INTRODUCTION
Some abnormal/extreme climate events are becoming more extreme in East Asia, depending on the season, due to global warming and rapid climate change [1]. It has been reported that abnormal climate events causes great damage to agricultural industry and crops [2,3]. In particular, climate events are important for forage crops that can be provided with high quality feed for livestock. Winter forage crops are vulnerable to abnormal climate events during winter, which is significant for survival. Therefore, on the Korean Peninsula, which belongs to the temperate climate zone and has four distinct seasons, the representative abnormal climate events which are fatal and prone to happen to winter crops are as follows: First, low-temperature events, which can occur after seeding in autumn, impede the growth and development of winter crops, making it difficult for them to obtain the energy needed for wintering [4]. According to the report on the climate of the Korean Peninsula [5], the damage caused by low-temperatures in autumn is expected to decrease gradually as global warming increases summer temperatures and extends the summer period. However, low-temperature event can still be fatal due to the increase in frequency and intensity of late monsoon rain (Kaul-Changma) and typhoon. Second, considering the characteristics of Italian ryegrass (Lolium multiflorum Lam., IRG), which has weak cold resistance, there is no doubt that the severe cold in winter is the most critical factor despite its good growth and development in autumn [6]. According to Min et al. [1], greenhouse warming has been assessed to lead to an increase in warm extremes and a decrease in cold extremes, but recent warming associated with Arctic sea ice-melting has tended to bring a cold snap to East Asia in winter. Therefore, the severe cold from warming is likely to become more deadly to IRG. Finally, if the spring drought persists for a long period, it causes a shortage of water resources following the winter dry season that damages the agricultural industry [7]. According to Kim et al. [8], there was a bimodal pattern of frequency between the short and long term for spring drought, which indicates the polarization of drought variation. Hence, as the dry season increases, spring drought will gradually emerge as an important abnormal climate event in the future. For abnormal/extreme climate events, the 90th percentile of temperature [9] and standard deviation [10,11] was mainly used as a standard. Although defining abnormal climate/weather by only considering temperature and precipitation was simple and efficient, the combined effects of several climate variables tend to be ignored. Furthermore, the selection by standard deviation with different multiples, such as one-fold, 1.5-fold, and two-fold, makes it difficult to handle consistent abnormal climate conditions. In this study, not only were temperature and precipitation considered to quantify the abnormal climate events, but also wind speed, humidity, and sunshine duration.
IRG has been generally cultivated in the southern inland and coastal regions of the Korean Peninsula, but this has gradually expanded from the southern to the central regions, and it is now cultivated in most regions except for the northern and central mountainous regions [12]. In general, IRG is vulnerable to the dry and cold conditions of the Korean winter due to lower cold and dry tolerance compared to other winter crops [13]. IRG is cultivated popularly in rotation system with rice, a major food crop, which is helpful to income of farmer in Korea. Furthermore, IRG is important winter forage crop in terms of feed value, such as feed nutritive and preference, on the southern and central regions of Korea [14]. Unfortunately, studies related to the effects of climate change and climate events on IRG have been seldom carried out in Korea, compared to the improvement of varieties and the development of cultivation techniques. Therefore, in this study, the impact of abnormal climate events on the IRG production was considered.
For studies on abnormal/extreme climate events on crop production, it is necessary to accumulate and analyze spatiotemporal climate information recorded in several places over a long period. Historical climate data has been used to assess climatic and soil factors on forage crops with various spatiotemporal characteristics. Climate information collected from the Korea Meteorological Administration (KMA) has been used for various purposes in the Korean agricultural field [15,16]. As part of a complex natural eco-system, the information on climate and soil factors was used to generate the structure of causality contains their direct and/or indirect pathways for forage crops, such as IRG [17,18], forage barley [19] and forage rye [20]. Climate data were also used to identify the trends in yields and climatic factors as a form of climate change research in Korea [21–23]. The drawback of performing this study using historical climate information was that it could not reflect abnormal climate conditions because only normal climates conditions because only normal climate conditions were taken into account.
