INTRODUCTION
As consumer’s incomes rise in many countries, there is a general tendency to shift from quantity towards higher pork quality, such as specialty cuts with reddish/pink color, flavor, and tenderness. The color of pork is considered as a key indicator of freshness and quality [1]; Intramuscular fat content (or marbling) is an important trait that is related to meat flavor [2]. Pork tenderness also strongly affects consumer satisfaction and thus, repeat buying [3]. The heritability of meat quality traits is low to moderate [2,4]. However, it is generally difficult to improve the meat quality-related traits using conventional breeding methods because the measurement of meat quality-related traits, such as pH, meat color, water-holding capacity, tenderness and marbling, can only be recorded and evaluated after slaughter. Therefore, molecular breeding techniques using genetic markers have emerged as potential alternatives for improving meat quality-related traits [5]. For example, RYR1, PRKAG3, PHKG1, and MYH3 were identified to enhance pork quality in the form of genetic markers [6–9].
Nowadays, DNA array chip technology, which is a highly parallel genomic assay integrated with high-density single nucleotide polymorphism (SNP) markers, has been developed and is available for identifying genes that affect complex and quantitative traits, such as meat quality-related traits [10]. The DNA array chips can provide genotype data for conducting genome-wide association studies (GWAS) to identify quantitative trait loci (QTLs) and their positional candidate genes for marker-assisted selection (MAS), which can also be used for conducting genomic selection (GS) to improve pork quality-related traits. Results from MAS and GS can efficiently and quickly contribute to the genetic improvement of pork quality by selecting piglets with genetic potentials for excellent meat quality-related traits [11].
This study was performed to detect QTL and their positional candidate genes that could be used to develop potential genetic markers to improve pork quality in a purebred Yorkshire population. In addition, we investigated the biological functions of the identified positional candidate genes affecting the variation in the meat quality through the analysis of biological pathways.
MATERIALS AND METHODS
This study was conducted in accordance with the guidelines of the Institutional Animal Care and Use Committee of the National Institute of Animal Science, the Republic of Korea (2015-137).
Using the OMNI Bead Ruptor (OMNI International, Kennesaw GA, USA) and DNeasy® Blood & Tissue Kit (QIAGEN, Hilden, Germany), genomic DNA was extracted from 50 mg of frozen muscle tissue of 543 pigs from a purebred Yorkshire population (406 castrated male pigs and 137 female pigs) originated from a GP (grandparents, single multiplier) breeding farm in the Republic of Korea. The experimental animals were born in the GP farm from July 2015 to November 2016 that was a closed population and later transported to nine breeding stock farms and raised until the slaughter. The pigs were slaughtered at an average (±SD) age of 200 (±11.7) days and the mean carcass weight (± SD) was recorded as 88.0 (± 9.3) kg.
Longissimus dorsi muscle samples were isolated from the carcass and meat pH 24 hr postmortem (pH24), redness (a*), yellowness (b*), cooking loss (cooking), shear force (shear), National Pork Producers Council (NPPC) meat color (Ncol), and NPPC marbling (Nmar) traits related to the meat quality were measured according to the method reported by Choe et al. [12].
Before the GWAS, we obtained the descriptive statistic values and validated the normal distribution of the phenotypic data. Putative outliers were detected based on the ascertainment of normality using the Ryan-Joiner method implemented in the Minitab program (Minitab, State College, PA, USA). The phenotypic values were transformed by natural logarithm [i.e., NPPC meat color, NPPC marbling] or square root [i.e., shear force] as necessary (Table 1). General linear model (GLM) analysis was conducted using the Minitab program (Minitab) to identify sources influencing phenotypic variation. The effects of sex (castrated male, female), farm, season (summer season, and other seasons), and carcass weight were evaluated. A highly significant effect (p < 0.01) of carcass weight (in the case of a*, b*, cooking loss, shear force, Ncol, and Nmar) was observed, and, therefore, included in the linear model for GWAS. Significant effects (p < 0.05) of sex (in the case of b*, Ncol, Nmar), season (ph24, b*, cooking) and farm (ph24, a*, b*, cooking, shear, Ncol) were also observed. Therefore, they were included as cofactors (i.e., sex, farm, and season) and a covariate (i.e., carcass weight) in the GWAS model.
