INTRODUCTION
Various stress factors such as environmental and nutritional stresses as well as weaning affect animal productivity and health. In the case of piglets with reduced digestive capacity, protein is not digested by salmonella in the gastrointestinal tract and fermentation of these proteins proceeds, which generate branch chain fatty acids and ammonia nitrogen. These products are toxic metabolites of the intestinal mucosa that damage the intestinal mucosa and cause diarrhea. This usually decreases villus height and digestive enzyme activity during the first few days after weaning [1]. Weaning stress is the main cause of economic loss to pig farmers. In addition, salmonella-infected pigs entering the human food chain is a cause of concern in terms of food safety. To address this concern, it is desirable to eliminate the problem at the weaning step [2].
Salmonella enterica serovar Typhimurium is widely isolated serotype of S. enterica in pigs. S. typhimurium isolate HJL777 is a strong, virulent strain in mice [3]. In pigs, the disease is susceptible to all ages, but it is most common in weaned pigs. The common clinical symptom in pigs with salmonella-infected is enterocolitis. Due to infected pigs can shed salmonella for at least 28 weeks, these pigs can become salmonella storages, which can be a potential factor for infecting other animals. S. typhimurium is highly contagious and can spread dramatically within pig populations once infected [4]. Piglets with salmonella-infected can cause significant economic losses to pig farmer.
Intestinal microbial communities associate with the overall development and metabolic of animals. In addition, the intestinal microbiome is a vital stimulus in the development of the immune system in pigs. The microbiome changes by many factors such as antibiotics, stress and diet [5]. The 16s rRNA gene sequences of intestinal microbes have been used to determine taxonomic identities. Additionally, when the production of 16s rRNA gene sequences is combined with high throughput DNA sequencing, it provides a means of describing microbial presence in various communities. Furthermore, Freeman et al. [6] provides a detailed atlas of gene expression in a variety of pig tissues through transcriptome analysis using RNA sequencing, with particular emphasis on the intestines. However, research into gene expression in pig intestines using RNA sequencing is still lacking.
Various methods to reduce or eliminate salmonella infection in young pigs have been used, such as vaccination, competitive exclusion, feed and water treatments, antibiotic administration, disinfection of animals, and segregated weaning to clean accommodations [2]. However, when weaned pigs are infected with salmonella, little is known about the exact effects of salmonella on the intestinal microbiome, gene expression patterns, and biological function based on genomics. In this study, we aimed to identify the change in gut microbiota and biological function of piglets when they were infected with salmonella. This was accomplished by analyzing the fecal metagenome and intestinal transcriptome using 16S rRNA and RNA sequencing. Many studies have researched the metagenomics features of gut microbial using feces and the role of the small intestine, which is primarily involved in the host’s immune system [7]. Our studies will help to prevent salmonella infection in young pigs. Furthermore, these studies will support disease prevention and improve productivity in the swine industry.
MATERIALS AND METHODS
In this study, we used six female three-way crossbred (Landrace × Yorkshire × Duroc) piglets. All animal experiments were performed with in accordance with national and university guidelines. The animal protocol reported in this study was approved by the Chonbuk National University Animal Ethics Committee in accordance with the guidelines of the Korean Council on Animal care (CBNU 2015-029). We randomly assigned weaned piglets at 4 weeks after birth to the control and treatment groups (n = 3). Each group was housed in an environmentally controlled separate room to prevent cross-contamination between groups in the experimental swine unit of the College of Veterinary Medicine at Jeonbuk National University. Each room was equipped with a ventilation fan and separate air-conditioning system. Piglets were fed ad libitum with fresh water and weaning food (FARMSCO, Anseong, Korea) for the entire duration of the experiment. The information of experimental animals in the experiment is the same as our previous paper.
The wild type S. typhimurium isolate HJL777 was used as a challenge strain, as described by a previous study [8]. After 1 week allowing for adaptation, 3 piglets in the infected group were orally challenged with 5×10E9 CFU) of HJL777 in 2 mL of sterile phosphate-buffered saline (PBS)-sucrose. All piglets were monitored daily for diarrhea during the 4-week post-challenge period. Induction of diarrhea by the challenge strain was confirmed by isolating the challenge strain from the rectal swab of a piglet with diarrhea [9].
For immunoglobulin G (IgG) measurements, we collected blood samples from piglets on days 0, 14 and 28 during the 4-week trial. Whole blood samples were collected from the jugular vein of pigs using a serum collection tubes (BD Vacutainer SSTTM II Advance, Becton Dickinson, Plymouth, UK), and serum was obtained by centrifuging at 2500×g for 20 minutes within 3 hours of collection. Serum was stored at –20°C for subsequent analysis of IgG. Standard ELISA was performed using a pig IgG ELISA quantification kit according to the manufacturer’s instructions (Bethyl Lab, Montgomery, TX, USA) to determine the IgG concentration in the serum.
At the end of the animal experiment, all piglets were euthanized by intramuscular administration of ZoletilTM 50 (Virbac; 7–10 mg/kg of body weight; Carros, Cedex, France) and xylazine (2.32–3.48 mg/kg; Bayer Korea, Ansan, Korea). Rectal fecal samples were collected from each piglet and transferred to sterile tubes, and the samples were immediately frozen on dry ice. For subsequent isolation of fecal microbial DNA, it was stored at -80°C. The small intestine was removed from the cavity and divided into 10 equal length portions. Each distal portion of the small intestine (segment 8) was transferred to a sterile tube and immediately frozen on dry ice and stored at −80°C for subsequent RNA isolation.
The small intestine (duodenum, jejunum, ileum) from piglets was collected and fixed with 10% neutral buffered formalin (NBF). Paraffin-embedded tissue blocks were cut to 5 μm. The excised samples were deparaffinized in xylene, hydrated with a series of ethanol, and then stained with hematoxylin and eosin (HE) to analyze the histological composition of the tissues. Stained sections were dehydrated in ascending ethanol, removed from xylene, and mounted on slides. Digital images were taken at a fixed 100× magnification using a Leica DM2500 microscope (Leica Microsystems, Wetzlar, Germany).
A 10 g rectal stool sample was aseptically removed from a sterile tube and placed in a Whirl-Pak® bag (Nasco, Fort Atkinson, WI) containing 90 mL of 0.1% peptone water. Samples were placed in the stomach for 2 minutes and serially diluted. The diluted samples were then plated on de Man, Rogosa & Sharpe (MRS) plates containing 0.05% (w/v) bromocresol purple (BCP). The plates were then incubated anaerobically at 37°C for 48 h. Counts were recorded in colony forming units per gram (CFU/g).
The Epicenter DNA Isolation Kit was used to isolate three biologically replicated DNA from each dietary group and extract approximately 900 ng of DNA from each sample. DNA quality was checked with the Bioanalyzer using the Agilent RNA 6000 Pico Kit (Agilent, Santa Clara, CA, USA). To sample the V3-V4 variable region of the 16S rRNA gene, all samples in the reservoir were prepared using the 16S library preparation protocol and the Nextera XT DNA index kit (Illumina, San Diego, CA, USA). Quantification of the library was measured using a CFX96 real-time polymerase chain reaction (PCR) system (BioRad, Hercules, CA, USA). Samples were then loaded into the MiSeq reagent cartridge (Illumina) and instrument. This loading resulted in automated cluster generation and 2 × 300 base pair (bp) paired-end sequencing.