Therefore, this study aimed to assess the impact of seasonal abnormal climate events on IRG production considering various climate variables. Besides this, production trends by year were checked in consideration of significant abnormal climate events in different regions.
MATERIALS AND METHODS
The IRG metadata (n = 1,107) including location, year, cultivar, seeding and harvesting dates, height and dry matter yield (DMY, kg/ha) were collected from reports of the National Agricultural Cooperative Federation and the Rural Development Administration of Korea from 1988 to 2013 (26 years). In general, abnormal/extreme climates in some regions may be normal in other regions; thus, it is necessary to classify the regions. According to Ko et al. [24], the southern Korean Peninsula was divided into five regions by temperature distribution: northern inland, central inland, southern inland, coastal, and mountainous regions. In this study, the central inland, southern inland and coastal regions were selected because the northern inland and mountainous regions were not suitable for IRG cultivation [18]. Suwon (latitude: 37° 25′ N, longitude: 126° 98′ E, n = 321), Jeonju (latitude: 35° 84′ N, longitude: 127° 12′ E, n = 107) and Jeju (latitude: 33° 51′ N, longitude: 126° 53′ E, n = 135) were selected as representative locations for the central inland, southern inland and southern coastal regions, respectively. These locations are similar to the region classification of weather forecasts based on administrative district (KMA). The cultivar mainly consisted of ‘Hwasan 101’ and ‘Kowinearly’, bred for improved winter survival cold tolerance in the central inland regions, while the southern inland and coastal regions consisted of experiments on various cultivars (Greenfarm and 41 other cultivars).
Based on the location, the raw meteorological data contains daily mean temperature, wind speed, relative humidity, precipitation amount, snowfall amount and sunshine duration were collected by open-application programming interface from the weather information system of KMA (1988–2013). To quantify the climate events for IRG, the season was divided into autumn, winter and next spring based on the earliest seeding date (03/September) and the latest harvesting date (12/June). In Korea, IRG is generally sown between late September and early October and harvested in late May [25]. Thus, growing periods were divided into autumn (September to November), winter (December to February), and next spring (March to May), respectively. To quantify abnormal climate events, the variables were temperature (°C), relative humidity (RH, %), precipitation amount (PA, mm), snowfall amount (SA, cm), sunshine duration (SD, hr) and wind speed (WS, m/s). Where, the temperature was: accumulated temperature (AT, °C) over 5°C in autumn and next spring, and mean temperature (MT, °C) in winter. Where, the lower limitation of AT, 5°C (41°F) was the base temperature of growing degree days for IRG [26].
In general, abnormal climate can be defined by various criteria, including the type, intensity and frequency of climate variables. In this study, abnormal and normal climate scenarios for autumn, winter and next spring were categorized by seasonal climate variables using statistical analysis. Here, a category that satisfies climatic conditions favorable for survival, growth and development for the high IRG yield was defined as a normal climate scenario. Meanwhile, climatic characteristics unfavorable to the survival, growth and development of IRG at a specific season were reflected to the abnormal climate scenarios.
In this study, various climate variables were used to generate the abnormal climate events as a season. In general, temperature and precipitation are critical for most abnormal/extreme climate events [9], and wind, humidity and sunshine are also important factors for crops. Unfortunately, since all these variables are highly related to each other, setting events independently with a single variable might be effective, but could not accurately reflect climate conditions. Therefore, in order to generate the seasonal climate events (low temperature in autumn, severe cold in winter and drought in next spring) for aim to compare with normal condition, principal component (PC) analysis was carried out with climate variables as follows [27]:
where Zq are climatic PC scores, Xp are climate variables, Λ is parameter of climate variable. p and q are the number of variable and climatic PC, respectively. These PC scores were used to characterize each climate scenarios in this study.
To consider various climate variables, distinct climatic characteristics were extracted via principal PC analysis with an eigenvalue over 1, and Euclidean-distance cluster analysis was used to classify abnormal and normal climate conditions by applying consistent criteria [27]. The year was classified by climatic PC scores using cluster analysis with Ward’s method; then, both were placed simultaneously to generate the climate scenario in scatter plots. Based on the year associated with the classified climate events, the DMY of IRG was compared between the events by t-test with a 5% significant level.
