INTRODUCTION
Global affluence and population growth are driving food demand and the amount of protein needed to survive [1]. As poultry is accepted as a good protein source for humans, poultry production needs to be strengthened in many countries, which will increase the number of high-density poultry farms [1,2]. However, there are concerns that intensive production systems may be more susceptible to disease outbreaks, as the potential for disease introduction and transmission is determined by factors such as the number and density of animals, the number and type of contact between herds, and sanitary measures [3–5]. Rapid detection and diagnosis is paramount to avoid an increased risk of spread of poultry infectious diseases in these production systems [1,6].
Fowl typhoid (FT) is a poultry systemic disease that causes significant economic losses in many countries through increased mortality and morbidity [7–10]. The disease is caused by Salmonella enterica serovar Gallinarum, which is distributed worldwide, and is usually characterized by reduced feed intake and egg productivity, mainly anemia, leucocytosis and haemorrhages, and death within 4 days [10,11]. FT has been frequently observed in broilers and causes severe mortality in broiler chicks [10]. A study has shown that FT had a mortality rate of 10.5% in broiler chicks in parts of Haryana between July 1987 and June 1990 [12]. In particular, in Korea, FT was the most serious bacterial disease in poultry in 1992, and it occurred in a total of 983 farms from 2000 to 2008, causing economic damage [13]. Clinical signs found in such FT-infected broilers include decreased growth rate, loss of appetite and dullness, decreased activity, increased thirst, droopy wings and typical loose greenish-yellow diarrhea [10,14,15]
Automation plays an important role in the poultry industry worldwide [16]. Automated systems operating through remote monitoring and control systems must store large amounts of data obtained through monitoring and enable easy access and real-time decision-making based on the recorded data [17]. Such systems can reduce the cost and labor required for livestock production and improve livestock production and quality. In addition, it would make it possible to identify abnormal behavior and symptoms in livestock and prevent disease outbreaks, thereby minimizing economic damage to farms[1,16,18]. Recently, automated systems have been developed based on poultry behavior or sounds, such as walking, standing, running, resting, sneezing, abnormal vocalizations, feeding sounds, and sound vibration frequencies [1,19–21]. Among them, sound-based make it difficult to accurately identify infections among thousands of poultry on commercial farms [6], but behavioral diagnosis has revealed markedly different postures and mobility between healthy and infected poultry [22]. According to several studies, the behavioral clinical symptoms of diseases in poultry include dyspnea, coughing, decreased feed and water intake, unstable gait, and sudden death, and in particular, decreased activity was reported.
Therefore, in this study, we tried to evaluate the potential of a behavioral characteristic observation method for detecting sick chickens. FT was induced by injection of S. Gallinarum into broilers, and the observed behavioral characteristic was non-movement behavior, and the applicability of developing a remote management system that can detect sick chickens early by monitoring the duration and frequency of the behavior was confirmed.
MATERIALS AND METHODS
The experimental protocol was reviewed and approved by the Institutional Animal Care and Welfare Committee of the National Institute of Animal Science, Rural Development Administration, Republic of Korea (2018-297).
S. Gallinarum A18-GCVP-014 (Jeonbuk National University, Jeonju, Republic of Korea; Genbank accession number: ON416860) stored at −70°C were streaked on MacConkey medium and grown for 18 h at 37°C. Ten colonies were picked and inoculated into 30 ml of Luria-Bertani (LB) broth and incubated for 13–15 hours. After the inoculated strain was grown to optical density (OD)590 = 1.22 (~1 × 109 Colony forming unit (CFU) /mL), the strain was diluted 10-fold to 1 × 108 CFU/ mL by phosphate-buffered saline (PBS).