Using the MiSeq output, the Quantitative Insights Into Microbial Ecology (QIIME) (version 1.9.1) [10] generates useful information about the microbial community in each sample. We performed the upstream and downstream analysis. In the upstream analysis, we merged paired-end reads and then conducted demultiplexing of the merged reads. As we performed this step, we applied --barcode_type ‘not-barcoded’ option because the barcode sequence has already been removed from our sequence data. Some of the chimeric sequences could be generated from multiple parent sequences in PCR amplification step and confirmed chimeras in our fasta files using the GREENGENES database [11] and vsearch (2.4.4 version) [12]. And then, we filtered out the identified chimera sequences from the fasta files. The filtered sequences were clustered into Operational Taxonomic Units (OTUs) to produce groups of organisms defined by unique phenotypic similarities that establish candidate taxa [13,14]. The OTUs were calculated based on sequence identity. If the sequence was more similar than the user-defined identity threshold, the sequence represented as a percentage is clustered together. The threshold at this level was set to 97% of sequence similarity, which was traditionally estimated to represent bacterial species [15]. The OUT picking was performed with open-reference OTU picking method. Reads were clustered in correspondence with the reference sequence, and the remaining reads that did not match the reference sequence were clustered in de novo method [16]. In the downstream analysis, we carried out diversity (Shannon and Simpson) and richness (Observed, Chao1, and ACE) analysis in control and salmonella-infected groups [17]. We compared microbial communities based on compositional structures. Using QIIME and Student’s t-test, we conducted unweighted and weighted UniFrac analysis in order to extract multi-level taxonomic abundance and to compare relative abundance and identify differentially abundant microbes in the control and salmonella-infected groups. To take into account different read productions, we used percentages instead of read counts. We performed the calculation of species richness for a given number of individual samples based on the construction of rarefaction curves using 3 scripts in QIIME [18]. The ‘multiple_rarefactions.py’ script was used to generate rarefied OUT tables, ‘alpha_diversity.py’ was used to compute alpha diversity metrics for each rarefied OUT table, and ‘collate_alpha.py’ was used to collate alpha diversity results. The detailed scripts and options related to aforementioned analysis were presented in our previous paper [19].
We predicted the functional-gene profile of microbial communities based on maker gene survey and database of reference genomes using Phylogenetic Investigation of Communities by Reconstruction of Unobserved States (PICRUSt) (1.1.4 version) [20]. We used ‘normalize_by_copy_number.py’ to normalize the OTU table by separating each OTU by the known and predicted 16S copy number abundance and then we used ‘predict_metagenomes.py’ to make the final metagenome functional prediction. The next step was collapsing table data to a specified level in a hierarchy using ‘categorize_by_function.py’. We used this script with ‘--level 2’ and ‘--level 3’ options. A value of 1 is the highest level. Using these results, we predicted taxonomic and functional profiles based on statistical analysis using STAMP (2.1.3 version) [21]. All unclassified reads were removed and we exhibited p-value greater than 0.05. At level 2 and level 3, effect size (difference between proportions) was less than 0.01 and 0.03, respectively. Categories are sorted by effect sizes calculated using the two-sided Welch’s t-test.
We isolated RNA from the ileum tissues using TRIzol reagent (Invitrogen) and identified total RNA quantity and quality using a NanoDrop1000 spectrophotometer (Thermo Scientific, Wilmington, DE, USA) and Bioanalyzer 2100 (Agilent Technologies, Palo Alto CA, USA). The mRNA from total RNA was converted into an appropriate template molecule library for subsequent cluster generation using reagents provided in the Illumina ® TruSeq™ RNA Sample Preparation Kit. Next, we formed a second strand to generate double-stranded cDNA for constructing the TruSeq library. Then, the short ds-cDNA fragment was ligated to a sequencing adapter and the appropriate fragment was separated by agarose gel electrophoresis. And then, the TruSeq RNA library constructed through PCR amplification and quantified using quantitative PCR (qPCR) based on the qPCR Quantification Protocol Guide. In addition, we used the Agilent Technologies 2100 Bioanalyzer (Agilent Technologies) to library validation. We carried out paired-end sequencing (read length: 101 bp, insert size: 150–180 bp) using the generated RNA library and the HiSeq™ NextSeq 500 platform (Illumina). We performed a commonly used RNA-seq pipeline to measure transcriptome levels based on the generated RNA-seq reads. First of all, we removed adapter and trimmed the reads to make clean reads using Trimmomatic (v 0.32). And then, these clean reads were mapped to the genome reference (Sscrofa 11.1) in Ensembl database using hisat2 (v 2.1.0). Finally, we predicted the count of uniquely mapped reads for each of the 25,880 annotated genes from 6 samples based on the Sus scrofa transfer format (GTF) using the featureCounts (v 2.0.0) in SUBREAD packages (v 1.6.0) [22]. The detailed options related to aforementioned analysis were presented in our previous paper.
To identify differentially expressed genes (DEGs) between the control and salmonella-infected groups, we used DESeq2 (1.10.1 version) in R packages [23]. Based on empirical Bayes shrinkage method variance and fold changes were estimated by modeling the number of reads according to a negative binomial distribution. The p-value was then used to evaluate statistical significance. To control the false positives in RNA-seq dataset, we adjusted these estimated p-values using the false discovery rate (FDR) method based on multiple testing. The significant DEGs were confirmed under criteria such as |log2 (fold change)| ≥ 1.5 and FDR ≤ 0.05. And then, we performed functional clustering and enrichment analysis based on the official gene symbols using overrepresentation enrichment analysis (ORA), gene ontology (biological process, cellular component, and molecular function) and Kyoto Encyclopedia of Genes and Genomes (KEGG) pathway database in WebGestalt [24]. Through these analysis, we predicted the functional groups by comparing transcriptome in the control and salmonella-infected groups (Fig. 1).
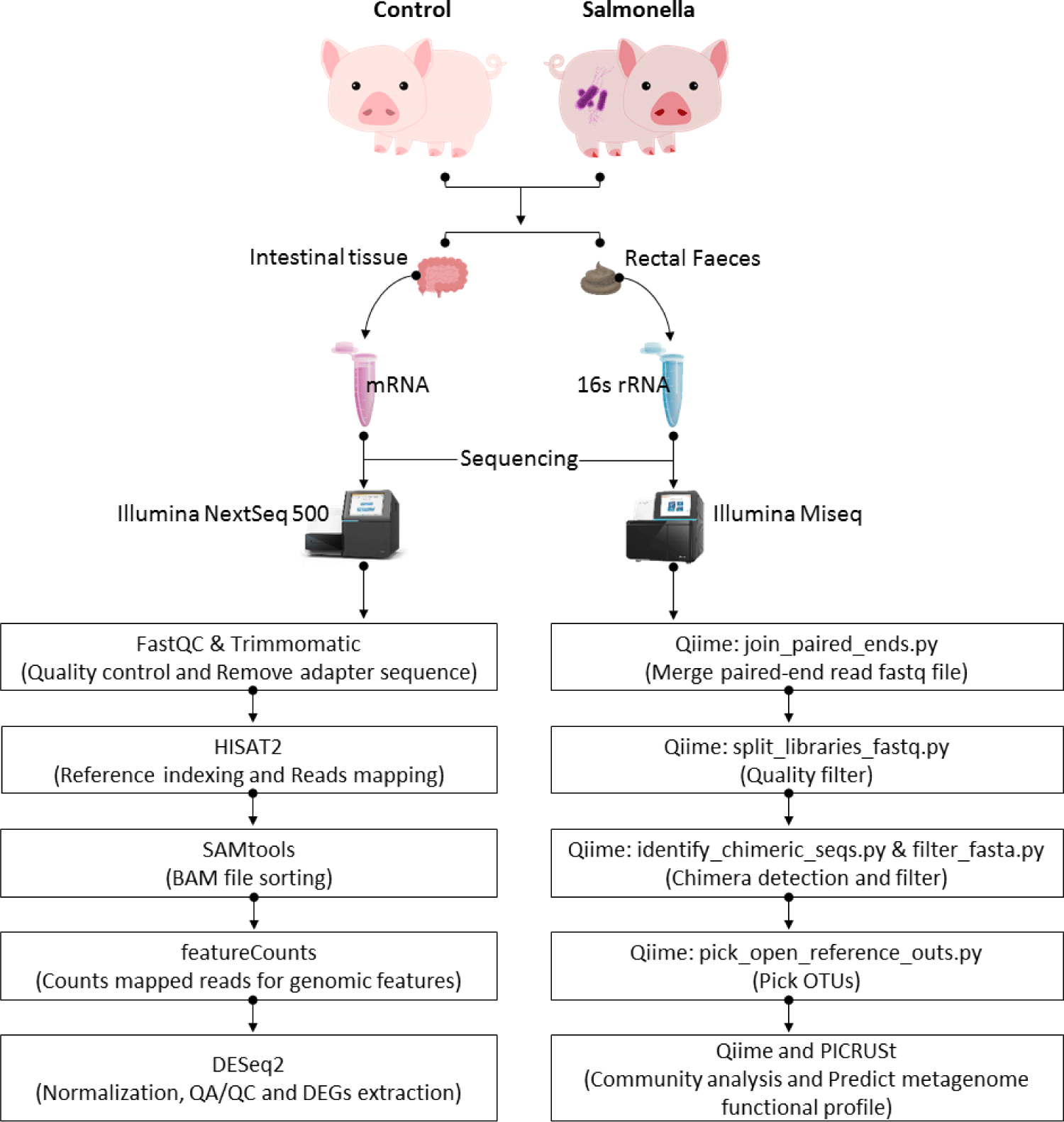
RESULTS
Piglets of the control group showed no clinical signs until 28 days after salmonella treatment, while diarrhea was observed from all piglets of the salmonella treatment group (Table 1). The number of lactic acid bacteria in fecal samples was significantly lower in the salmonella treatment group compared to the control at p < 0.05 (Fig. 2).
