INTRODUCTION
Feed cost is an important economic expenditure of pig breeding industry, accounting for more than 60% of the entire of pig breeding cost [1,2]. Improving feed efficiency (FE) is an effective strategy to reduce feed cost in the pig industry. Residual feed intake (RFI) is widely used to measure the FE [3]. RFI is defined as the difference between the actual feed intake and the predicted feed intake, the latter is calculated based on the intake amount required for maintenance and growth during a certain period [4,5]. The heritability of RFI has been reported to be between 0.10 and 0.42 in pig, which is moderate heritability [6–8], thus there is much room for raising pig FE by improving RFI. Low RFI denotes high efficiency at converting feed into body mass [9,10].
The selection of RFI in pigs not only improves FE, but also changes energy metabolism, which can explain the variation mechanism of RFI in pigs. It has been reported that low-RFI pigs with longissimus muscle have high glycogen content and low activities of metabolic enzymes involved in glycolytic pathway, fatty acid oxidation pathway, and energy balance [11]. In addition, low-RFI pigs exhibit the low activities of lactate dehydrogenase involved in glucose metabolism and hydroxylacylCoA dehydrogenase involved in fatty acid oxidation [12]. Mitochondria is the main site for energy metabolism. Moreover, in the low-RFI line, the reactive oxygen species production in the white portion and red portion of the semitendinosus is reduced in the mitochondria [13]. Although the effect of RFI selection on animal metabolism can partly explain the mechanism of RFI variation, the underlying mechanism of FE remains largely unknown.
MicroRNAs (miRNAs), a class of small endogenous noncoding RNAs with 19 to 25 nucleotides, play important roles in post-transcriptional regulation [14,15]. MiRNAs have been reported to be related to FE. A total of 25 DE miRNAs have been identified in longissimus dorsi of significantly different RFI pigs, of which, miR-208, miR-29c, and miR-1 are related to skeletal muscle growth and development [1]. In cattle, 25 miRNAs are differentially expressed (DE) in liver of high and low RFI individuals, among which, bta-miR-143, bta-miR-122, bta-miR-802, and bta-miR-29b are mainly related to glucose homeostasis and lipid metabolism [16]. It has been reported that bta-miR-486, bta-miR-7, bta-miR15a, bta-miR-21, bta-miR-29, bta-miR-30b, bta-miR-106b, bta-miR-199a-3p, bta-miR-204, and bta-miR-296 are mainly involved in such signaling pathways as insulin, lipid, immune system, oxidative stress response, and muscle development, and they are also associated with RFI in cattle [17].In addition, miR-665, miR34a and miR-2899 may regulate cattle RFI by controlling 14-3-3 epsilon and heat shock protein family B (small) member 1 (HSPB1) proteins [18]. These results indicate that miRNAs play an important role in regulating FE.
Liver, as a complex metabolicorgan, affects the distribution of nutrients, and it regulates the muscle and lipid generation by affecting energy metabolism, thus it is an important organ for regulating FE [19,20]. In this study, miRNA-sequencing was performed to comprehensively analyze a miRNA expression in the liver of high- and low-FE pigs. Subsequently, the relationship between our DE miRNAs and the previously reported DE genes analyzed. Our study may provide an insight into the molecular mechanism of FE in pigs.
MATERIALS AND METHODS
In this study, 236 castrated boars from population of Yorkshire pigs were raised in ACEMA64 (ACEMO, Pontivy, France) automated individual feeding systems in the Agricultural Ministry Breeding Swine Quality Supervision Inspecting and Testing Center (Wuhan, China) [1]. Based on the FE measurements, the performances of 30 animals with the lowest RFI (high FE) and 30 animals with the highest RFI (low FE) were compared (Table 1). On average, pigs in the high-FE group consumed significantly less feed per day than pigs in the low-FE group, and there was a reduction in fat deposition, which is consistent with the results reported in other literatures[12,21–23]. The individuals with extreme FE differences (3 vs. 3) were selected based on the RFI value for miRNA sequencing, and there was no difference in body weight between these individuals (Table S1). Liver tissue samples of each pig were collected after slaughter, immediately frozen in liquid nitrogen within 30 minutes, and stored at −80°C. Total RNA was extracted from the frozen liver samples using TRIzol regent for miRNA sequencing (Invitrogen, Carlsbad, CA, USA). All experimental protocols were approved by the Ethics Committee of Huazhong Agricultural University (HZAUMU2013-0005).