A total of forty 1-day-old Ross 308 males were used to observe the symptoms and behavior of sick chickens. The rearing facility was equipped with a monitoring isolator for broilers (Jeonbuk National University, Jeonju, Republic of Korea) that was used to continuously observe and record the behavior of the birds (Fig. 1). The isolator was manufactured to accommodate 40 chicks (2 m × 2 m) and was equipped with two feeders and eight nipple drinkers. In addition, to keep the chicks warm, the floor was covered with 5 cm thick rice hull, and a heat supply was installed to control the temperature. The experiment was conducted for 4 weeks, from September 18 to October 15, 2019. The temperature was set to 33°C at the age of 1 day, and was subsequently lowered approximately 2°C–3°C every week and finally maintained at approximately 21°C. The diet was a uniform industrial diet without antibacterial properties and was provided ad libitum together with unlimited drinking water. Continuous photo surveillance was maintained throughout the experiment period.
The pathogen used in this study was S.Gallinarum, which is the causative agent of FT. Pathogen inoculation was performed at 14 days of age, a period when chickens are most susceptible to FT [23,24]. The route of infection was oral administration using 0.5 mL of the culture with 1.9 × 108 CFU/mL, a concentration corresponding to lethal dose (LD)20 (lethal dose for 20% mortality) [25,26].
The top-view camera used was a fixed Internet Protocol (IP) camera (AXIS M3066-V Network Camera, Axis Communications, Lund, Sweden) installed ~1.7 m above the isolator. The camera’s horizontal field of view (HFOV) was set to 132° and the vertical field of view (VFOV) to 96°, pointing downwards to capture a top view of the inside of the isolator. The video images were captured with a resolution of 1280×960 pixels in the moving picture experts group-4 (MPEG-4) format at 30 fps for 24 h every day. The recorded video was transmitted and saved to a network attached storage server (NAS; Synology, New Taipei City, Taiwan), powered over Ethernet (PoE; Advantech, Taipei, Taiwan) connection. An individual marking method using different colors was employed to observe the behavioral patterns of each broiler. Forty markers were made by combining black, yellow, green, and blue colors, with a width of 6 cm and a length of 4 cm (Fig. 2).
The frequency of daily clinical symptom observation and the number of chickens that died were investigated to determine whether the infection was caused by S. Gallinarum inoculation. Clinical symptoms caused by diseases, such as respiratory distress, drowsiness, diarrhea, weakness, feather characteristics, and death were recorded [9]. The symptom was observed twice daily (09:00, 20:00) for two weeks after inoculation and changes were recorded.
Gross lesions were assessed on chickens that died during the observation period and chickens that survived the experiment. Chickens that died during the experiment were immediately observed. The degree of enlargement of the liver, spleen, and heart or necrotic lesions was evaluated, and scores of 0, 1, 2, or 3 were assigned, respectively. A score of 0 indicated no lesions, and a higher score indicated more severe lesions [27] (Fig. 3).
To determine whether the observed lesions were caused by inoculation with S. Gallinarum, a part of the liver was collected. The liver slices obtained were diluted with buffered peptone water (BPW; Difco, Franklin Lakes, NJ, USA) at a ratio of 1:9. Thereafter, Rappaport-Vassiliadis (RV; Sigma-Aldrich, St. Louis, MO, USA) broth was used for the specific selection and culture of Salmonella species, and the diluted sample and RV broth were mixed to obtain a ratio of 1:99. The culture medium mixed with RV broth was incubated at 40°C for 24 h, followed by streaking on xylose lysine tergitol 4 (XLT4; Difco) agar plates. The XLT4 agar plate was cultured in an incubator at 37°C for 20 h, and 16s rRNA sequencing was subsequently performed to identify the isolated strain [28].
To determine the serotype of the FT causing strain, serum was collected from all living individuals at the end of the experiment. The collected serum was first screened using a slide agglutination test. Subsequently, the serotype was confirmed by a micro-aggregation (MA) test using the antigen of S. Gallinarum.
The serum plate agglutination (SPA) test was used to confirm S. Gallinarum infection in the broilers. For this, 20 μL of chicken sera and 20 μL of crystal violet-stained antigen were placed on a glass slide and mixed appropriately with a toothpick. A reaction that appeared within 2 min was confirmed, and if positive, granules were formed slowly within 2 min. If negative, granules did not form within 2 min, which means that there was no antibody against S. Gallinarum infection [29].