Group | Piglets | Diarrheic piglets | Piglets died by diarrhea |
---|---|---|---|
Control | 3 | 0 (0%) | 0 (0%) |
Salmonella1) | 3 | 3 (100%) | 0 (0%) |
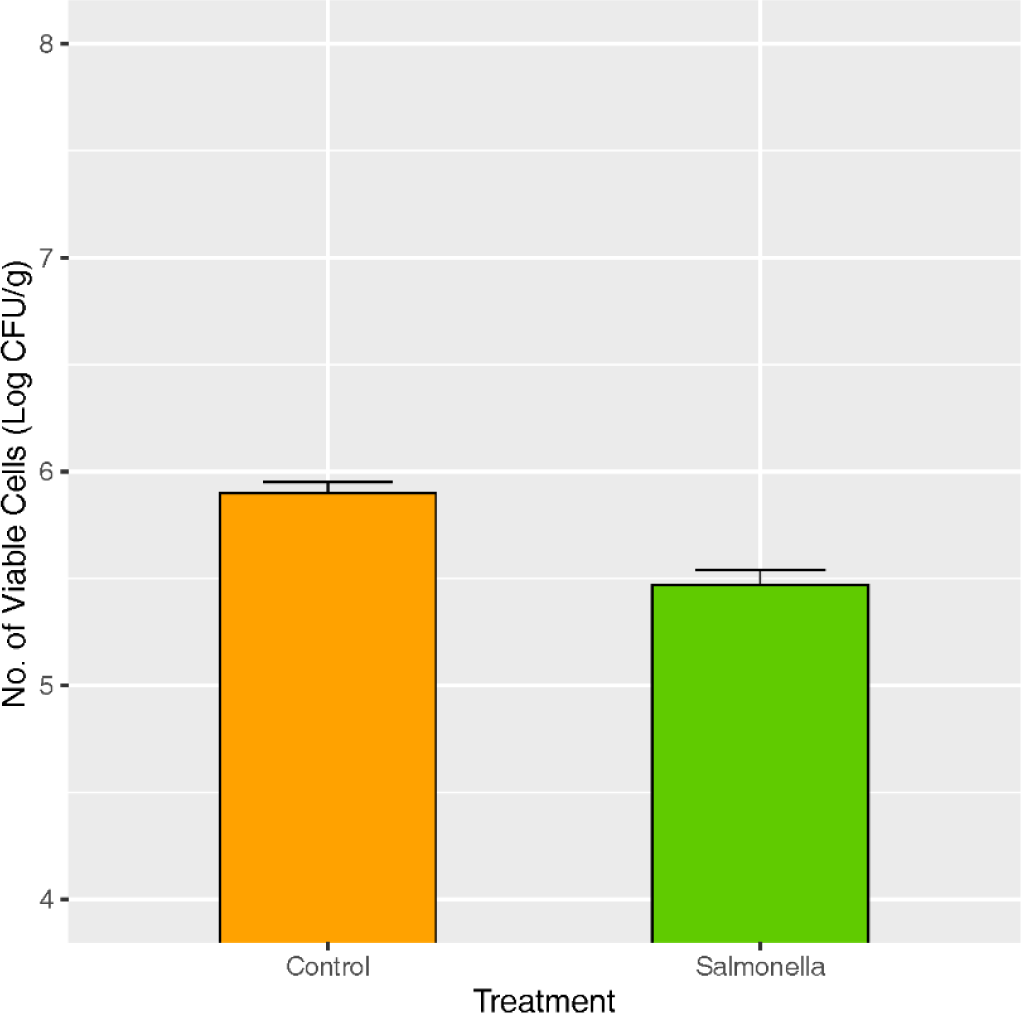
When piglets were infected with salmonella, photomicrographs of the epithelial layers of the small intestine, colon and cecum showed decreased intestinal villi height and crypt depth (Fig. 3). In addition, we found that the height of the villi was significantly reduced in the segments of the ileum, jejunum and duodenum.
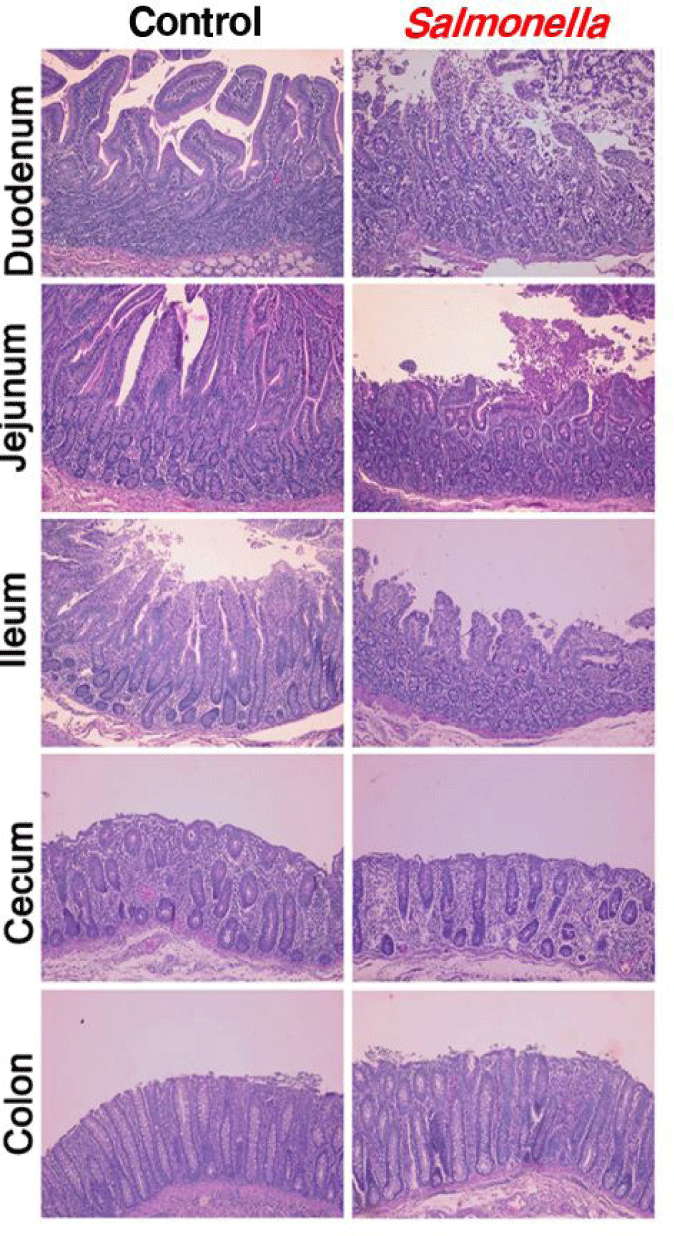
The salmonella-infected group had significantly higher number of microbial species than the control group (Figs. 4A, 4D, and 4E). The explicitly model evenness suggested that the salmonella-infected group had significantly higher microbial diversity than the control group (Figs. 4B and 4C).