SNP marker genotypes were determined from genomic DNA samples of 543 purebred Yorkshire pigs using the Axiom Porcine Breeders array (Thermo Fisher, Waltham, MA, USA). SNP markers with minor allele frequency (MAF) less than 1%, genotyping error rate greater than 10%, and Hardy-Weinberg equilibrium less than 10−6 were removed from the analyzed SNP makers using PLINK program version 1.9 [13]. A total of 45,926 SNP markers on 18 autosomal chromosomes were left after the quality control procedure.
Estimation of the heritability of each meat quality-related trait in this study was conducted using the following linear mixed model (LMM):
where y is the vector of the phenotype of interests and b is the vector for fixed cofactors (sex, farm, and season) and a linear covariate (carcass weight); u is the vector of random additive genetic effects following a normal distribution u~ N(0, G), in which G is the genomic relationship matrix (GRM) whose matrix elements are consisted of pairwise genomic relationship coefficients calculated using the genotypes of 42,399 SNP markers, and is the additive genetic variance component; e is the vector of random residuals following a normal distribution e~N(0, I), in which I is the identity matrix, and is the residual variance component. The GEMMA program was used for building up the GRM and the estimation of the and for each meat quality-related trait based on the restricted maximum likelihood method (REML). The X and Z are the incidence matrices of b and u, respectively.
The GWAS for the meat quality-related traits was performed for 543 Yorkshire pigs based on a single-marker univariate LMM using GEMMA software [14]. The LMM equation for GWAS (Eq. 2) was as follows:
where Y is the univariate phenotype; a is the vector of fixed effect of the SNP marker; b, u, and e are the same vectors used in Eq. 1. The X, Z1 and Z2are the incidence matrices of b, a, and u, respectively.
The percentage of phenotypic variance explained by an SNP (%VarSNP) was computed as follows (Eq. 3) [15]:
where p is the minor-allele frequency of the SNP marker; α is the additive genetic effect of the SNP marker; is the phenotypic variance for each meat quality-related trait. The p, α and were estimated using the GEMMA program. To address multiple comparison issues in GWAS, the significance threshold level was determined using the q-value method; a q-value less than 0.1 (q < 0.1), which corresponds to p < 2.48E-06, was designated as the threshold of the significance level; a q-value that is 0.1 or more and less than 0.2 (0.1 ≤ q < 0.2), which corresponds to 2.48E-06 ≤ p < 2.55E-05, was designated as the threshold of the suggestive level [16]. The effects of the trait-associated SNP markers on the phenotypes were estimated using the GLM implemented in Minitab (Minitab). Statistical power analysis of the GWAS was conducted using the method developed by Wang and Xu [17] for LMM-based GWAS under different assumptions regarding the sample size. The CMplot package implemented in R was used to visualize the GWAS results [18]. The Ensembl database (URL: http://asia.ensembl.org/Sus_scrofa/Info/Index) based on Sus scrofa 11.1 was used to select the genes that were close to the trait-associated SNPs as positional candidate genes.
After selecting the SNP marker group with a nominal p-value less than 0.01 based on the results of the GWAS analysis, a list of positional candidate genes of the SNP marker group was prepared using the biomaRt in the R package [19]. The analysis of biological pathways between genes was performed using the Kyoto Encyclopedia of Genes and Genomes (KEGG) library of the Enrichr database, and a p-value of 0.05 was used as a significance level to detect a significant biological pathway [20,21].
RESULTS AND DISCUSSION
Phenotypic data analysis was performed on seven meat quality-related traits collected from 543 purebred Yorkshire pigs. The overall means, SD and ranges of the traits of interests are presented in Table 1. The observed phenotypes did not display obvious deviations from the normality assumption (Fig. 1). The phenotypes recorded for this study showed Among them, mean ± SD of yellowness and shear force were 1.81±1.14 and 7.47±0.86, respectively (Table 1). Heritability estimates range from 0.087 (ph24) from 0.28 (a*) (Table 1).