The MA test was conducted on broiler serum samples tested positive using the SPA test. The titers of anti-S. Gallinarum IgG in serum samples were measured using an enzyme-linked immunosorbent assay (ELISA), as described previously, with some modifications [30]. Briefly, 96-well plates were coated, washed, and blocked as follows: plates were coated overnight at 4°C with 100 ng of S. Typhimurium ultrasonic antigen in 100 μL of coating buffer (0.016 M Na2CO3, 0.034 M NaHCO3 [pH 9.6]), followed by removal of the coating solution, washing twice with 350 μL of washing buffer (PBS + 0.05% Tween 20), and blocking for 2 h at 37°C with 200 μL of blocking buffer (washing buffer + 2% bovine serum albumin [BSA]). Serum samples (100 μL) were diluted in dilution buffer (PBS + 2% BSA) at 1:400 and incubated in the wells for 1 h at 37°C, with 100 μL of dilution buffer used as a negative control. Then, 100 μL of 1:8,000 horseradish peroxidase (HRP) rabbit anti-mouse-IgG gamma conjugate and HRP-conjugated goat anti-chicken IgG (H+L) (KPL, Gaithersburg, MD, USA) or 1:10,000 HRP-conjugated goat anti-chicken IgA antibody and HRP-conjugated goat anti-chicken IgM antibody (Bethyl Laboratories, Montgomery, TX, USA) in dilution buffer was added to the wells and incubated for 1 h at 37°C. Subsequently, 100 μL of the 3,3’,5,5’-Tetramethylbenzidine (TMB) substrate was transferred to the wells and allowed to react for 1 h at room temperature. Subsequently, 50 μL of stop solution (4.5 N H2SO4) was added to terminate the reaction. The OD 450 was measured immediately using an ELISA plate reader (PerkinElmer, Waltham, MA, USA). All samples were independently run in triplicate, and logarithmic antibody titers were calculated for further analysis.