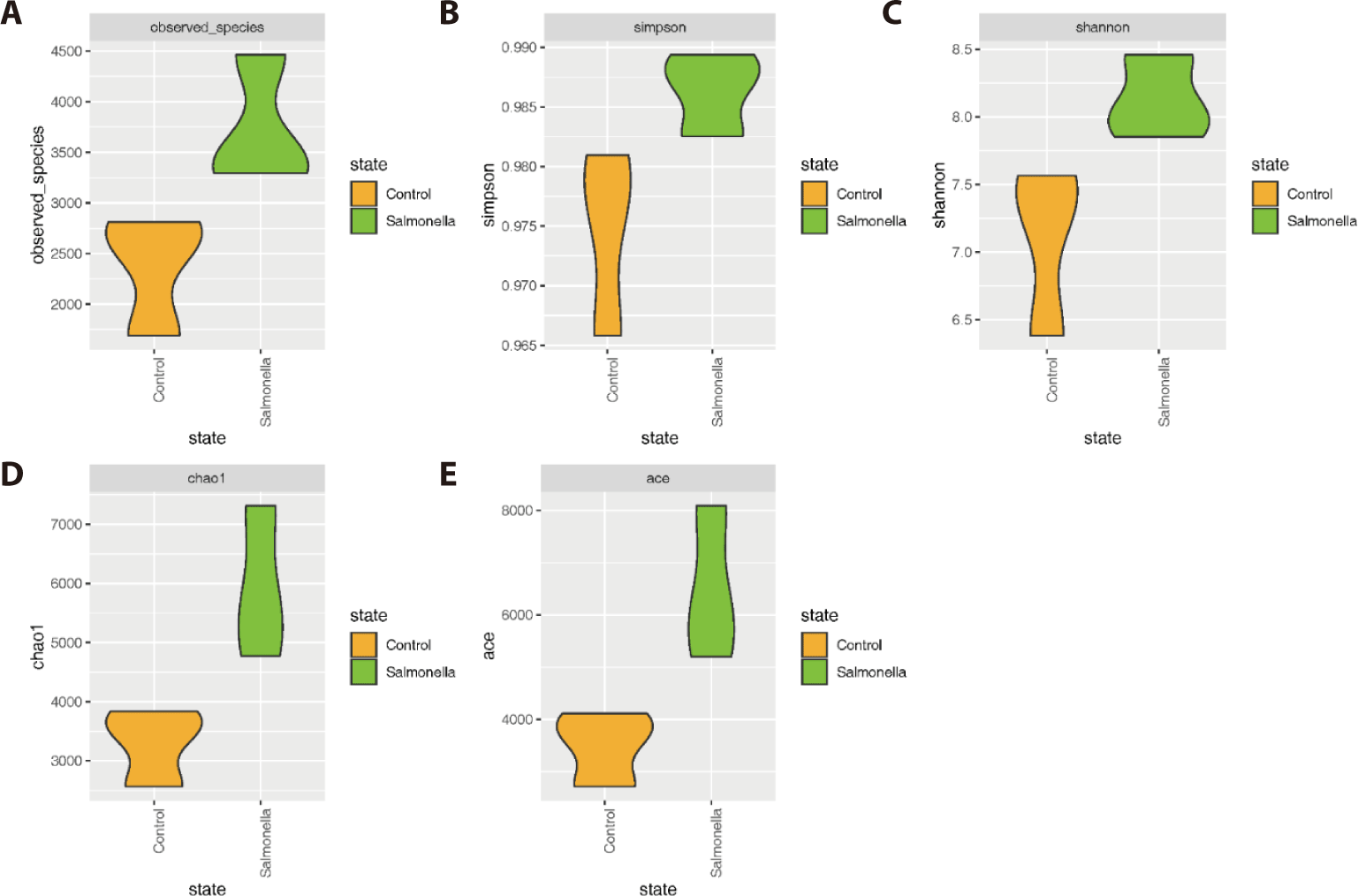
This clustering in the unweighted UniFrac analysis reveals the qualitative presence of salmonella bacteria in the fecal matter (or gut) of the salmonella-infected group. Also, there was similar distinct clustering of two groups on the weighted UniFrac, meaning that the salmonella-infected group tended to be separated from the control group microbiota (Fig. 5).
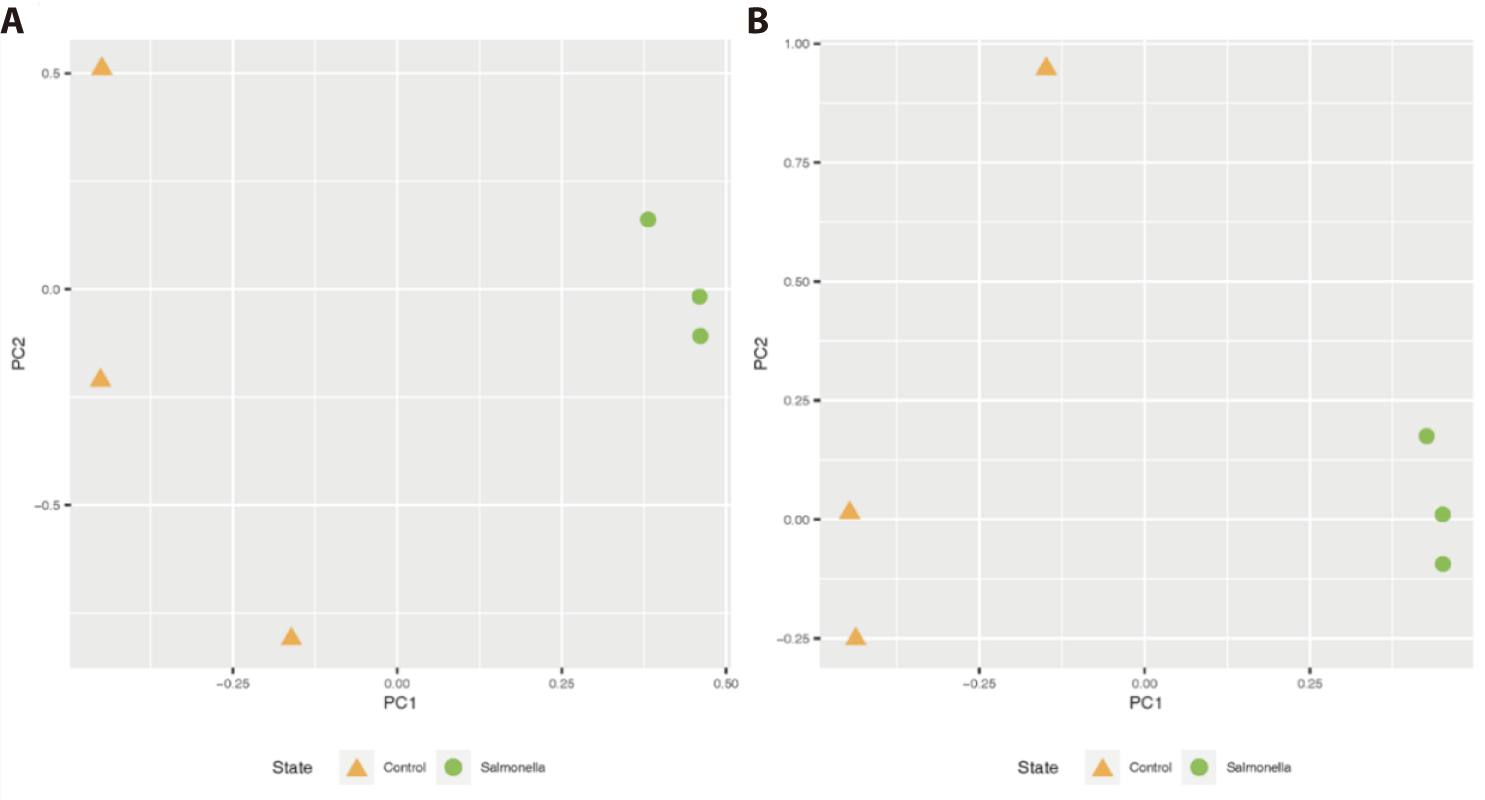
In the rarefaction curve figure, the number of OTUs in the salmonella-infected group was higher than in the control group (Fig. 6). Based on these result, it was hypothesized that salmonella caused changes in the intestinal microbiome, and thought that the difference between the two groups was clearly distinguished.