The total RNA of each liver sample was used for small RNA library construction. The miRNA sequencing library of each sample was prepared with TruSeqR Small RNA library Kit (Illumina, San Diego, CA, USA) according to manufacturer’s instructions. After quality control, six miRNA libraries were sequenced on Illumina HiSeq3000 platform at the Genergy Biotechnology, shanghai, China.
The clean reads of miRNA were obtained from raw data after trimming adapters and filtering low-quality reads. Then, clean reads were mapped to the reference genome of Sus scrofa v. 11.1 (http://ftp.ensembl.org/pub/release-104/fasta/sus_scrofa/dna/) with miRdeep2 [24]. The reference genome was downloaded from Ensembl (EMBL-EBI, Hinxton, Cambs, UK), and the miRNA reference sequences were obtained from the miRBase database (version 22) (The University of Manchester, Manchester, UK). The expression level of each miRNA was normalized according to the following formula: Normalized read count = Actual miRNA count/Total clean read count ×1000000 [25–27]. The known miRNAs were verified and novel miRNAs were predicted by the MiRDeep (v2.0.0.7) software (Max Delbrück Center for Molecular Medicine, Berlin, Germany) [28]. The sequences mapped to the pig reference genome were considered as potential miRNA sequences. The miRNAs whose sequences matched those of mature miRNAs in miRBase20.0 were identified as known miRNAs. Novel miRNAs were predicted based on unmatched sequences by MiRDeep2, and the secondary structures of novel miRNA were predicted by RNAfold (v2.0.1) (University of Vienna, Vienna, Austria) [29].
The R package of DESeq (v4.0.3) (European Molecular Biology Laboratory, Heidelberg, Germany) [30] was used to analyze the differences in miRNA expression level between the high-FE and low-FE pigs. The Fold change between high-FE and low-FE was calculate according to the following formula: |log2 (Fold change)|= log2(high-FE/low-FE). The p-value between the two groups was calculated using the following formulas:
among them N1 and N2 represent the total count of clean reads in miRNA libraries of high-FE and low-FE liver tissue samples, respectively; x and y represent the normalized expression levels of a given miRNA in miRNA library of high-FE and low-FE liver tissue samples, respectively [31]. The DE miRNAs were identified according to the criteria of p-value < 0.05 and | log2 (Fold change) | ≥ 1.
The relative expression levels of the DE miRNA in liver tissues were quantified by real-time qRT-PCR. Three high-FE samples and three low-FE samples were used for qRT-PCR analysis. The specific primers of miRNAs are listed in Table S2. The miRNA reverse transcription was performed with Thermo Scientific Revert Aid First Strand cDNA synthesis Kit (Thermo Fisher Scientific, Waltham, MA, USA). The pig U6 snRNA was used as the internal control. The miRNAs were quantified on Roche Lightcycler 480 Sequence Detection System (Roche Holding AG, Basel, Switzerland) according to the instruction manual. The 2−ΔΔCt method was used to analyze the relative expression levels of miRNAs, and the Student’s t-test was used to analyze the expression difference between the high-FE and low-FE pigs.
To explore the functions of significantly DE miRNAs between high-FE and low-FE pig, the miRNA target genes were predicted using DIANA miRPath (http://snf-515788.vm.okeanos.grnet.gr/) (University of Thessaly, Volos, Greece) with homologous human miRNAs.
The GO enrichment analysis (with expression analysis systematic explorer [EASE] < 0.01), and KEGG pathway analysis (with EASE scores = 0.1) were performed using DAVID Bioinformatics Resources (https://david.ncifcrf.gov/) (National Cancer Institute at Frederick, Frederick, MD, USA).
We selected the differentially expressed genes (DEGs) in livers of High and Low FE pigs, which were also targeted by DE miRNAs, based on our previous study results [32]. These genes were considered as the potential core genes. To identify all possible miRNA-mRNA interactions, the regulatory networks between DE miRNAs and their target mRNA were visualized using an open source software—Cytoscape v3.6.1 (Institute for Systems Biology, Seattle, Washington, USA.) [33].
RESULTS
To identify DE miRNAs between high and low FE groups (n = 3 in each group, Table S1), six small RNA libraries of the liver tissues from high and low FE pigs were constructed for solexa sequencing. After sequencing, 15.78–38.56 million raw reads per sample were obtained. After eliminating the adaptor sequences and filtering low quality reads and short fragments (less than 18nt), 15.24–32.20 million clean reads per sample were obtained, accounting for 83.48%–97.32% of the raw reads (Table 2). The length distribution of most clean reads ranged from 21 to 23 nt, and the length distribution peak was 22 nt (Fig. S1). This result was consistent with the length range of miRNA.