The image data analysis was performed through images of broilers inoculated with FT pathogens using a top-view camera. In order to detect a broiler, the chicken area must be accurately recognized, so a model was developed that finds the chicken area in the image through Convolutional Neural Networks (CNN), a deep learning system (Fig. 4). After that, continuous observation was made through an IP camera to which a system for identifying broilers was applied, and objects were displayed in various colors depending on the time they did not move. The non-moving object was determined to have not moved when more than 95% of the total pixels of each object were maintained by comparing the images continuously taken by the IP camera with the previous photographed images. Also, if the appearance of the broiler detected in the next image did not match the previous image, the generated mark was removed and set up in a way that it was observed again.
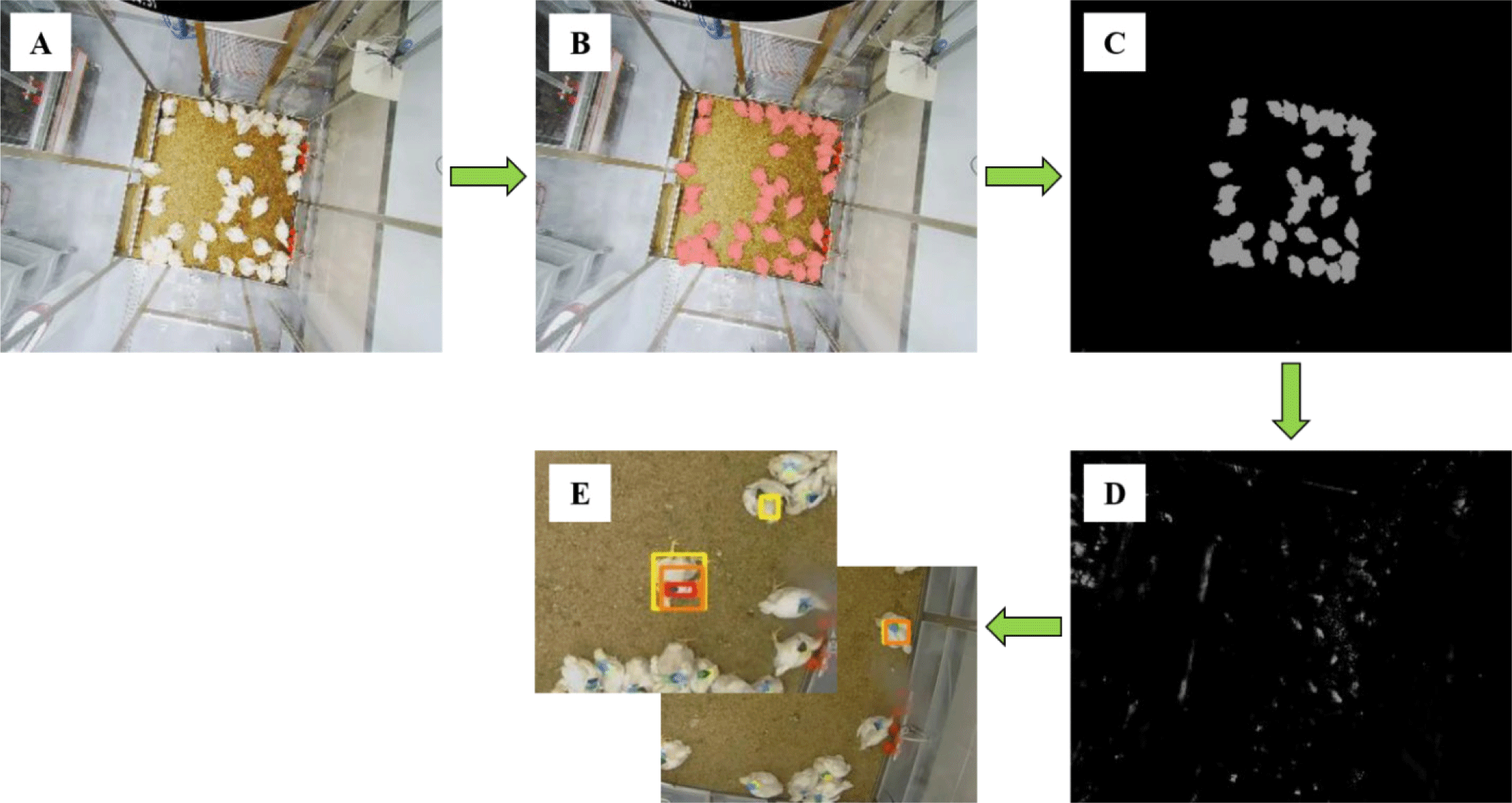
Fig. 5 shows the overall algorithm for the creation and removal of markers by observing non-movement behavior duration and movement of broilers using top-view camera. It was observed for 14 days after S. Gallinarum inoculation, it was set to display the following three colors according to the duration from the moment when non-movement of each individual was detected: yellow color, not moving for 5 minutes; orange color, not moving for 15 minutes; red color, not moving for more than 30 minutes. Based on the results detected by the IP camera, the detection accuracy of infected chickens through non-movement behavior was analyzed.