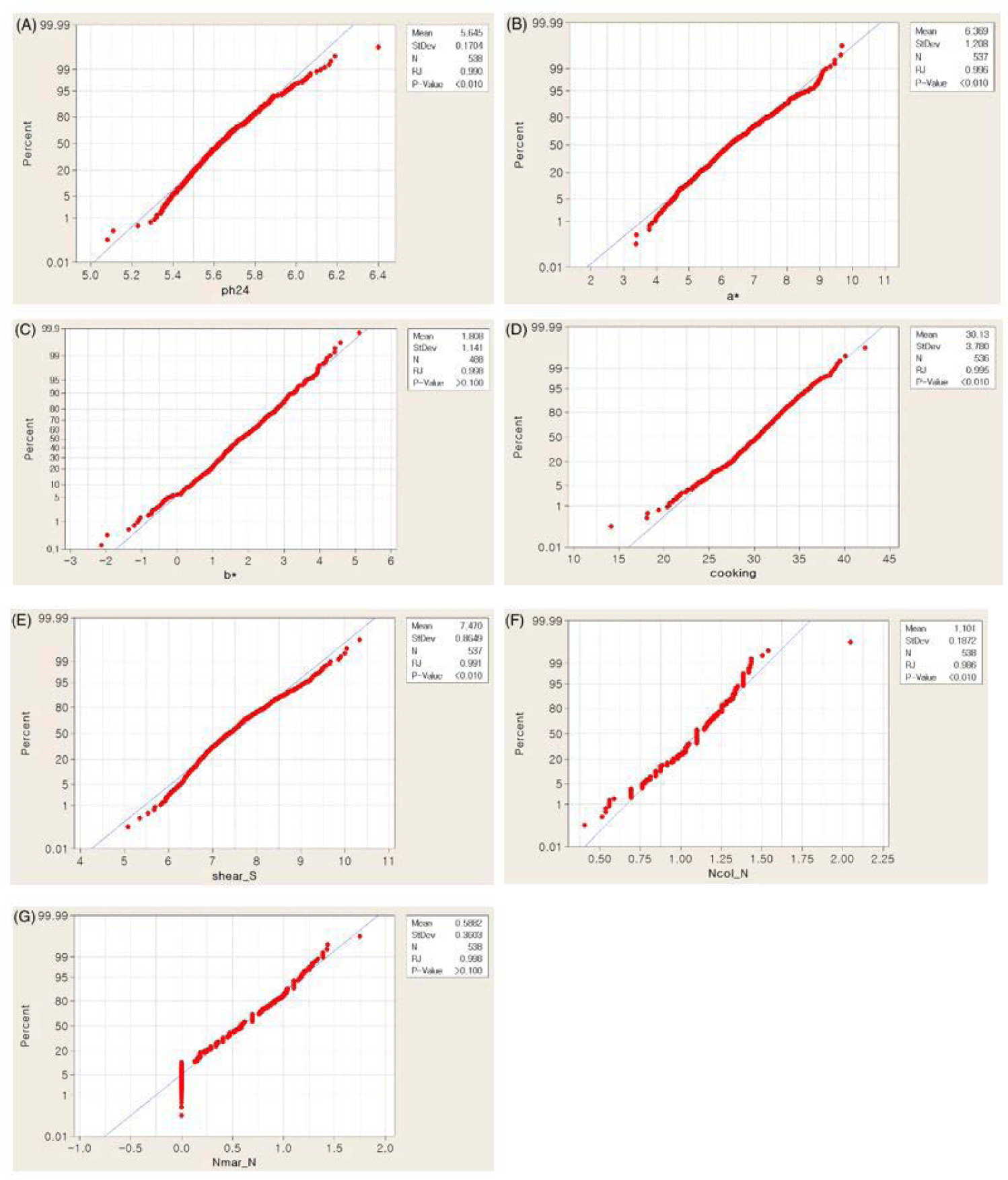
As a result of the GWAS for seven traits related to the meat quality using the study population, two trait-associated SNP markers on porcine chromosome 2 (SSC2, AX-116172218, and AX-116679656) and one trait-associated SNP marker on the chromosome 8 (SSC8, AX-116692048) were identified as significant and suggestive QTLs (Fig. 2) (Table 2). The QTLs identified in SSC2 affected the meat color (b*). In a study of European commercial pigs by Harmegnies et al. [22], the QTL region was overlapped with the meat color (b*) QTL identified in this study. The QTL identified on SSC8 was found to affect the shear force trait; however, the QTL identified on SSC8 is yet to be reported based on the Animal QTLdb search [23]. The degree of inflation of the GWAS results (i.e., lambda values) revealed that the effect of population structure due to the genetic relationship between individuals on the association results was insignificant in this Yorkshire study population (Fig. 2). For the rest of meat quality-related phenotypes, our GWAS did not reveal the presence of any statistically significant or suggestive QTLs (Fig. 2).