The clean reads were aligned to the precursor and mature miRNAs in the miRBase 22.0 database. In total, 218, 213, 212, 222, 215, and 221 mature annotated porcine miRNAs were identified in the high-FE-126, HIgh-FE-130, high-FE-160, low-FE-302, low-FE-306, and low-FE-307 respectively (Table S3). A total of 188 miRNAs were co-expressed in these six individual pigs, of which 77 mature miRNAs were abundantly expressed in livers of High-FE and low-FE pigs, and 2 miRNAs (ssc-miR-7139-5p, ssc-miR-144) were specifically expressed in the low-FE group (Fig. S2). The top 20 mature miRNAs with largest read count were listed in Fig. 1.
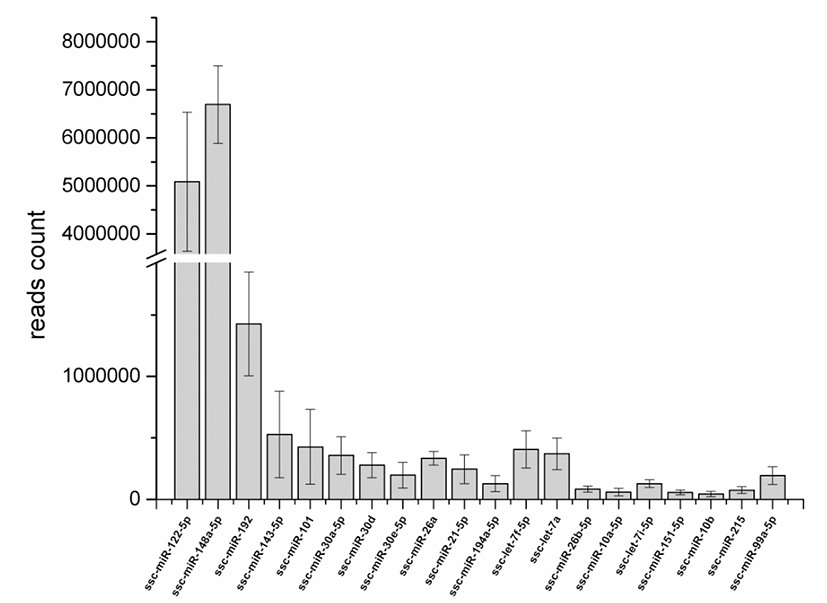
The miRDeep2 was used to identify novel miRNAs from sequencing data (Table S4, Fig. S3), and predict their precursor sequences and hairpin structure (Fig. S4). In total, 136, 151, 113, 184, 281, and 242 novel miRNAs were identified to be homologous to human or mouse in the six individuals (high-FE-126, high-FE-130, high-FE-160, low-FE-302, low-FE-306, and low-FE-307). Among these newly identified miRNAs, 28 miRNAs were co-expressed in all six individuals, and one miRNA was specifically expressed in in high-FE pigs and 24 miRNAs were specifically expressed in low-FE pigs. Since the expression levels of most novel miRNAs were relatively low in our results, they were not further analyzed.
To explore the relationship of miRNAs and FE in liver, we compared the expression patterns of the miRNAs in liver between high-FE and low-FE pigs. In our study, 14 DE miRNAs were identified between high-FE group and low-FE group, of which five miRNA were downregulated and nine miRNA were upregulated in high-FE pigs relative to low-FE pigs (Fig. 2, Table 3). Two of these identified DE miRNAs (ssc-miR-10386 and ssc-miR-1839-5p) were not homologous with those of human, but the remaining 12 miRNA were homologous with 12 human miRNAs (Table 3). Cluster analysis of these 14 DE miRNAs exhibited the expression patterns of miRNAs in different samples (Fig. 3).