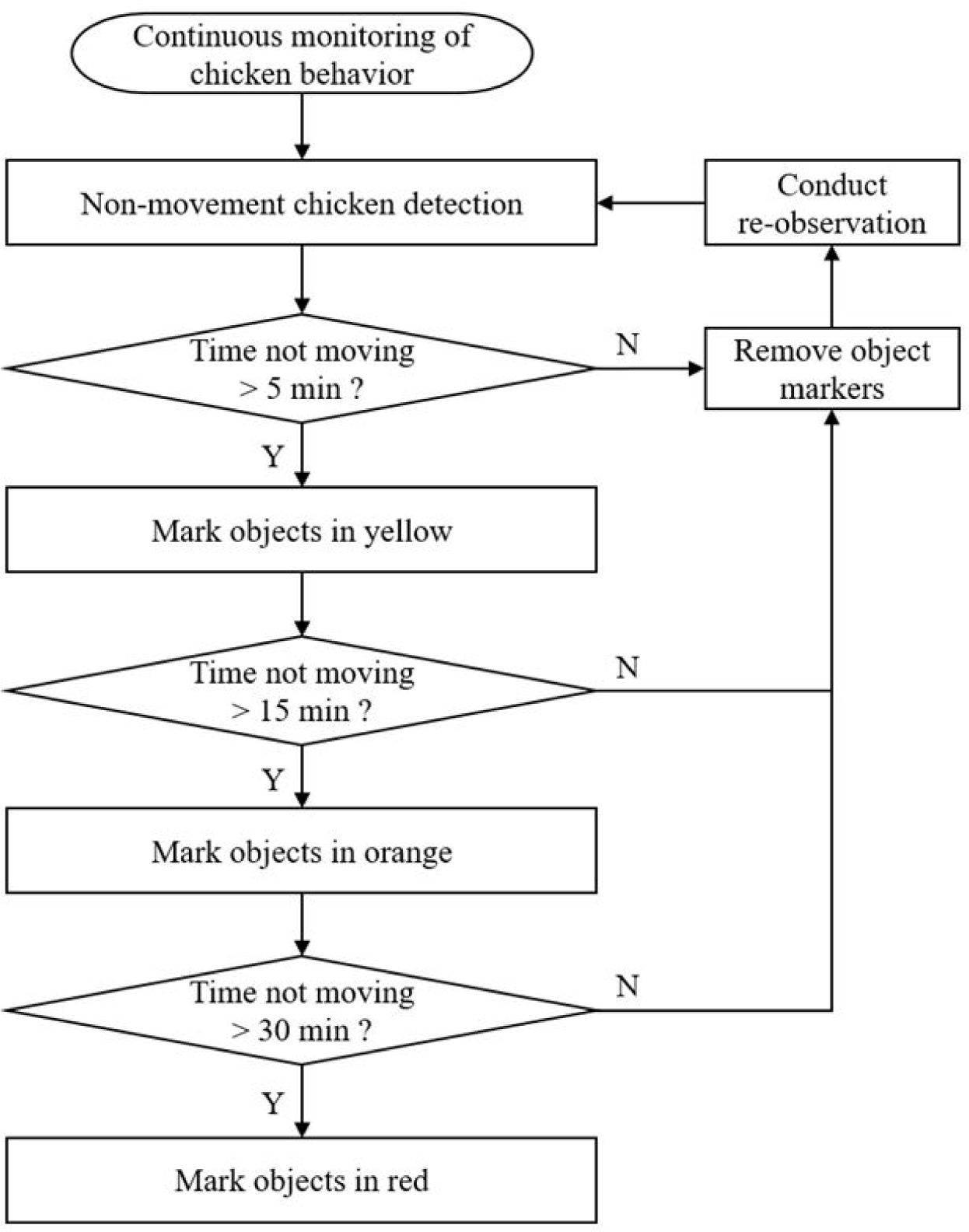
RESULTS
The daily mortality and clinical symptoms observed following S. Gallinarum inoculation are presented in Fig. 6. Mortality due to infection started to appear from the 6th day after inoculation, and the highest number of five deaths per day was observed on the 7th day. Fourteen chickens died during the experimental period. The first clinical symptoms were observed on the 4th day after inoculation, and the highest number was observed on the 10th day (72.4%). From the 11th day onwards, the number of symptomatic individuals decreased and the birds showed signs of recovery.
The gross lesion scores of broilers are presented in Table 1. The average liver lesion score was 2.00 for dead chickens and 1.04 for live chickens, and for the spleen the lesion score was found to be 1.21 for dead chickens and 0.23 for live chickens. From this, it was judged that the chickens that died during the experiment had suffered from multiple issues, including more severe damage to the liver and spleen due to S. Gallinarum infection. However, the heart was shown to be severely affected, even in live chickens. Overall, dead chickens scored 3.57 for liver, spleen, and heart lesions, whereas live chickens scored 2.38.
