Introduction
Small antral follicles represent the fundamental developmental unit of the mammalian ovary and, as such, serve the needs of the entire reproductive life span. After pubertal, cohort of small antral follicles enters to gonadotrophin-sensitive development upon FSH (Follicle Stimulating Hormone) stimulation, called cyclic recruited follicles [1, 2]. Cyclic recruitment, although obligatory, does not guarantee ovulation because growing follicles are vulnerable to atresia and thus may fall out from the growth trajectory [3]. Among somatic cells of the antral follicles, granulosa cells will undergo serious changes morphologically and physiologically during the processes of proliferation, differentiation, ovulation, lutenization and atresia [4].
To understand gene function of the cellular processes, genes must be studied in the context of networks. Emerging tools from systems biology going beyond simple gene ontology (GO) terms like causal network modelling linking gene expression analysis to gene interaction information are sorely needed [5, 6]. Nowadays, biological networks allow a comprehensive examination of the complex mechanisms for targeted empirical studies [7]. Numerous studies have been conducted in attempt to identify candidate genes of reproductive biology via gene networks analysis such as development of rat primordial follicles [8, 9], early embryo development in mouse [10] and bovine [11], gene networks of bovine oocytes [12] and bovine granulosa cells of small and large follicles [13]. Protein-protein interaction (PPI) networks are one the most known approaches for representation of candidate genes/proteins beyond of high-throughput studies [13–19].
In previous study, we surprisingly have shown differences in transcriptome profiles of ovine (sheep) granulosa cells between small antral follicles (1–3 mm) collected during the follicular and luteal phases [20]. Therefore, the specific purpose of the present study was to survey the differentially expressed genes (DEGs) from the previous study using the analysis of PPI networks in order to identification of candidate genes that probably those are impressive in cyclic recruitment of ovarian follicles.
Materials and methods
The main dataset of the present study was belonged to 663 DEGs in ovine granulosa cells between small antral follicles (1–3 mm in diameter) collected during the follicular and luteal phases [20]. These DEGs afterwards were annotated using a standard Ensembl gene annotation system.
A large PPI network was reconstructed from the 646 genes/protein symbols (Additional file 1) of the Ensembl annotation (Ovis_aries.Oar_v3.1.91). In order to map pairwise interactions, all computational methods in STRING database Version. 10.0 [21] containing neighborhoods, gene fusion, co-occurrence, homology, co-expression, experiments, databases and text mining, were utilized with the medium confidence score (> 0.4).
Cytoscape Version 3.1.1 [22] was used to plot and analyze the centralities, clustering and modularity of the PPI network. The MCODE (Molecular Complex Detection) v1.4.0-beta2 was performed to screen modules of PPI network with degree cutoff = 2, node score cutoff = 0.2, k-core = 2, and max. Depth = 100 [14]. This is a well-known automated algorithm to find highly interconnected subgraphs that detects densely connected regions in large PPI networks that may represent molecular complexes [23]. Also, the functional modules were chosen with the number of nodes ≥16 and nodes score [19].
Biological significance of these predicted modules were inferred by ClueGO [24] plugin of Cytoscape. The statistical significance of the biological terms analyzed was calculated with Right-sided enrichment hypergeometric test and Benjamini and Hochberg P-value correction [25] to reduce false positives- and negatives. Kappa statistics were used to link and grouping of the enriched terms and functional grouping of them as described by Bindea et al. [24]. The minimum connectivity of the pathway network (kappa score) was set to 0.4 units.
In this study, all networks were utilized to identify hub genes/proteins which important in folliculogenesis during the ovine estrous cycle. Hubs were detected by calculating the node degree distribution [16] using the Network Analyzer (http://apps.cytoscape.org/apps/networkanalyzer) plugin of Cytoscape [24]. Degree gives a simple count of the number of interactions of a given node [26]. Additionally, we utilized several centrality parameters including stress, betweenness and closeness instead of using degree centrality itself. The mathematical formulas for the calculation of centrality parameters including stress, betweenes and clossness, are available in Additional file 2 that has been retrieved from Zhuang et al. [26]. By extract direction of PPI from STRING Version. 10.0 [21], a directed gene network was reconstructed from the hub genes/proteins. These important proteins were extracted from hub genes/proteins via network analysis and modularity of the network. This small, but important, graph is visualized by Cytoscape Version 3.1.1 [22]. Using CluePedia v.1.1.7. [24] plugin of Cytoscape, hub genes/proteins were placed in a directed gene network based on its molecular function.
Result and discussion
Among 646 DEGs (Additional file 1), 498 proteins were annotated on the Total PPI network. Also, 2191 edges containing neighborhoods, gene fusion, co-occurrence, homology, co-expression, experiments, databases and text mining, were interacted between such genes/proteins (Fig. 1 and Additional file 3). The statistics of network containing network density, network diameter and clustering coefficient were 0.018, 9 and 0.275, respectively. The power law of degree distribution was followed with an R2 = 0.895. Meanwhile, R2 is computed on logarithmized values. Some proteins in this network had high values in all degree, stress and betweenness centralities, such as FYN, CDK1, RAC1, ACTG2, FGR, APP, MMP2, SYK, CDH1, TGFB1, PTPN6, ITGB7, FOS, ACTN1, GNAI2, INSRR, BMP4, BMP2, LYN and HCK (Additional file 3). This list was utilized to identify some candidate genes among hubs as major regulators in cyclic recruitment of ovine small antral follicles.
