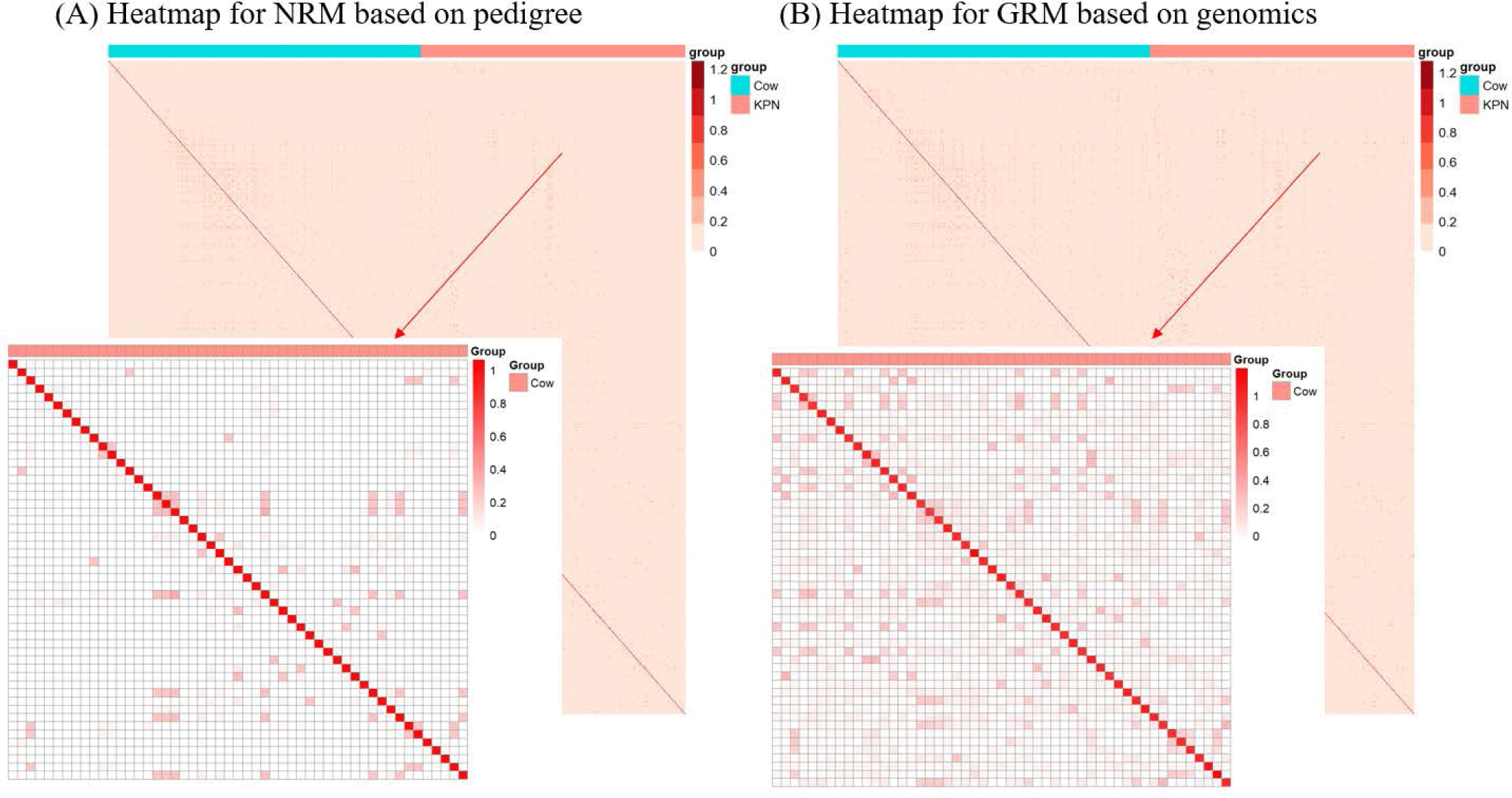
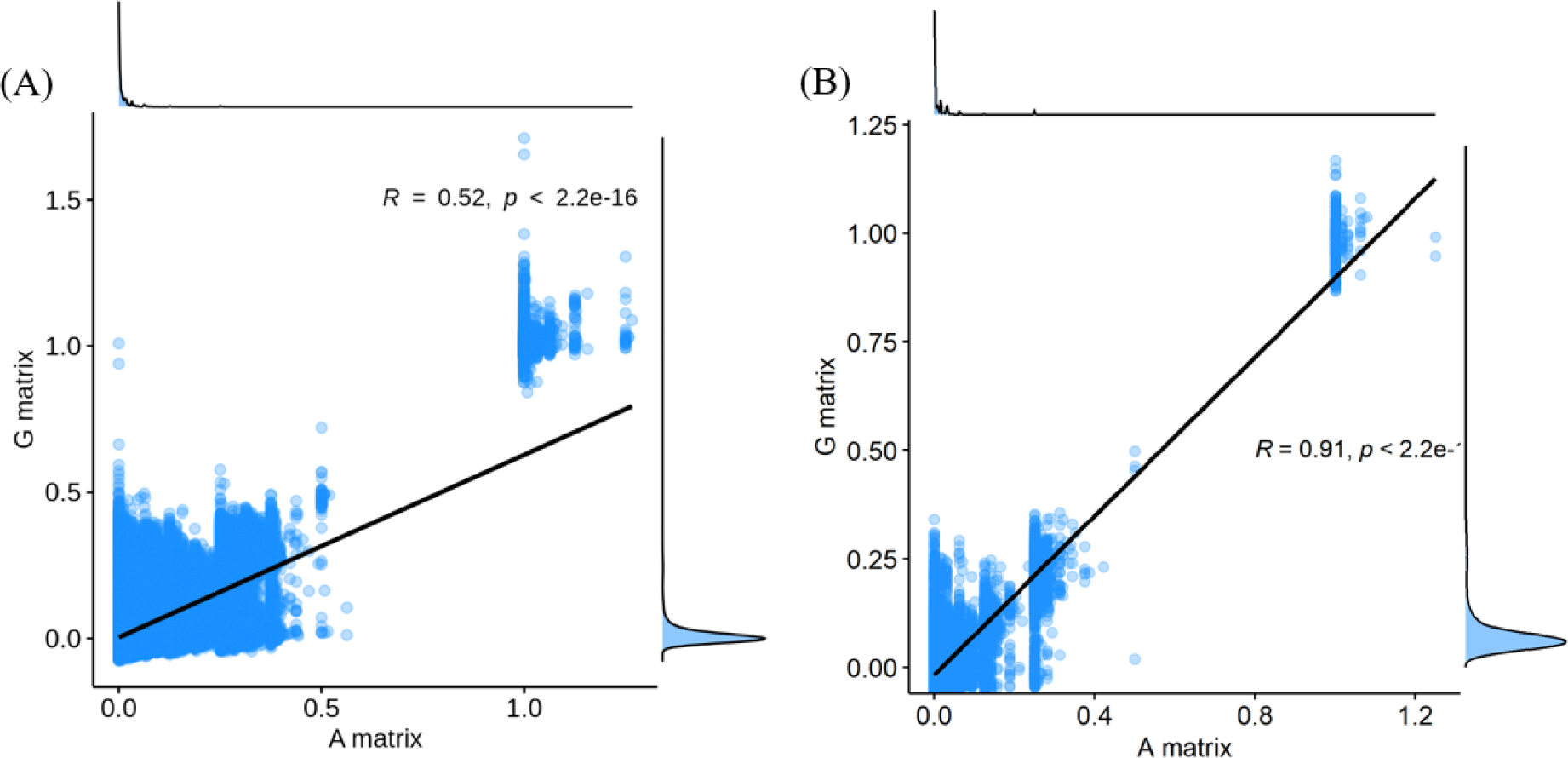
Genetic relatedness between reference and test populations is reflected in the Me and effective population size (Ne) [34], where Me represents the number of independent chromosomes arising during gametogenesis; the lower the Me value, the closer the relationship between the reference and test populations [19]. Fig. 8 shows the correlation between the Me values of the reference and test cow populations, and the accuracy of the EBVs for the 481 test cows. The correlations between the Me values and accuracy values for CW, EMA, MS, and BFT were −0.74, −0.75, −0.73, and −0.75, respectively. The reference population included 356 KPN half-sibs and the replacement cow population consisted of 104 KPN half-sibs; 60 KPNs were common between the two populations (Fig. 9A). Fig. 9B shows the Me values and accuracies of the EBVs of test cows with common KPN sires and different KPN ancestors. The Me value of the common KPN cows was 1,237.09 for all traits, and the accuracies were 0.64, 0.62, 0.63, and 0.66 for CWT, EMA, BF, and MS, respectively. In contrast, the Me value of the counterparts was 2,063.22 for all traits, and the accuracies were 0.6, 0.59, 0.6, and 0.63 for CWT, EMA, BF, and MS, respectively. It is logical to suggest that the closer genetic relationship between the reference and test animal (low Me) led to more accurate EBV predictions. A set of simulation data was generated based on parameters with an Ne of 1,000, 51 quantitative trait loci (QTLs), and heritability of 0.3 to estimate the accuracy of the GBLUP method [35-37]. This simulation study showed that lower Me (NQTL) values led to more accurate GBLUP and BayesB results. Me is defined as the variance of identity by descent among individuals for genetic relatedness.