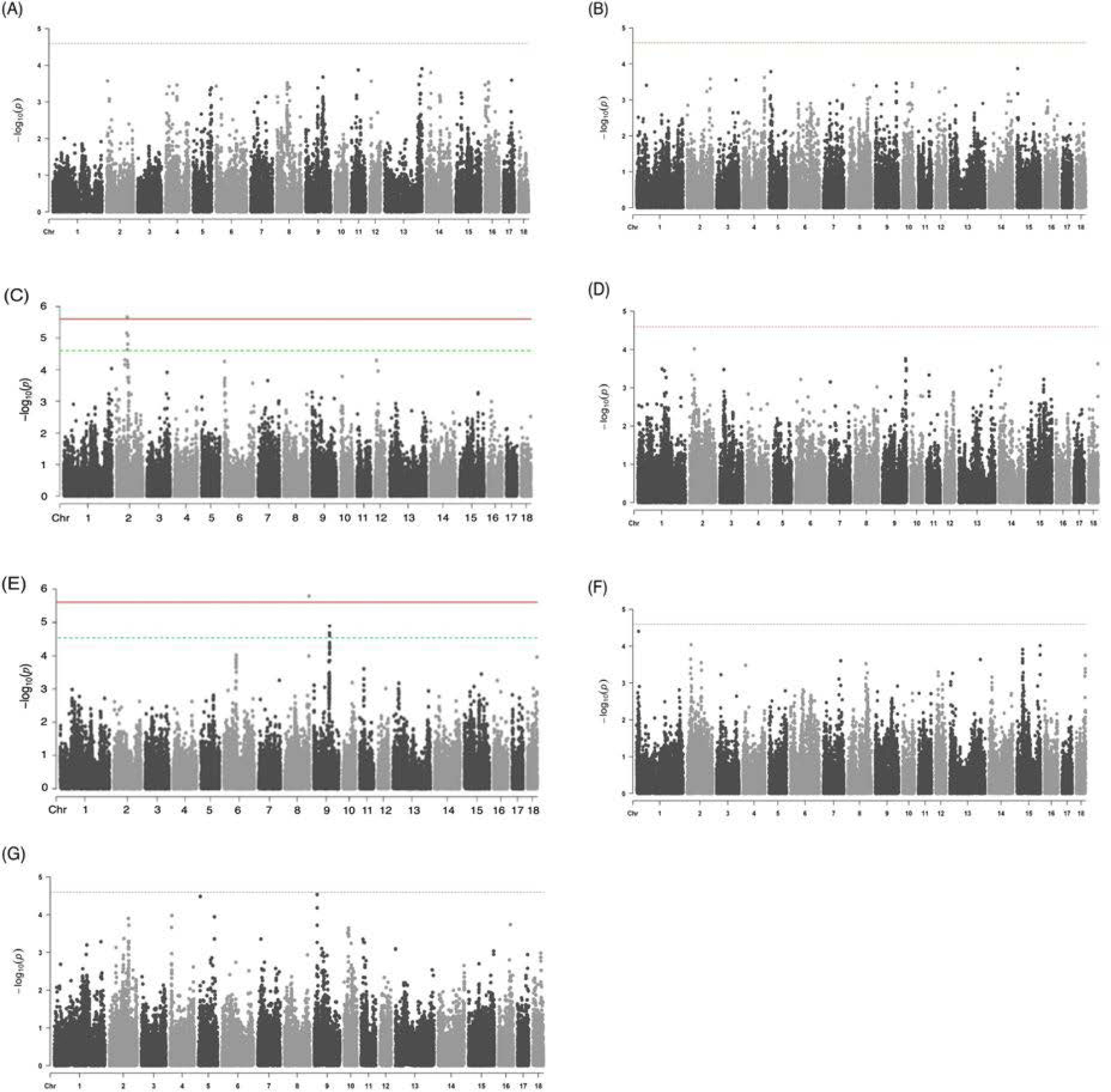
The two SNP markers (AX-116172218 and AX-116679656) in the QTL region identified in SSC2 explained 4.25% and 3.92% of the phenotypic variance of the meat color (b*) trait, respectively, whereas the SNP marker (AX-116692048) in the QTL region on SSC8 accounted for 4.57% of the phenotypic variance in shear force (Table 2). The effects of trait-associated SNP markers on the phenotypes are shown in Fig. 3. The results show that the T alleles is positively associated with b*, whereas the A allele was positively associated with shear force. The genic action was mostly additive for the two traits examined.
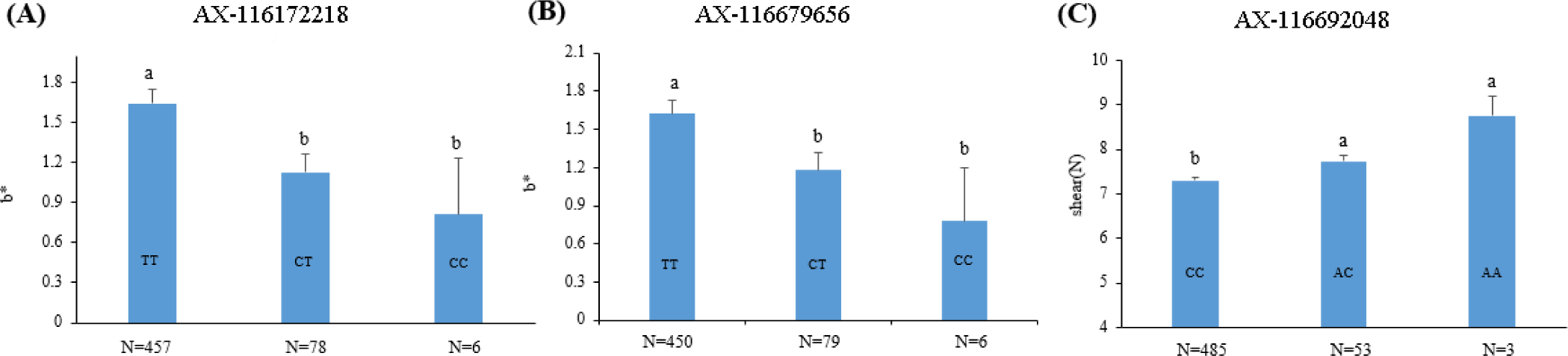
Considering the effects of population structure and polygenic background using the DNA array chip data, we calculated the statistical power of GWAS to detect a QTL under the currently used sample sizes (i.e., 448 for b* and 537 for shear force) and a significance threshold of a p-value = 2.48E-06 corresponding to q = 0.1 [17]. When the p-value of 2.48E-06 was used, the calculations indicated that a sample size of 537 (the case for shear force) was necessary to achieve 95.0% statistical power to identify a trait-associated marker with a %VarSNP value of 5.2%. For a sample size of 448 (the case for b*), the calculations indicated that 74.1% and 81.6% statistical power could be achieved with %VarSNP values of 3.9% and 4.3%, respectively. This result suggests that the use of the moderate sample sizes in this study provided sufficient statistical power to identify a biologically meaningful QTL [17].