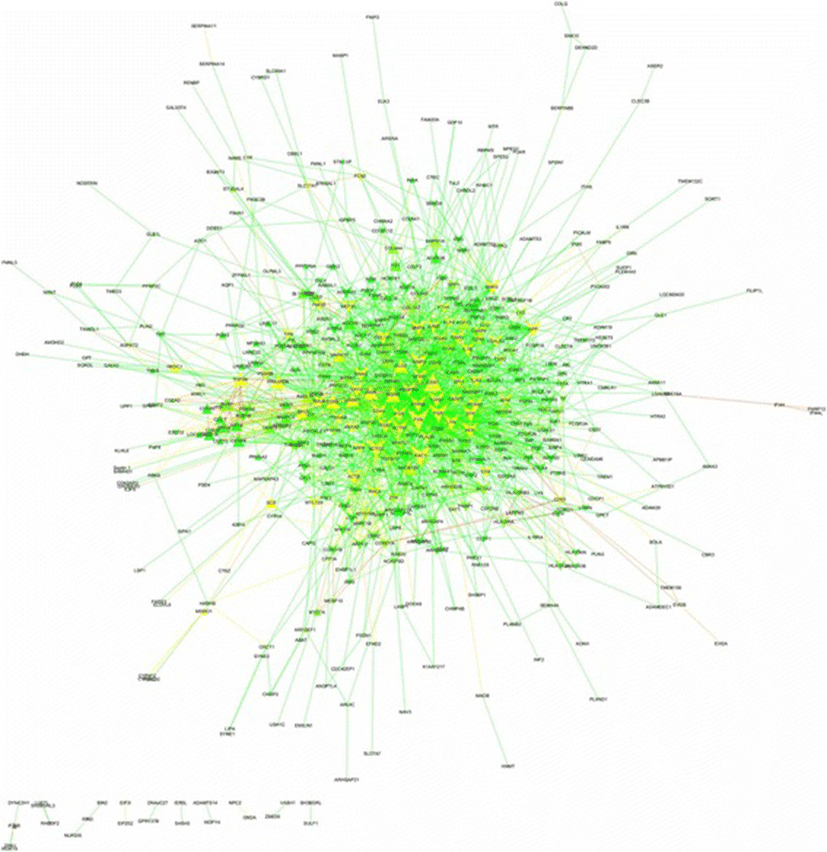
From the total PPI network, thirteen modules were extracted, among which five subnetworks (modules of 1, 2, 4, 6 and 12) were detected with the intra-connection nodes ≥16 and node score > 2.0 (Table 1). A module/subnetwork is a group of closely related proteins that act in concert to perform specific biological functions through PPI network that occurs in time and space [15]. In the biological processes (BP), the highest significant terms for module 1, module 2, module 4, module 6 and module 12 were related to the regulation of B cell receptor signaling pathway, Fc receptor mediated stimulatory signaling pathway, positive regulation of reactive oxygen species metabolic process, innate immune response activating cell surface receptor signaling pathway and positive regulation of DNA replication, respectively (Table 2). As opposite performances among these modules, we have surprisingly identified four modules of 1, 2, 4 and 6, in relevant to immune system in comparison with module 12 in relevant to cell proliferation (Table 2).
Abbreviations: PPI Protein–protein interaction, DEGs Differentially expressed genes
Abbreviations:BP Biological process, PPI Protein–protein interaction
Among identified hub genes/proteins in Table 3, twenty-five hub genes (FYN, RAC1, FGR, MMP2, SYK, LYN, TGFB1, PTPN6, FOS, CDH1, ITGB7, HCK, APP, ACTN1, GNAI2, BMP4, BMP2, PTPRC, NFKB1, VAV1, IL1B, COL1A1, TIMP1, PDGFRA, BTK), were up-regulated in ovine granulosa cells of small antral follicles during the follicular phase (up-regulated genes in Follicular has been shown in Additional file 1). Interestingly, these hubs were significantly connected to immune system and phagocytosis (Table 2). On the contrary, three (CDK1, INSRR and TOP2A) were up-regulated in ovine granulosa cells of small antral follicles during the luteal phase (up-regulated genes in Luteal has been shown in Additional file 1).