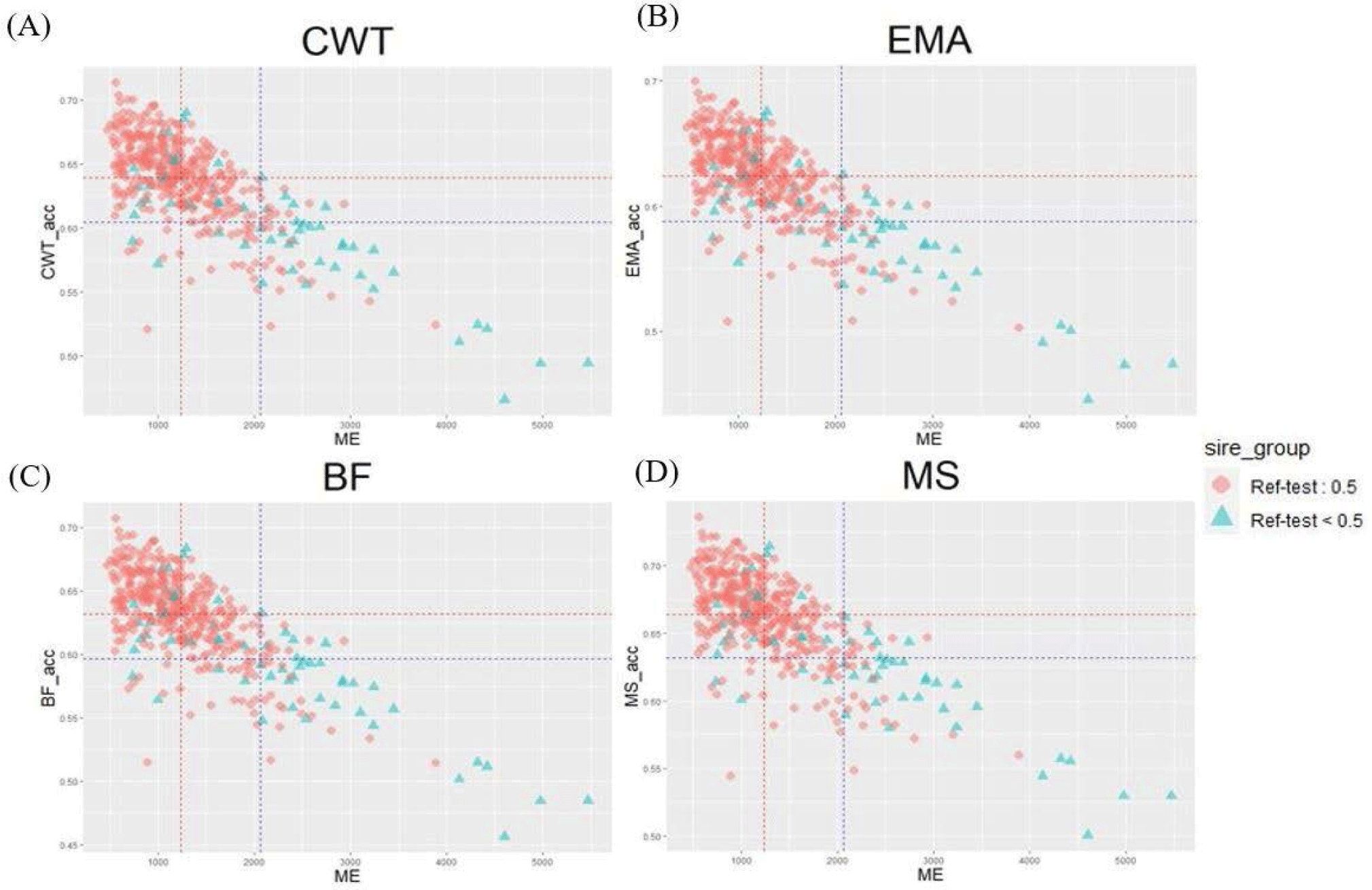
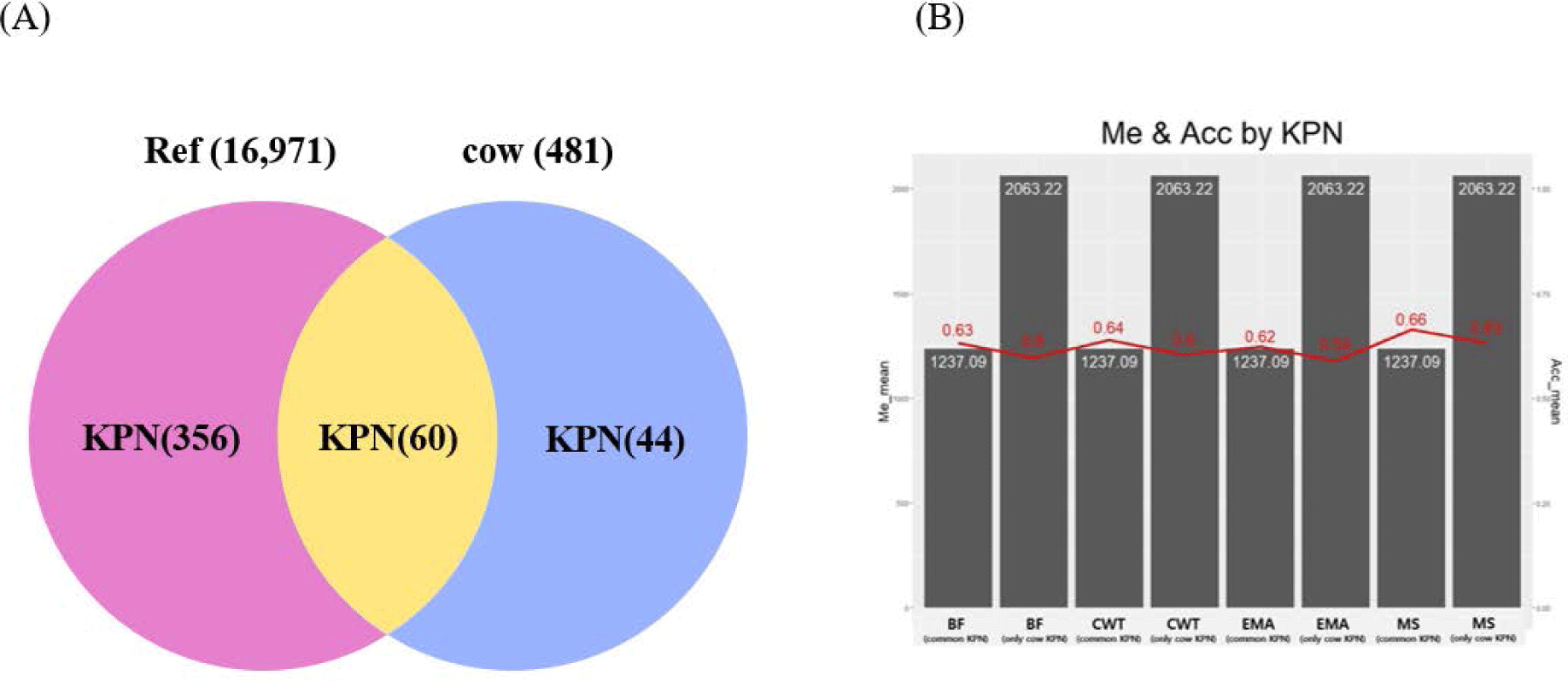
In conclusion, we compared genomic prediction accuracy among three methods (PI, PBLUP, and GBLUP), using 258 KPN bulls with 95% accurate TBVs derived from BLUP. GBLUP outperformed PBLUP and the PI in terms of the accuracy of the EBVs. The accuracy of the GBLUP method was about 12% higher than that of the PBLUP method when using pedigree information. The accuracy of the GEBV method was higher than that of the PBLUP and PI for all traits, as reflected in cow EBVs estimated using collateral information (half-sib dataset). We propose that genomic data can be used to obtain random inheritance information about alleles from parents; this explains the high prediction accuracy of the GBLUP method used in this study. Moreover, genetic relatedness between the reference and test (cow) populations affected the accuracy of the EBVs estimated from the additive genetic relationship and Me. A difference in Me values between common KPN and cow KPN of about 820 increased the accuracy of the GBLUP by about 3.25%. Measuring the Me value of animals could be useful for determining the accuracy of cow EBVs estimated using GBLUP.