The PC analysis, cluster analysis, and t-test were performed by SPSS 24.0 (IBM, Armonk, NY, USA).
RESULTS
For monthly climate variables, the temperature was remarkable between regions (Table 1). In January, the coldest month in Korea, MT only exceeded 5°C in the southern coastal region, while in the central inland and south inland regions, it recorded −2.30 and −0.20, respectively. This indicates that there may be no difficulty with overwintering in the south coastal region despite the low cold tolerance of IRG [28]. Hence, it was hypothesized that severe winter cold was fatal to the DMY of IRG in inland regions rather than coastal regions. After the Korean monsoon season, the PA trend rapidly decreased until winter, regardless of region. In the central inland and southern inland regions, the March PA (mm) was lowest during spring, with 44.20 and 55.60 respectively. On the other hand, the March PA in southern coastal regions tended to be high, at 91.70 mm. This implies that spring drought would be serious in inland regions. WS was generally high in the southern coastal regions, and its fluctuations were somewhat stable except during spring, regardless of region. SA was recorded in the inland regions until March, but in the southern coastal regions, it was concentrated in winter. These regional and seasonal climate variables were used to quantify climate events such as autumn low-temperature, severe winter cold and spring drought.
For the regional and seasonal climate variables, two significant PCs were generated (p < 0.05), except for the southern coastal region in autumn (Table 2). The climatic characteristics of PCs were similar in both inland regions, regardless of the season: In autumn, PC 1 was associated with positive RH, positive PA and negative SD, respectively, and PC 2 with positive AT. In winter, PC 1 and PC 2 were strongly related to RH and WS, positive MT and negative SA, respectively. For spring, PC 1 and PC 2 were related to positive RH and PA, positive WS and negative SD, respectively; however, AT, PA, and SD did not noticeably contribute to only one PC. In the southern coastal regions, only PC 1 was strongly related to negative MT and positive WS in the winter. Finally, PC 1 and PC 2 were associated with positive RH, positive PA and negative SD, negative AT and positive WS in the spring, respectively.
Based on the climatic PCs, the year and climate variables were plotted together to classify abnormal climate events from normal (Figs. 1, 2, and 3). In the central inland regions (Fig. 1), the autumn normal and low-temperature events could be divided into the second quadrant close to SD of PC 1 and AT of PC 2, and a fourth quadrant close to RH and PA of PC 1 and far from AT of PC 2, respectively. For winter, normal and severe cold events were placed in the first quadrant, where RH of PC 1 and MT of PC 2 were located, and the third quadrant, where WS of PC 1 and SA of PC 2 were located, respectively. For spring, normal and drought events were distributed on a right quadrant where RH and PA of PC 1 were mainly arranged, and a left quadrant where WS, SD, and AT of PC 2 were arranged, respectively. The characteristics of climatic PCs of the southern inland region were similar to those of the central inland region (Fig. 2). However, in winter, normal and severe cold events were located in the second and fourth quadrants, respectively; because the sign of climate variables in PC 1 was opposite between central and southern inland regions. In the southern coastal regions (Fig. 3), autumnal climate events were not classified as normal and low-temperature events. In the case of winter, it was difficult to distinguish severe cold from normal because of the ambiguous position of climate variables, although PCs were statistically distinct. The spring droughts were prominent in the third quadrant related to high AT, high SD and low PA.