Abbreviations:PPI Protein–protein interaction
As shown in Fig. 2, the hub genes of PTPN6 and FYN are revealed the highest in-degree and out-degree, respectively (Additional file 4, and Fig. 2). Regarding to protein differential expression in normal tissues from HIPED (the Human Integrated Protein Expression Database), PTPN6 is overexpressed in Peripheral blood mononuclear cells, Lymph node, Blymphocyte, and Monocytes (http://www.genecards.org/). Moreover, FYN is another hub with highest out-degree, is also overexpressed in Peripheral blood mononuclear cells (http://www.genecards.org/). Regardless, PTPN6 and FYN being up-regulated in the ovine granulosa cells of small antral follicles during the follicular phase, represents an accumulation of blood immune cells into small antral follicles of the follicular phase in comparison with luteal phase. Therefore, the protein of FYN can be known as an upstream regulator in inhibition of ovarian folliculogenesis. This protein is belonged to the protein tyrosine kinase (PTK) family as illustrated in regulatory model of molecular function in Fig. 2. These signaling molecules regulate a variety of cellular processes including cell growth, differentiation, mitotic cycle, and oncogenic transformation (http://www.genecards.org/).
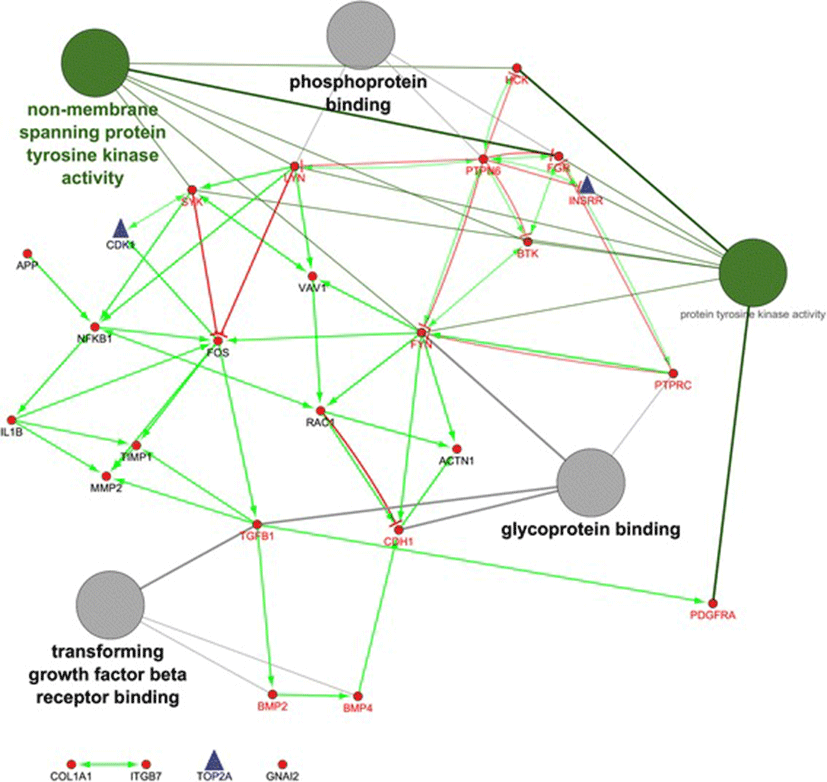
As shown in Table 2, the subnetwork 2 with the eight hubs were became the highest in relative to the others. The most significant term of BP in module 2 was belonged to Fc receptor mediated stimulatory signaling for the hub genes/proteins, including FYN, HCK, RAC1, SYK and VAV1 (Table 2). This can regulate immune responses through interacting with Fc receptors [27]. Moreover, the up-regulated hubs of PDGFRA, COL1A1, BMP2, CDH1, ACTN1, FOS, FYN, and TIMP1 in the follicular phase, they also were up-regulated in bovine and porcine granulosa cells of small atretic follicles [28, 29]. By contrast, the up-regulated hubs of CDK1 and TOP2A in the luteal phase, they also were up-regulated in the bovine granulosa cells of small heathy follicles [28]. These genes were cohesively interconnected around up-regulated nodes in classical mitosis checkpoint controllers [30]. Furthermore, TOP2A is expressed mainly during the S to G2 /M phase of the cell cycle and is likely to play a major role in DNA catenation during mitosis [31]. Interestingly, such hubs all were belonged to module 12 whose been enriched in positive regulation of DNA, cell cycle and progesterone-mediated oocyte (Table 2). Therefore, the hubs including CDK1, INSRR and TOP2A, represented the proliferation of ovine granulosa cells from small antral follicles during the luteal phase [20], whose probably are crucial in cyclic recruitment of small antral follicles. This is closer to the second theory in recruitment of antral follicles during menstrual cycle by Baerwald et al. [32].
Conclusion
In this study, we evidenced that cyclic recruitment of small antral follicles mostly occurs in the luteal phase in comparison with follicular phase during the ovine estrous cycle. Based on analysis of PPI network and its modulation, we identified some biomarkers whose potentially impress on cyclic recruitment of ovarian follicles. Surprisingly, FYN was identified as upstream regulator that probably inhibits the proliferation of granulosa cells. By contrast, hub genes of CDK1, INSRR and TOP2A, were known as inducers in proliferation of granulosa cells among genes were up-regulated in luteal phase in comparison with follicular phase. These results may provide valuable genetic markers for increasing ewe prolificacy with focus on cyclic recruitment of ovarian small follicles. Nevertheless, further studies using an experimental approach and a greater number of individuals are warranted for the verification of such candidate genes.