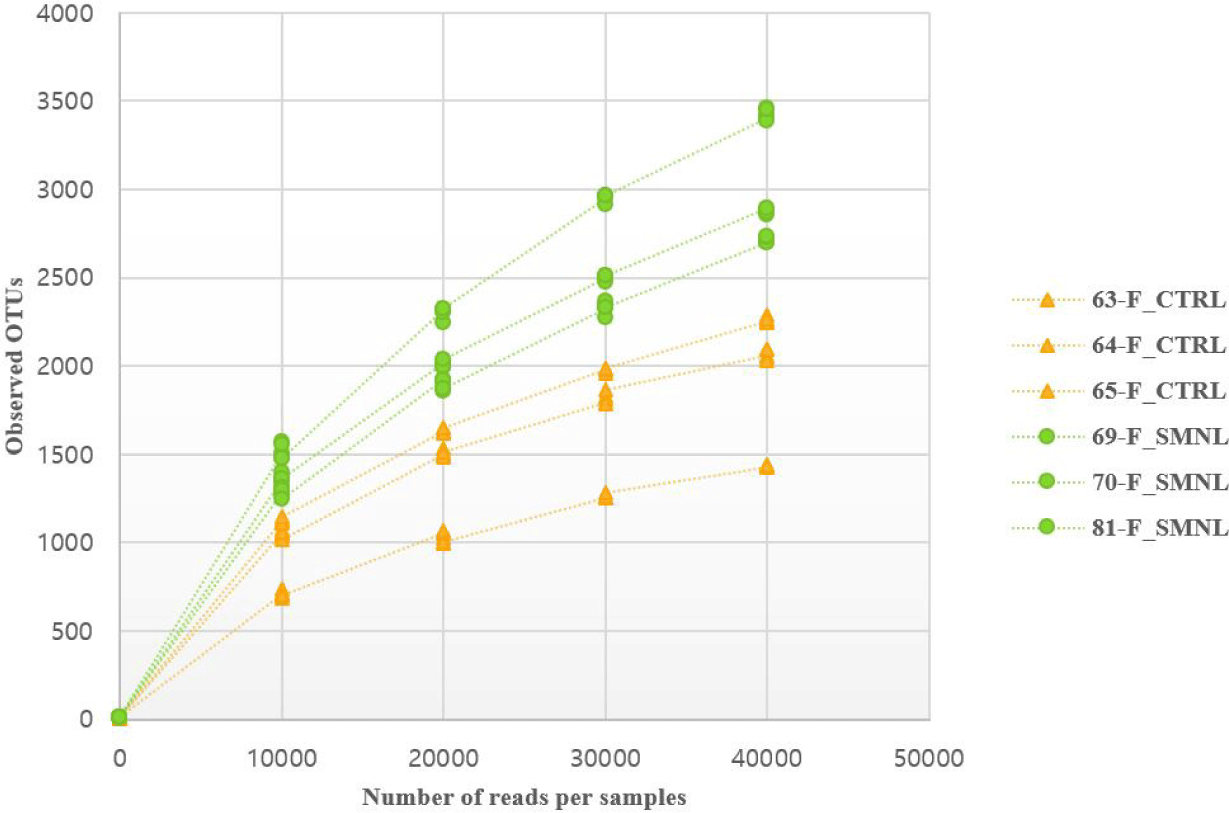
We confirmed the diversity of microbial composition and relative abundance at the phylum level in the fecal microbiome of piglets by 16S rRNA sequencing. Bacteroidetes and Firmicutes were two most abundant phyla in both groups. Also, Spirochaetes and Proteobacteria numbers were more increased in the salmonella-infected group than in the control group. The percentages of relative abundances of Bacteroidetes, Proteobacteria, Firmicutes, and Spirochaetes were significantly different at p-values 0.0001, 0.0093, 0.018, and 0.047 between the control and salmonella-infected groups, respectively (Fig. 7 and Table 2).
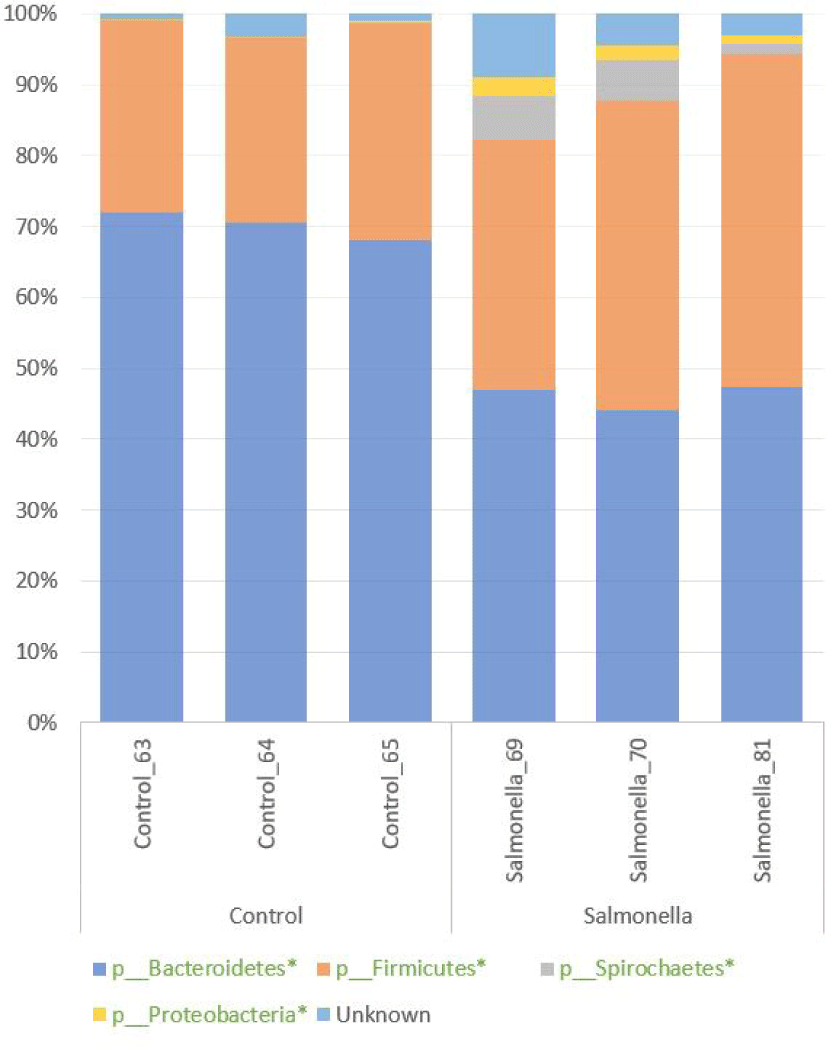
We observed that Bacteroidetes and Clostridia were the most abundant in both groups at class level of microbial taxonomy. Also, Spirochaetes and Erysipelotrichi numbers were more increased in the salmonella-infected group than in the control group. The relative abundances of the orders Bacteroidales and Spirochaetales between the two groups were significantly different with p-values of 0.0001 and 0.048, respectively (Fig. 8 and Table 3).