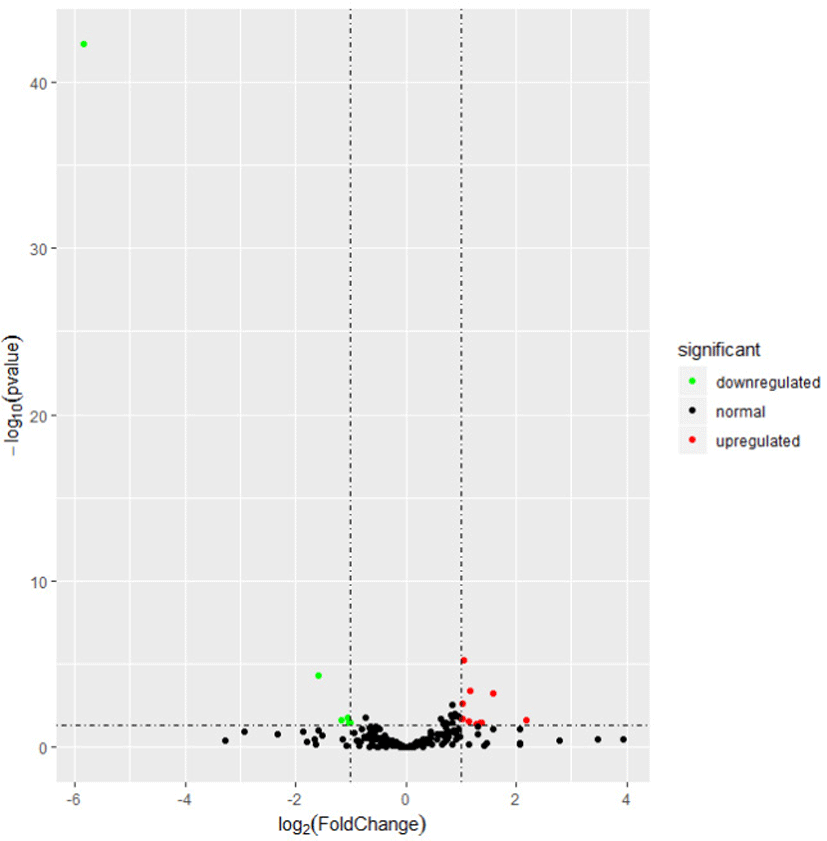
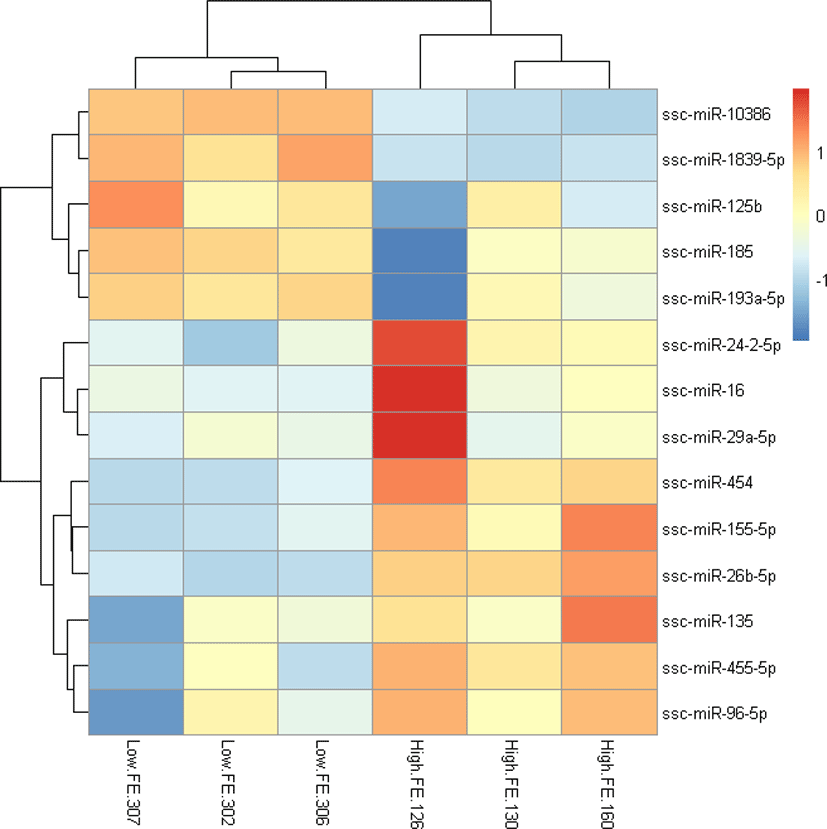
To verity the reliability of the miRNA sequencing data, five DE miRNAs (ssc-miR-26b-5p, ssc-miR-155-5p, ssc-miR-185, ssc-miR-125b, ssc-miR-193a-5p) were randomly selected for qRT-PCR analysis. Compared with that in high-FE liver, the expression level of ssc-miR-26b-5p and ssc-miR-155-5p in low-FE liver was significantly downregulated, whereas the expression level of ssc-miR-185, ssc-miR-125b, and ssc-miR-193a-5p was significant upregulated. These qRT-PCR results were consistent with the miRNA-sequencing data, indicating the reliability of miRNA sequencing data (Fig. 4).