Table 2 shows the results of re-isolation of S. Gallinarum from the livers of dead and live broilers. S. Gallinarum was isolated from the livers of all dead broilers. However, in live broilers, the pathogen was isolated in only 23 of 26 isolates. Of the three broilers in which no pathogen was detected, two had liver lesions and one was asymptomatic. Overall, pathogen re-isolated from the liver showed a detection rate of 92.5% based on 37 detections out of 40 broilers.
Table 3 shows the results of the serological analysis of broilers that survived after two weeks of experimental monitoring. When SPA analysis was performed, the serum of seven out of 26 broilers showed agglutination with S. Gallinarum antigen. Broiler serum samples that tested positive in the SPA test were analyzed with the MA test. All tested sera showed agglutination reactions in 96-well microplates, and two samples showed high antibody titers in ELISA analysis.
Table 4 shows the detection results of dead chickens through the non-movement behavior detection algorithm, and was operated normally from the 7th day due to a malfunction of the camera. As a result, the IP camera detected 10 of the 14 dead chickens in advance, and 2 of the 4 chickens that could not be detected due to a problem with the IP camera. Therefore, the detection of non-movement behavior chickens showed an accuracy of approximately 83% by pre-detecting 10 out of 12 dead chickens (excluding 2 chickens due to technical problems). For live chickens, the IP camera detected 12 out of 26 chickens (Data not shown). Overall, IP camera detection by the algorithm pre-detected dead chickens with relatively high accuracy.
DISCUSSION
The purpose of this study was to develop a technology capable of the early detection of infected chickens by inducing FT to observe non-movement behavioral characteristics of disease induced broilers. FT, caused by S. Gallinarum, remains an economically important avian septic disease in many parts of the world [31]. The FT-infected herds exhibit abnormal behavior and symptoms, including high morbidity and mortality, with birds exhibiting moderate to severe depression, low feed intake, and diarrhea [32]. Mortality rates range from 10% to 80%, affecting birds of all ages but mainly young chickens 2–3 weeks of age [24]. When forty-two 6-day-old specific pathogen free (SPF) chickens were inoculated with S. Gallinarum and observed for 7 weeks, 20 chickens died, and the total morbidity and mortality were 75.6% [33]. In another study, when 15 4-week-old Brown Nick chickens were infected with S. Gallinarum, eleven chickens died within 2 weeks, resulting in a 73.6% mortality rate [34]. S. Gallinarum infection in chickens results in gray-white necrotic lesions in the liver and spleen [35], and significant enlargement of the liver and spleen has been reported compared to uninfected chickens [34]. In addition, the lesions appear as a bronze dis-coloration of the liver, and several secondary lymphoid follicles appear in the spleen. In the case of the heart, there are necrotic foci, multiple white nodules with distorted shapes, and severe degeneration or fragmentation of myocardial muscle fibers is observed [36].
After inoculating the 1-day-old Hy-line layers with S. Gallinarum, the infection was confirmed by re-isolation. When re-isolation was conducted from the liver and spleen 1 week after inoculation, the pathogen was isolated from all tested chickens, whereas 2 weeks after inoculation some re-isolation attempts were unsuccessful. In particular, the number of S. Gallinarum present in the liver and spleen gradually decreased over time, and chickens showed a tendency to recover [37]. In a similar study, 6-week-old commercial chickens were inoculated with S. Gallinarum and observed for three weeks. Testing of the liver, spleen, and cecum for S. Gallinarum confirmed that the level of infection gradually decreased to 75% after 1 week, 50% after 2 weeks, and 0% after 3 weeks [38]. In our study, the detection rate of S. Gallinarum in the livers of dead chickens was 100%, suggesting that the chickens died due to infection with S. Gallinarum and the occurrence of FT. In addition, the result of re-isolation from live chickens after the end of the experiment revealed that the concentration of the pathogen gradually decreased over time and the chickens recovered, as in other studies.