The HAUS augmin-like complex subunit 8 (HAUS8) gene was identified as a positional candidate gene in the QTL region of AX-116679565, and lncRNA was detected as a positional candidate gene in the QTL region of AX-116692048. HAUS8 on SSC2 has been reported to be related to cytoskeletal tissue and the microtubule system, which play essential roles in cell migration [24]. The identified lncRNA gene in SSC8 produces a long noncoding RNA consisting of more than 200 nucleotides. lncRNA affect post-transcriptional modifications and serves various functions within cells after forming extensive networks of ribonucleoprotein complexes with many chromatin regulators [25,26]. lncRNA is considered a candidate gene for traits related to the muscle development process, such as shear force, because it is expressed during the muscle development process, and is known to regulate the proliferation, differentiation, and fusion of myoblasts [27]. By running the biomaRt for SNP marker groups with a p-value less than 0.01, 162 and 142 genes were detected as positional candidate genes that could affect meat color (b*) and shear force traits, respectively.
The Enrichr database was used to search for biological pathways related to b* and shear force. The identified biological pathways and the positional candidate genes belonging to them are shown in Table 3. Among them, four positional candidate genes (integrin-binding sialoprotein; IBSP; dentin matrix acidic phosphoprotein 1, DMP1; FRAS1 related extracellular matrix 2, FREM2; dentin sialophosphoprotein, DSPP) were detected for extracellular matrix (ECM)-receptor interactions. ECM-receptor interaction is known to affect the differentiation of intramuscular adipocytes in chicken, and it has been reported that this could be implicated in meat quality [28]. In addition, in the Hippo signaling pathway, four positional candidate genes (discs large MAGUK scaffold protein 2, DLG2; frizzled class receptor 4, FZD4; discs large MAGUK scaffold protein 4, DLG4; bone morphogenetic protein 5, BMP5) were also detected. It has been reported that this pathway promotes the proliferation of skeletal muscle stem cells or is closely related to muscle growth and development, and adipocyte proliferation and differentiation [29].
The most significant biological pathway associated with the shear force trait was the glycosaminoglycan degradation (GAG) pathway with the hyaluronidase 1 (HYAL1) and hyaluronidase 3 (HYAL3) genes. This pathway is primarily found in mucopolysaccharides, connective tissues, bone tissues, intercellular mediators, and epithelial tissues and has been reported to be closely related to the regulation of proliferation and differentiation of muscle cells [30]. A significant association with shear force was also detected for the glycerolipid metabolism pathways that include diacylglycerol kinase beta (DGKB), glycerol-3-phosphate acyltransferase 3 (GPAT3), and diacylglycerol kinase eta (DGKH) genes. This pathway is closely related to the synthesis, transportation and esterification of fatty acids involved in the increase of intramuscular fat in chicken breast tissue [31]. The phosphatidylinositol signaling pathway, which includes DGKB, phosphatidylinositol-5-phosphate 4-kinase type 2 alpha (PIP4K2A), and DGKH genes was also found to be associated with shear force. This pathway is involved in cell proliferation, survival, and metabolism; it plays an essential role in cell signaling, such as the insulin signaling pathway, and is closely related to endocytosis and exocytosis [32,33]. The insulin metabolism plays an important role in the formation of fat, which affects the meat quality of Korean native cattle (Hanwoo) by promoting marbling by accumulating the remaining glucose in the body [34].
In addition, pathways of choline metabolism in cancer, glycerophospholipid metabolism, and ABC transporters were detected, Further studies on the relationship between these pathways and shear force are needed. Based on the results of the biological pathway analysis, it is thought that candidate genes affecting meat color (b*) affect differentiation of intramuscular adipocytes, growth, and development of muscles, and fat accumulation in muscles, and that candidate genes for the shear force trait are related to the growth and differentiation of cells and synthesis of fatty acids.
CONCLUSION
QTLs affecting the meat quality-related traits were to identified using a purebred Yorkshire population, and biological pathways that could affect meat color (b*) and shear force traits were detected by identifying positional candidate genes in the QTL region. The results of this study can be utilized as basic molecular genetic data to improve the meat quality of pork after passing verification procedures in other independent populations in the future.