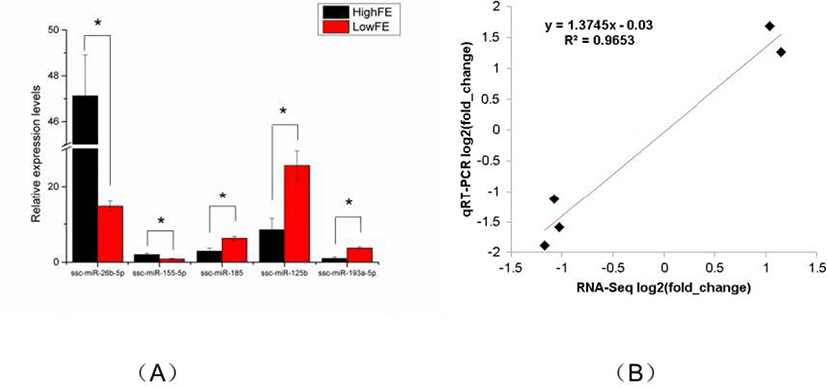
To examine the functions of the DE miRNAs in the comparison of high-FE pigs vs. low-FE pigs, the target genes of the DE miRNAs homologous to human were predicted. The results indicated that 7025 target genes of DE miRNAs were predicted which included 5118 unique genes (Table S5). Among these target genes, Fatty acid synthase (FASN), lysosomal associated membrane protein 3 (LAMP3) and fatty acid elongase 7 (ELOVL7) have been reported to be DE in liver tissues of high-FE and low-FE pigs [32].
The GO enrichment analysis showed 5118 target genes were mainly enriched in 3 GO categories (biological processes, cellular components, and molecular functions). The 515 GO terms were significantly enriched in biological processes, 127 GO terms were significantly enriched in cellular components, and 162 GO terms significantly enriched in molecular functions (Table S6). The top 20 biological processes in which the target genes were enriched were related to transcription (DNA-templated), regulation of transcription (DNA-templated), positive regulation of transcription from RNA polymerase II promoter, and negative regulation of transcription from RNA polymerase II promoter. The cellular components in which most target genes were enriched were mainly associated with nucleus, cytoplasm, cytosol, nucleoplasm, and membrane. The molecular functions in which most target genes were enriched were mainly related to protein binding, metal ion binding, DNA binding, ATP binding, and transcription factor activity (sequence-specific DNA binding). The top 20 significant GO terms in each of 3 GO categories were shown in Fig. 5.
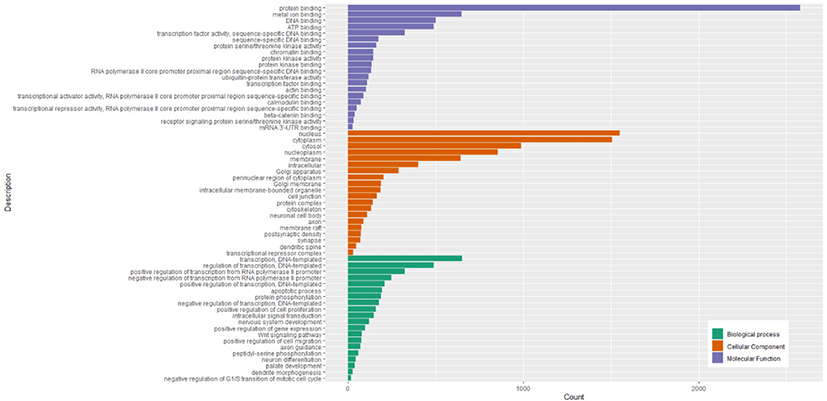
The miRNA target gene KEGG pathway analysis showed that the target genes of miRNAs were mainly enriched in 88 pathways (Table S7), and the top 20 pathways were shown in Fig. 6. Most of these enrichment pathways were associated with the growth and development such as PI3K-Akt signaling pathway, insulin signaling pathway, mTOR signaling pathway, Wnt signaling pathway, GnRH signaling pathway, transforming growth factor (TGF)-beta signaling pathway, and hypertrophic cardiomyopathy (HCM). Hierarchical clustering analysis was further performed to elaborate the relationship between DE miRNAs and their target pathways (Fig. 7). The miRNAs with the similar functions were clustered together.