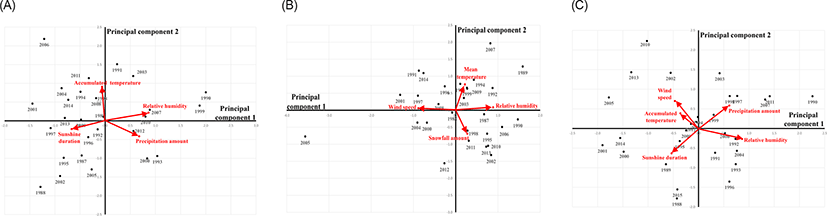
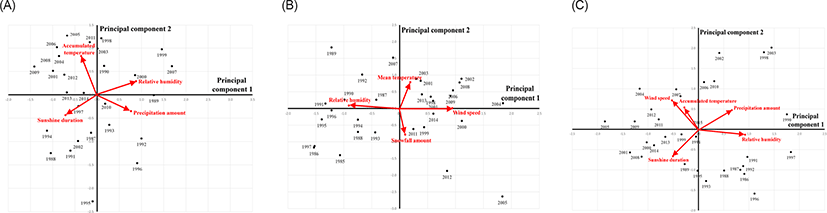
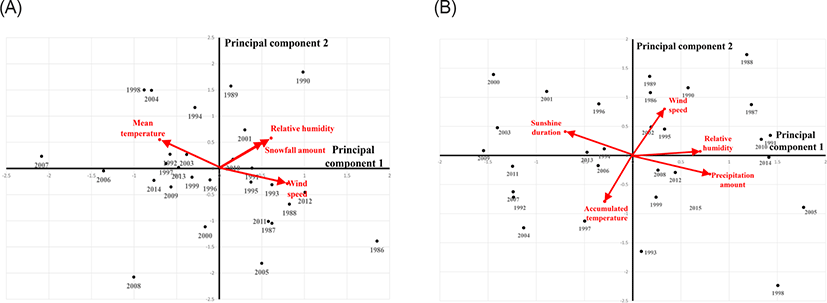
Years were also classified, based on the position of climate events. Years where the classification was not clear for each position were divided using individual cluster analysis based on the major climate variable related to PC 1 and PC 2. For example of the central inland region, 1988, 2002 and 1995, located on the third quadrant in autumn, were classified as low-temperature events using cluster analysis taking into consideration the PA of PC 1 and AT of PC 2. After classifying the year into each climate event, the impact of the climate event on IRG production was assessed.
To assess the impact of abnormal climate events on IRG production, DMY was compared by t-test (Table 3). In the central inland, the difference in DMY was greater in order of severe winter cold (2,620 kg/ha), spring drought (2,048 kg/ha) and autumn low-temperature (1,452 kg/ha). In the southern inland region, the only severe winter cold reduced DMY by 4,127 kg/ha (p < 0.05). It was not expected that the damage was greater in the southern inland region than in the central inland region, where the MT was lower. In the southern coastal region, the low-temperature event was not outside of the normal range, and the severe winter cold event was not effective (p = 0.61). The difference in DMY was 2,061 kg/ha between normal and spring drought (p < 0.05).
The impacts of significant abnormal climate events on DMY of IRG were described by identifying differences in climate variables between normal and abnormal climate events as shown in Tables 4, 5, and 6. In the central inland regions (Table 4), the PA of the autumn low-temperature event was twice as high as the PA of the normal event (p < 0.05). In winter, the differences in RH, WS, and MT were clear between severe cold and normal events (p < 0.05). Finally, between normal and spring drought, the differences in RH and PA were noticeable (p < 0.05). In the southern inland regions (Table 5), only the severe winter cold event was effective, and the differences in MT, RH and SA were important between winter severe cold and normal events (p < 0.05). In the southern coastal regions (Table 6), for the significant spring drought event, the effective climate variables were AT, PA, and SD (p < 0.05). It was concluded that the difference in these climate variables led to the difference in the DMY of IRG between normal and abnormal climate events.
The DMY trends of IRG by year with the happening of significant abnormal climate events were shown in Fig. 4, where the climatic characteristics were shown with heat-maps of PC scores to explain the intensity of abnormal climate events. First, in the case of the autumn low-temperature event in the central inland region (Fig. 4A), since 2000, the colors of PC 1 and 2 had tended to change to dark (low) and light (high), respectively. This indicates that the tendency of the low-temperature event was gradually weakening. The severe winter cold events in 1988, 1993, 1996, 1997, 2000, and 2005 were noticeable based on PC 1 related to WS, but PC 2 related to MT has been higher since 2000. Finally, for the spring drought, there were no specific patterns in PC 1, but the color of PC 2 was lighter since 2000. In the southern inland region (Fig. 4B), the high PC 1 in 2005 and low PC 2 in 2005, 2011, and 2012 were clear, indicating that the severe winter cold event based on WS and SA was serious. For the spring drought in the southern coastal region (Fig. 4C), the high PC 2 with low PC 1 stood out since 2005, which indicates low PA and strong WS. In these heat maps, the DMY fluctuation tended to change rapidly with the greater gap of color by year.