In this study, when the SPA test was performed on live chickens, seven out of 26 chickens tested positive and most chickens did not show agglutination reactions. In general, the SPA test, which can be used to detect Salmonellae or Mycoplasma gallisepticum, is a very simple and sensitive method, but is suitable for detecting pathogen antibodies within 10 days of infection with the pathogen [39]. When the SPA test was performed on 279 chicken sera infected with S. Gallinarum and an agglutination reaction was observed, 125 samples showed a positive reaction, showing a detection rate of 44.8% [29]. In another study, 555 samples were collected from 30 poultry farms to determine whether they were infected with Salmonella. Using the SPA method, 38 samples (7%) showed a positive reaction, but in the analysis using fecal leukocytes, 82 samples (14.8%) were positive. In other words, a comprehensive investigation and diagnosis based on multiple analyses, rather than diagnosing infection through serum analysis alone, is necessary [40].
Recently, many studies have been conducted on the detection of chickens suffering from stress-inducing environments or diseases by monitoring specific behavior [41,42]. When chicken movement and drinking time were directly monitored using time-lapse video and deep learning algorithms at various temperature and humidity indices (THI), it provided a 98% chicken detection and tracking accuracy, and there was a moderate correlation between water intake time and THI [42]. In one study, 2D posture shape descriptors (circle variance, elongation, convexity, complexity, and eccentricity) and mobility features (walking speed) were analyzed for early detection of chickens infected with the Newcastle disease virus. Consequently, chickens were detected with high accuracy, and the proposed system contributed to the development of an automatic broiler monitoring system capable of early warning and prediction [22]. In addition, when monitoring the skeletal angle and posture of 6-week-old broilers infected with avian influenza virus H5N2, high or low accuracy was obtained according to each characteristic, but an accuracy of approximately 99% was obtained when all characteristics were considered [43].
It has been investigated that there are many types of behaviors in poultry rearing, such as sitting, lying, standing, feeding, drinking, walking, and preening [44–47]. Among them, the sitting behavior is a state in which the poultry’s ventral part and the fibula and tibia of the leg are in contact with the floor, and it is a behavior that occurs frequently in broilers and laying hens [47–49]. This behavior is similar to that observed in our study, and in the case of broilers, it is affected by the rearing density and environment, and the duration time and frequency increase as the body weight increases [50–53]. In particular, the time and frequency of sedentary behavior increased under various stress conditions (increased density, high temperature environment, air concentration in the facility, harmful substances in feed, etc.) [54–58]. However, the results of the investigation on the sitting behavior or non-movement behavior during disease outbreaks in broilers are not known, so further research is required on the behavioral observation time and observation method for more efficient detection.
In this study, when 14-day-old broilers were infected with S. Gallinarum, clinical symptoms were observed on the 4th day and dead chickens on the 6th day after inoculation. When gross lesions of various organs were examined, the dead chickens displayed more severe organ damage than the live chickens, and the pathogens were re-isolated from the livers of dead chickens. When looking at the overall results including serological tests results, the infection and FT progression occurred normally in the conducted study. IP camera detection through the algorithm detected dead chickens in advance with an accuracy of 83%, and some live chickens were also detected. In other words, it seems that the detection of sick chickens by behavioral observation can be detected in advance with high accuracy. However, the detection of sick chickens through the non-movement behavior has a disadvantage in that the sensitivity is still low, so that the object cannot be detected more quickly. In future studies, it is necessary to improve the detection criteria, and complex clinical symptom detection studies are required to increase the sensitivity and accuracy.
CONCLUSION
This study aimed to lay the foundation for the development of early detection technology using non-movement behavior observation for detecting sick chickens in order to improve the management of poultry farms. After inoculation with S. Gallinarum in broilers, most chickens suffered damage to several organs due to infection, and the presence of infection was confirmed by serum agglutination analysis. Many infected chickens showed clinical signs and non-movement behavior was observed. The detection of sick chickens using IP cameras pre-detected dead chickens with high accuracy. The detection technology developed based on the results of this study is expected to be of great help in the remote management of poultry farms.