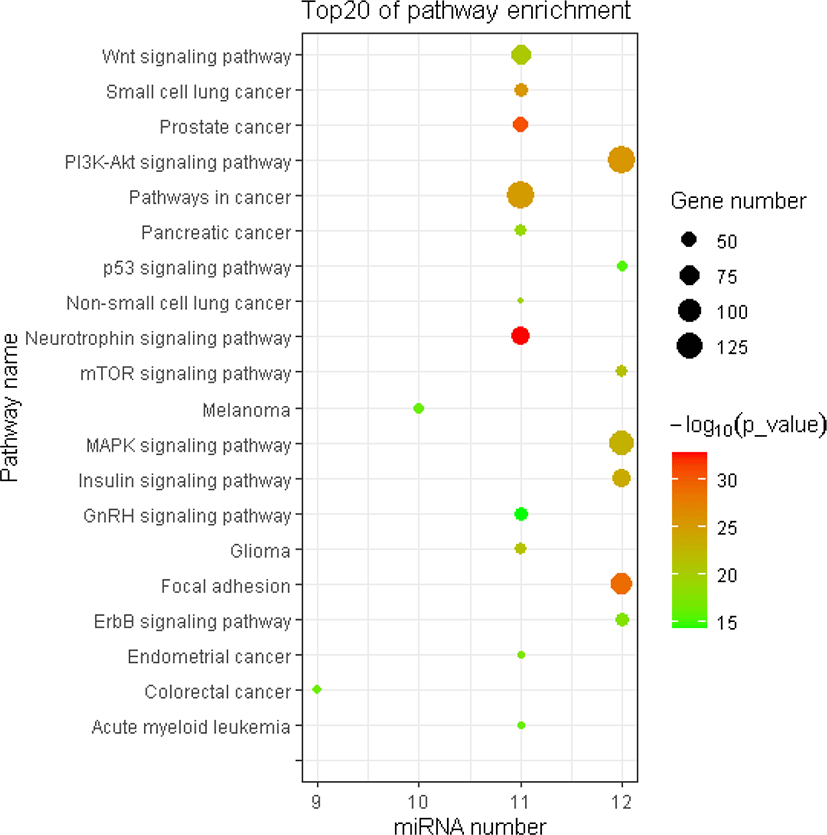
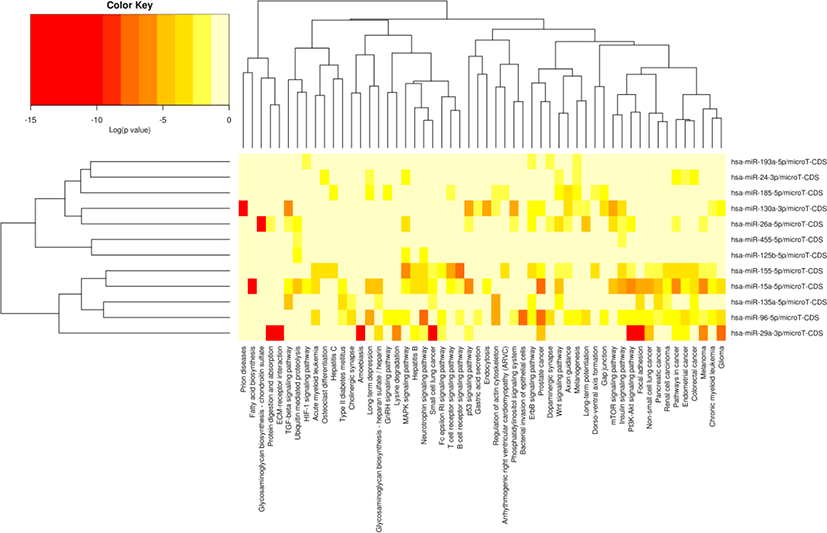
To clarify the molecular mechanisms of the FE trait, miRNA-mRNA association analysis of liver tissues in high-FE and low-FE pigs was conducted based on our previous study results [32]. To explore the potential roles of the miRNA in regulating target gene expression, we examined 532 well annotated DEGs (Table S8) and 14 DE miRNAs. Ninety-eight DE targets genes were identified from 11 miRNAs in the livers between high and low FE pigs (Fig. 8).