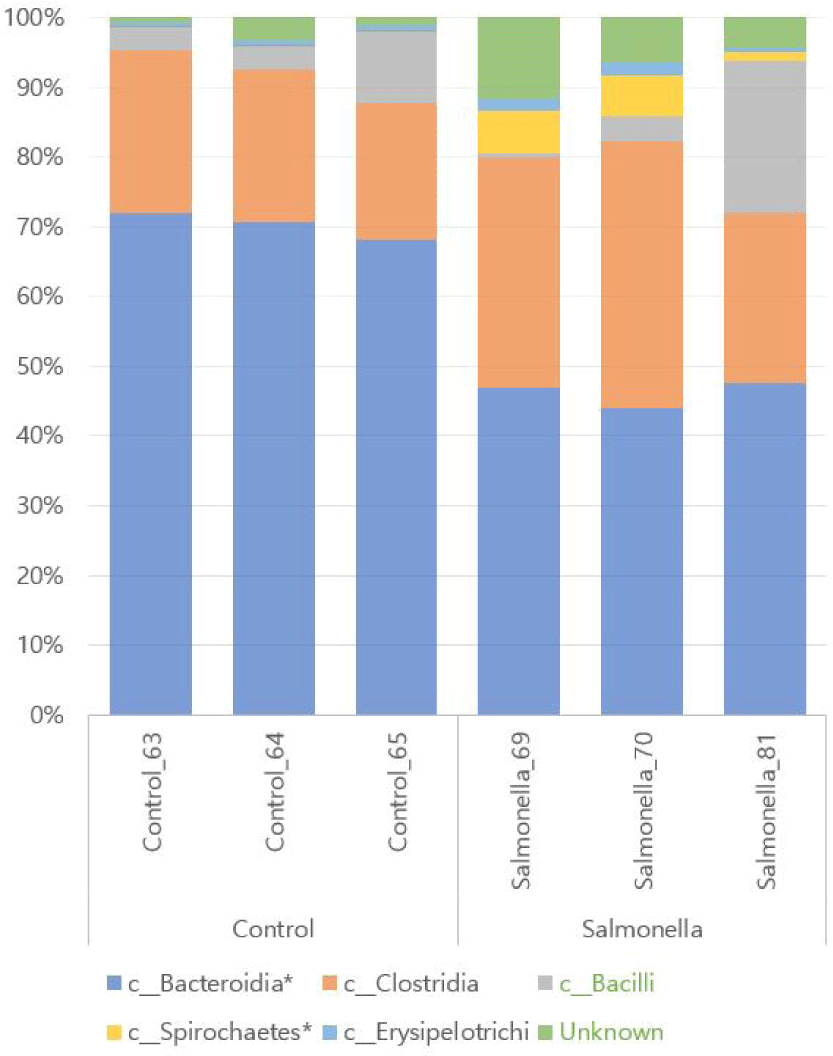
We confirmed that Bacteroidales and Clostridiales were the most abundant in both groups at order level of microbial taxonomy. Additionally, numbers of Spirochaetales and Erysipelotrichales were more increased in the salmonella-infected group than in the control group. The relative abundances of the orders Bacteroidales and Spirochaetales between two groups were significantly different with p-values of 0.0001 and 0.049, respectively (Fig. 9 and Table 4).
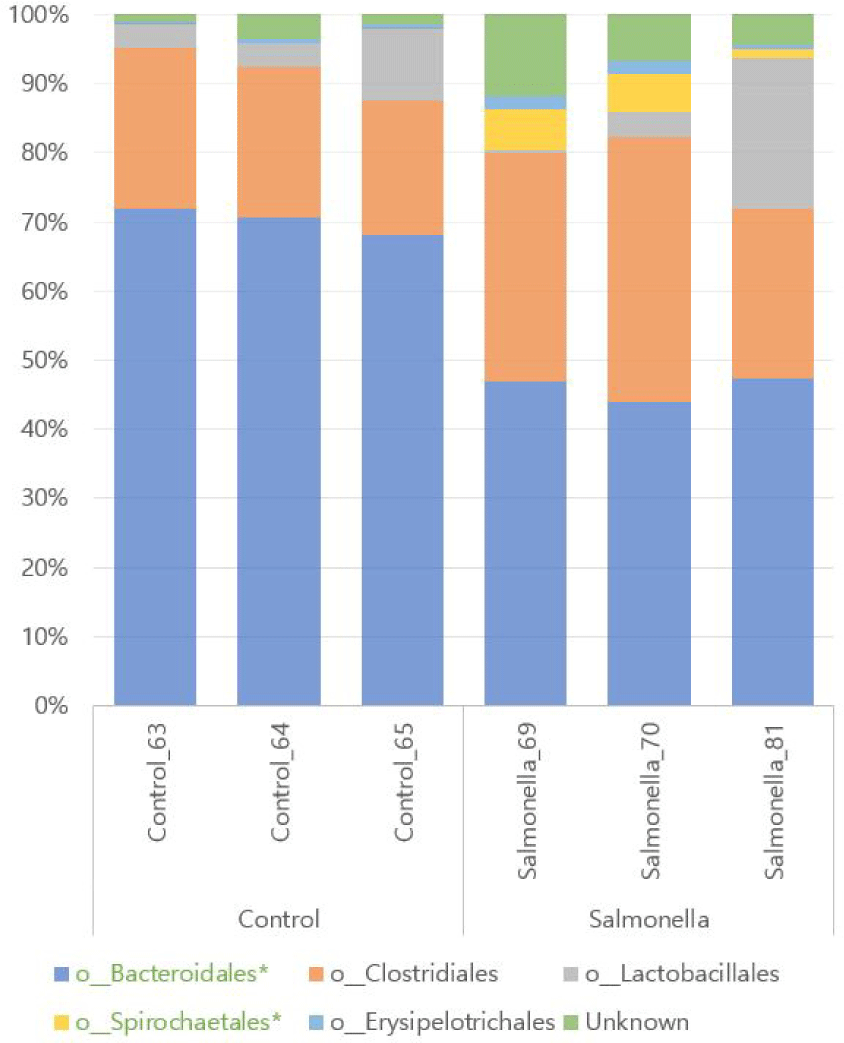
We found a total of 12 different microbial at family level of microbial taxonomy in two groups and Prevotellaceae and Ruminococcaceae were the most abundant. Also, Porphyromonadaceae, Spirochaetaceae, Clostridiaceae, Erysipelotrichaceae, and Christensenellaceae were increased in the salmonella-infected group. The abundances of families Prevotellaceae, Porphyromonadaceae, Clostridiaceae, and Spirochaetaceae were significantly different between the two groups (p < 0.05, Fig. 10 and Table 5).