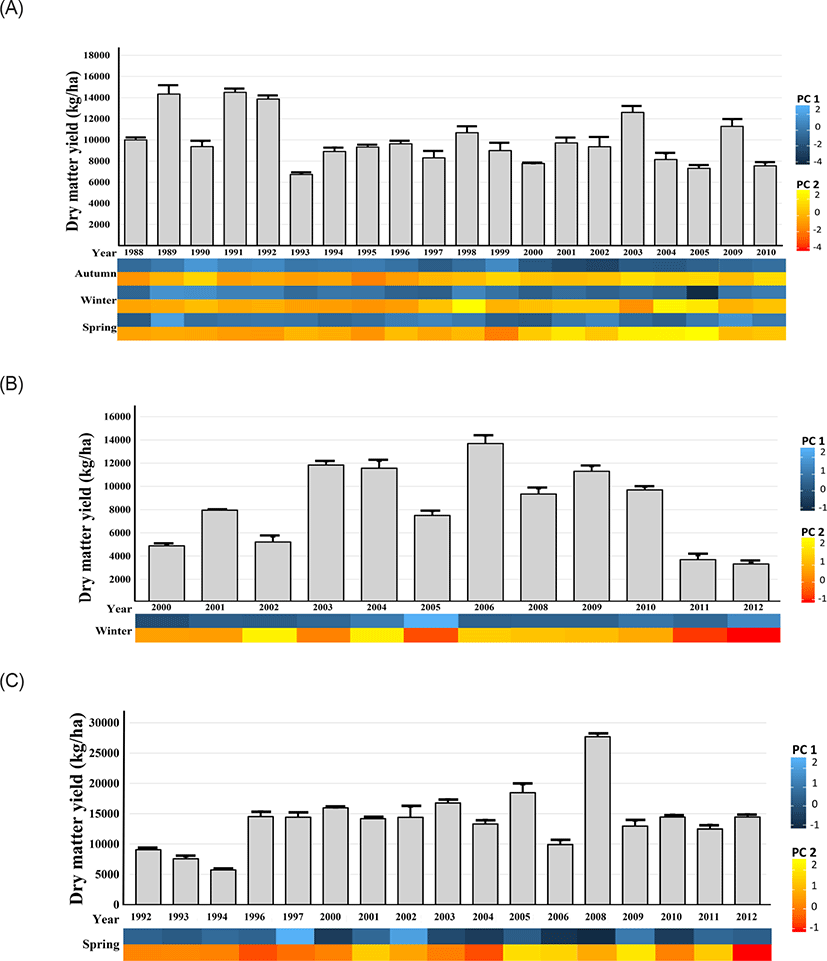
DISCUSSION
In this study, on abnormal climate events affecting the DMY of IRG, the abnormal climate events of severe winter cold and spring drought were considered in central inland, southern inland and southern coastal regions of Korea. All abnormal events were critical in the central inland region, while severe winter cold and spring drought events were fatal in the southern inland and coastal regions, respectively. The impact of severe winter cold event was greater in the southern inland region than in the central inland region, despite of lower winter MT in the central inland region. It concluded that despite frequent severe cold events in winter, yield damage was lower due to the cultivation of relatively strong cold-resistant varieties in the central inland region. In general, it is clear that wintering is difficult in the central inland region than in the southern inland region due to high temperature and humidity. Therefore, for the low yields in the southern inland region, it was concluded that other factors, such as soil physical properties and cultivation management for wintering, also contributed to the survival of IRG. According to Kim and Sung [18], IRG yield in paddy fields was lower than in upland fields due to the short growing period by the rice-IRG rotation system. Therefore, climate is the most important factor, but other factors in the severe cold below zero also affected the yield difference. Furthermore, the development and selection of new varieties have been intensively performed to expand to the central and northern regions of Korea [29]. Between normal and severe cold events in winter, the difference in MT was 1.05°C in the central inland region and 1.97°C in the southern inland region. This implies that not only frequency and intensity but the climatic fluctuation was important between normal and abnormal events. In both inland regions, increasing PC 2 related to winter MT was clear since 2000, but it was less important than PC 1 related to WS and RH. It is certain that the winters are getting warmer, but there is no scientific evidence that these changes lead to specific patterns for a severe cold event. Rather, it is likely that WS and RH were more involved in the severe winter cold event. Park et al. [30] reported a similar result that, in Korea, cold days tended to decrease with year, but there was no significant trend in cold surges.