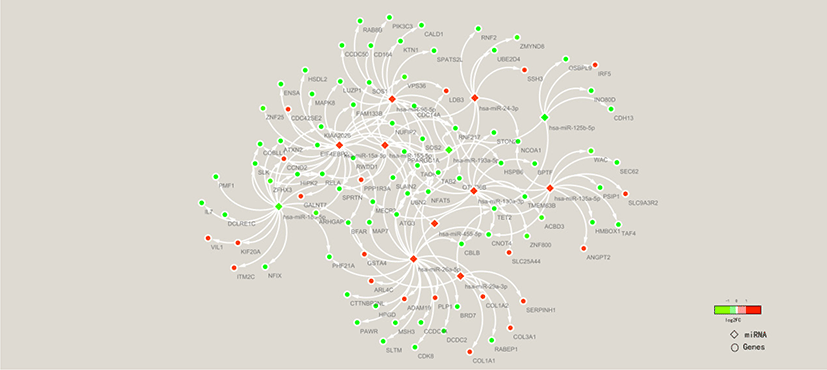
DISCUSSION
High-RFI (low-FE) and low-RFI (high-FE) pigs were chosen to identify the miRNA related to FE. The low-RFI pigs have higher conversion efficiency and lower energy metabolism, meaning that the energy intake of low-RFI pigs is mainly used for protein deposition while reducing fat accumulation [11,12,34–36]. In addition, phenotypic comparisons between high-FE and low-FE pigs showed lower feed intake and fat deposition in low-FE pigs [21]. Thus, animals with lower RFI are higher efficient at converting feed into body mass, whereas those with higher RFI have lower FE. Therefore, the improvement of FE could effectively reduce feed intake and feed cost. The miRNAs are important post-transcriptional regulators of gene expressions and participate in many biological processes [37]. In this study, we systematically analyzed the miRNA profiles of liver tissues in high-FE and low-FE pigs. The FE-related DE miRNAs and important FE-related signaling pathways were identified in this study. It have been reported that carbohydrate metabolism, lipid metabolism, hepatic lipid accumulation and Metabolism of xenobiotics by cytochrome P450 and butanoate and tryptophan Metabolism are associated with FE in pigs [38–42]. A number of miRNAs relate to carbohydrate metabolism (miR-135a-5p, miR-29a-3p, miR-15a-5p, miR-96-5p, miR-155-5p, miR-26a-5p, miR-185-5p, and miR-125b-5p), lipid metabolism (miR-16 and miR-135a-5p), hepatic lipid accumulation (miR-130a, miR-125b, miR-185, and miR-26a) and Metabolism of xenobiotics by cytochrome P450 and butanoate and tryptophan Metabolism (miR-185, miR-29a, miR-135a, miR-130a, miR-125b, miR-26a, miR-15a, and miR-96, miR-155, and miR-24, miR-130a, miR-26a, miR-15a) were DE between high FE and low FE pigs.
The top 2 highly expressed miRNAs were ssc-miR-122-5p and ssc-miR-192 in both high-FE and low-FE pigs. These two miRNAs have been confirmed to be abundant in liver and to participate in fat metabolism [43–47]. The ssc-miR-122 plays an important role in lipid metabolism [48]. It has been reported that ssc-miR-122 is a liver-specific miRNA, and it is expressed almost exclusively in the liver [49,50]. In addition, ssc-mir-122 has been identified as a candidate miRNA of average daily gain trait in pigs [51]. Thus, the high expression of mir-122 in the porcine liver might also play a role in regulating the FE. The functional investigation reveals that ssc-miR-192 can promote hepatic lipid accumulation [52]. It has also been demonstrated that miR-192 is abundant in the liver [53]. The KEGG pathway analysis of these two abundant liver miRNAs indicates that their predicted target genes are enriched in glucagon signaling pathway, glycolysis / gluconeogenesis, citrate cycle (TCA cycle), insulin signaling pathway, AMP-activated protein kinase (AMPK) signaling pathway, and biosynthesis of amino acids. Therefore, miRNAs with high abundance in the liver of porcine may be an important regulator for energy metabolism and lipid metabolism.