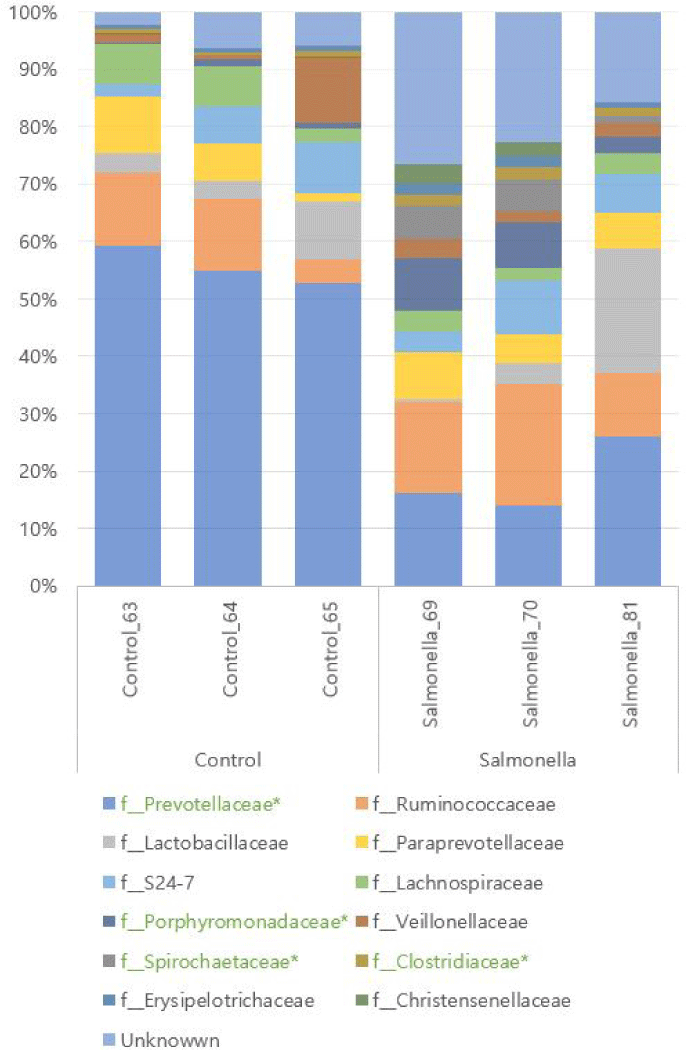
We identifed KEGG Orthology group (KOs) abundances based on 16S rRNA sequences using PICRUSt tool. As a result of analysis, 6908 Kos was predicted. Bray-Curtis compositional dissimilarity represented significant differences of predicted functions (p < 0.001) in the control and salmonella-infected groups based on OTU analysis. Further analyses focused on differences between the control and salmonella-infected groups. Analyses of functions at KO level 2 functions in these groups identified 11 differentially abundant KOs among the control and salmonella-infected groups (Fig. 11A). These functions include lipid metabolism, biosynthesis of other secondary metabolites, and immune system-related functions. The pathways of biosynthesis of other secondary metabolites and immune system-related functions were identified as having a slightly higher abundance in the control group. The pathway of lipid metabolism was identified as having higher abundance in the salmonella-infected group. Analyses of functions at level 3 in these groups identified 29 differentially abundant KOs among the control and salmonella-infected groups (Fig. 11B). The observed functions include butanoate metabolism, propanoate metabolism, and fatty acid metabolism, which all had higher abundance in the salmonella-infected group.
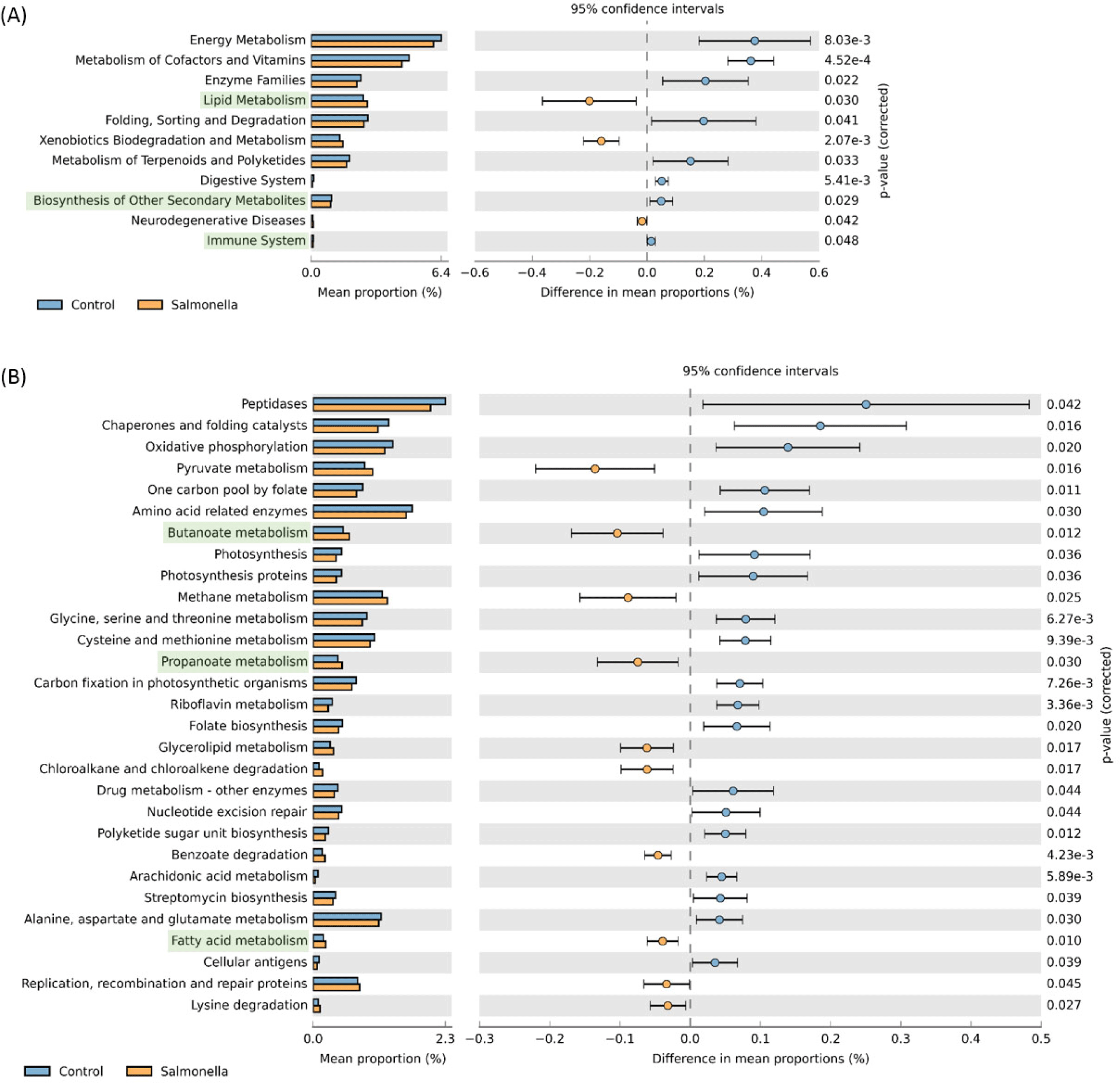
We generated RNA-seq reads from the small intestine of the control and salmonella-infected groups in piglets. Using the Trimmomatic tool, we found that the mean sequencing quality criteria passed in the control and salmonella-infected groups, respectively, were 94.61% and the average number of sequence reads was 15.8 million and 15.6 million. And then, we identified that most of mapping rate was over 96%, which could be considered to have been successfully mapped to the pig reference genome (Sscrofa11.1) (Table 6).