In this study, the spring drought was fatal in central inland and southern coastal regions. In particular, the frequency of spring drought events in southern coastal regions was greater than normal; thus, the spring drought is abnormal in terms of intensity and production impact, but in terms of frequency, it seems to be a NEW NORMAL in Korea since 2000. There is no doubt that RH and PA were such important climate variables for the spring drought event that they were extracted on PC 1 regardless of region. Physically, the cooling of the air has the liquidizing properties of water vapor, so in inland regions where it is easily dried compared to coastal regions where the specific heat is low and sufficient moisture is maintained, the low RH in winter causes a severe cold [31]. The PA of spring drought in the southern coastal region was half as normal. For the spring drought in inland regions of the Korean Peninsula, spring PA in 2000 and 2001 was less than 50% of the average year, whereas spring PA was unusually high in 2003 [7,32]. The spring PA in the southern coastal regions is only 16 percent of the annual PA [33], and the Korean rainfall concentrated in the summer monsoon season will likely become even more severe, increasing the chances of a long dry season in spring [23]. As to the severity of the spring drought, Lee et al. [34] reported that if there was no rain for more than two weeks, concerns about agricultural drought were particularly high. However, it also reported that the spring drought response had improved and the damage had gradually decreased as the agricultural water facilities improved. Furthermore, the southern region was more susceptible to drought than the central region of the Korean Peninsula. Therefore, storage-oriented water management will be very important in the face of abnormal drought, such as regional torrential rainfall, increase in drought variability and incidence, and prolonged drought due to climate change. Finally, yield damage was the least for the low-temperature event in autumn, and this had significant impact only in the central inland region. However, given the noticeable trend of autumn low-temperature events in the central inland region since 2000, it was determined that there is a high risk of such events becoming prominent as a serious abnormal climate event in the future.
The information on climate events generated through this study will help to predict the production of winter crops under the climate event happening, and it can be expected to help to provide a guideline for cultivating IRG in abnormal climate events and to assess its vulnerability. Unfortunately, because of the small sample size, it is not possible to separate the impacts of abnormal climate events from two or more combinations of climate events by considering each seasonal climate event. Furthermore, the climate events were investigated based on monthly records without considering the growing stage of IRG; thus, it is difficult to reflect the duration and occurrence timing of climate events for the growth stages of winter crops. In the future, climate variables for the seasonal duration of abnormal climate events using daily records will be investigated to reflect the growth stage of IRG.
CONCLUSION
The study was carried out to assess the impact of abnormal climate events such as autumn low-temperature, severe winter cold and spring drought on the production of Italian ryegrass in the central inland, southern inland, and southern coastal regions of Korea. The yield damage caused by severe winter cold event was serious in both inland regions; in particular, the damage was more fatal in the southern inland region, even though the frequency of events was lower. In consideration of the trend and frequency of spring drought events, it is likely that drought becomes a NEW NORMAL during spring in Korea. Finally, the damage caused by low-temperature events in autumn was lower than other abnormal events, although its tendency had changed since 2000. The greater the difference in climate characteristics by year, the more the production fluctuations tended to change rapidly. Therefore, for the abnormal climate events related to winter forage crops in Korea, a strategic approach focusing on prediction and quick judgment is required, since we cannot be routinely prepared for the characteristics of rare events.