Lipid metabolism in liver tissue has been reported to affect FE in pigs [40,41]. Two miRNAs involved in lipid metabolism (ssc-miR-16 and miR-135a-5p) have been found to be DE in liver in high-FE vs. low-FE pigs comparison. The ssc-miR-16 (hsa-miR-15a-5p) was up-regulated in the liver of high-FE pigs. One previous study has reported that miR-15a participates in multiple physiological processes, including adipocyte differentiation and lipid accumulation [54]. Moreover, the miR-15a/16 has been found to be negatively correlated with trglyceride and total cholesterol in liver tissue of pigs [55]. The ectopic overexpression of miR-15a strongly up-regulates the expression level of FASN mRNA, and this FASN mRNA has been found to be up-regulated in liver of high-FE pigs relative to low-FE pigs [56,57]. LAMP, a predicted target gene of miR-15a, has been found to be down–regulated in liver of high-FE pigs compared with that in low-FE pigs [32]. LAMP3 can regulate lipid metabolism of liver [58]. It should be noted that miR-15a has been reported to be associated with FE in bovine [17,59]. The miR-135a-5p, which can suppress adipogenesis by activating canonical Wnt/β-catenin signaling, is up-regulated in the liver of high-FE pigs, relative to low-FE pigs [60,61]. The KEGG pathway analysis indicates that the predicted target genes of miR-135a-5p are mainly enriched in thyroid hormone signaling pathway, insulin secretion, and cAMP signaling pathway. In addition, ELOVL7, a predicted target gene of miR-135a-5p, is down-regulated in liver of high-FE pigs. ELOVL7 is a key enzyme gene responsible for polyunsaturated fatty acid (PUFA) synthesis, and this gene has been reported to be associated with FE [62–65].
The miR-130a plays a key role in the fine-tuning of liver metabolic processes, and its expression is significantly up-regulated in the livers of high-FE pigs. It’s has been reported that miR-130a can inhibit lipid accumulation by down-regulating FASN, and both RNA-seq and qRT-PCR data indicate this gene is up-regulated in liver of high-FE pigs [66,67]. The miR-24 has been identified to be upregulated in liver of High-FE pigs, and knockdown of miR-24 results in the reduced hepatic lipid accumulation and the decreased plasma triglycerides [68]. In addition, miR-125b, miR-185, and miR-26a have been reported to participate in the lipid accumulation in liver [69–72].
Previous studies have shown that the DEGs between high-FE and low-FE pigs were significantly enriched in “carbohydrate metabolism” and “uptake and conversion of carbohydrates” [41]. In our study, the target genes of miR-135a-5p, miR-29a-3p, miR-15a-5p, miR-96-5p, miR-155-5p, miR-26a-5p, miR-185-5p, and miR-125b-5p were enriched in the GO terms of carbohydrate digestion and absorption. Sufficient evidence indicates that miRNAs (miR-135a, miR-29a, miR-15a, and miR-96) participate in glucose metabolism and glucose transporter 4 (GLUT4) pathway which plays a crucial role in insulin resistance and is closely associated with type 2 diabetes mellitus (T2DM) [73–75]. The miR-29a can decrease fasting blood glucose levels by negatively regulating hepatic gluconeogenesis and inhibit insulin-stimulated glucose transport in adipocytes [76,77]. The miR-155 can positively regulate glucose uptake and glycolysis [78]. The miR-26a can regulate insulin signaling and metabolism of glucose and lipids [79]. The miR-185 in mice and diabetic patients is significantly downregulated, and this miRNA is associated with blood glucose [80]. The miR-125b can decrease glucose uptake and inhibit insulin signaling pathway [81–83].
Metabolism of xenobiotics by cytochrome P450 and butanoate and tryptophan Metabolism have been found to influence FE [42]. Cytochrome P450 can regulate synthesis of lipids, steroids, and hormones, and the members of cytochrome P450 family have been found (CYP1A1, CYP2J2, CYP26A1) to be DE in liver of high-FE and low-FE pigs [32,84,85]. Butanoate is a dietary fiber metabolite and it is closely related to energy metabolism [86]. In this study, the target genes of miR-185, miR-29a, miR-135a, miR-130a, miR-125b, miR-26a, miR-15a, and miR-96 were enriched in metabolism pathways of xenobiotics by cytochrome P450; the target genes of miR-26a, miR-96, miR-155, miR-125b, and miR-24 were enriched in butanoate metabolism pathway; and the target genes of miR-130a, miR-26a, miR-96, miR-15a, miR-185, and miR-24 were enriched in tryptophan metabolism pathway.
CONCLUSION
Overall, a total of 212–221 known porcine miRNAs and 136–281 novel miRNAs were identified. The 14 miRNAs were identified to be significantly DE in the comparison of high-FE vs. low-FE pig liver, of which 12 miRNAs were homologous to human miRNAs. The KEGG pathway enrichment analysis indicated that these DE miRNAs might influence FE by regulating the pathways related to lipid metabolism, carbohydrate digestion and absorption, metabolism of xenobiotics by cytochrome P450, butanoate and tryptophan Metabolism. Our findings provide an insight into the role of miRNAs in the regulation of pig FE.