We observed that readcount data of host expression distinctly divided between the control and salmonella-infected groups through principal component analysis (PCA) analysis (Fig. 12A). We identified 31 DEGs in the small intestine of piglets using RNA-seq analysis (Table 7) and compared these DEGs to individual samples using heatmap visualization to confirm the expression pattern (Fig. 12B). In the salmonella-infected pigs, we identified 23 up-regulated expression genes and 8 down-regulated expression genes (Fig. 12C). These data suggests that salmonella bacteria causes a significant alteration of the small instestine gene expression pattern in weaned piglets.
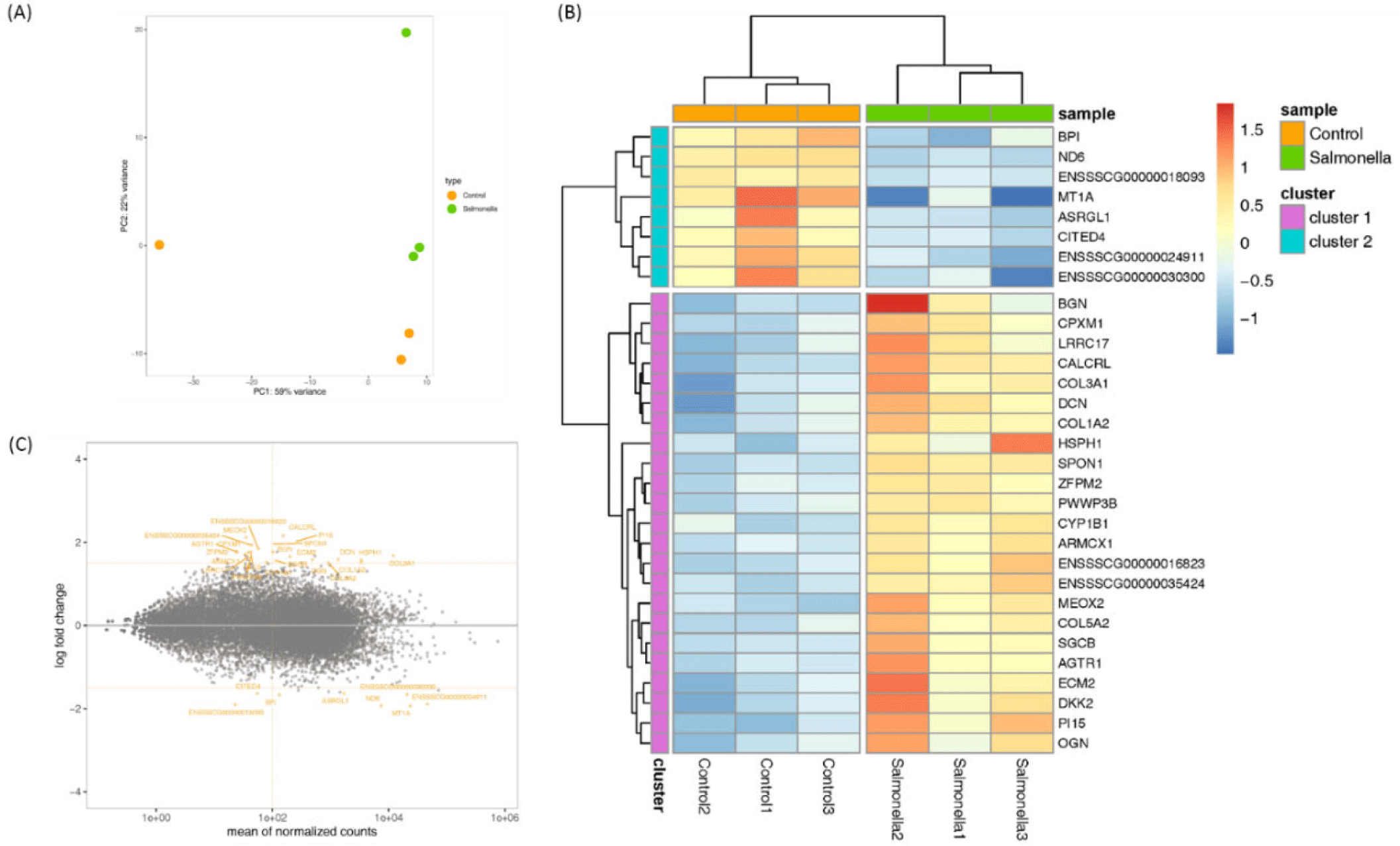
We used WebGestalt to perform DEG co-occurrence analysis in the small intestine of piglets by comparing control and salmonella-infected groups and identified 10 gene ontology across cellular component ranges (Table 8). This analysis found that DEG is primarily involved in the extracellular mechanisms involved in salmonella adhesion to host cells during infection. 5 gene ontologies were associated with curli fimbriae and biofilm that were related to adhesion of salmonella to host cells (extracellular region part [GO:0044421], extracellular region [GO:0005576], extracellular space [GO:0005615], extracellular matrix [GO:0031012] and extracellular matrix component [GO:0044420]). Decorin (DCN), peptidase inhibitor 15 (PI15), carboxypeptidase X, M14 Family Member 1 (CPXM1), biglycan (BGN), collagen type III alpha 1 chain (COL3A1), dickkopf WNT Signaling Pathway Inhibitor 2 (DKK2), bactericidal/permeability-increasing protein (BPI), and leucine rich repeat containing 17 (LRRC17) were relevant to the gene ontologies associated with extracellular mechanisms. We also found 3 gene ontologies associated with the extracellular matrix structural constituent (GO:0005201), extracellular matrix structural constituent conferring compression resistance (GO:0030021), and lipopolysaccharide (LPS) binding (GO:0001530) in the molecular function range. Like gene ontologies in the cellular component range, these gene ontologies were related to extracellular mechanisms. In the biological process range, we found that DEGs were mainly involved in the immune response and symptoms associated with salmonella infection. 3 gene ontologies were associated with decrease of muscle growth and development in piglets (muscle tissue morphogenesis, muscle organ morphogenesis, and muscle cell proliferation) and another 3 gene ontologies were associated with symptoms that occur when a salmonella infection enters the bloodstream of a piglet (heart development, blood vessel morphogenesis, and blood vessel development). 1 gene ontology was involved in the immune response (negative regulation of immune system process). Zinc finger protein, multitype 2 (ZFPM2) and COL3A1 were relevant to most ontologies, such as muscle tissue morphogenesis (GO:0060415), muscle organ morphogenesis (GO:0048644), heart development (GO:0007507), muscle cell proliferation (GO:0033002), blood vessel morphogenesis (GO:0048514), and blood vessel development (GO:0001568). Calcitonin receptor like receptor (CALCRL) was relevant to heart development (GO:0007507), muscle cell proliferation (GO:0033002), blood vessel morphogenesis (GO:0048514), and blood vessel development (GO:0001568). BPI and LRRC17 were relevant to the negative regulation of immune system processes (GO:0002683). We subsequently performed KEGG pathway analysis and identified viral myocarditis (ssc05416) out of 10 pathways. This pathway was related to heart development, blood vessel morphogenesis, and blood vessel development among gene ontologies in the biological process range. Sarcoglycan beta (SGCB) was relevant to viral myocarditis (Table 9).
Additionally, we identified that 4 genes (ZFPM2, BPI, DCN, and BGN) among 31 DEGs were related to mammalian immune systems based on the Innate Immune Database (IIDB). In the salmonella-infected group, serum IgG levels increased significantly compared to IgG levels in the control group (Fig. 13).
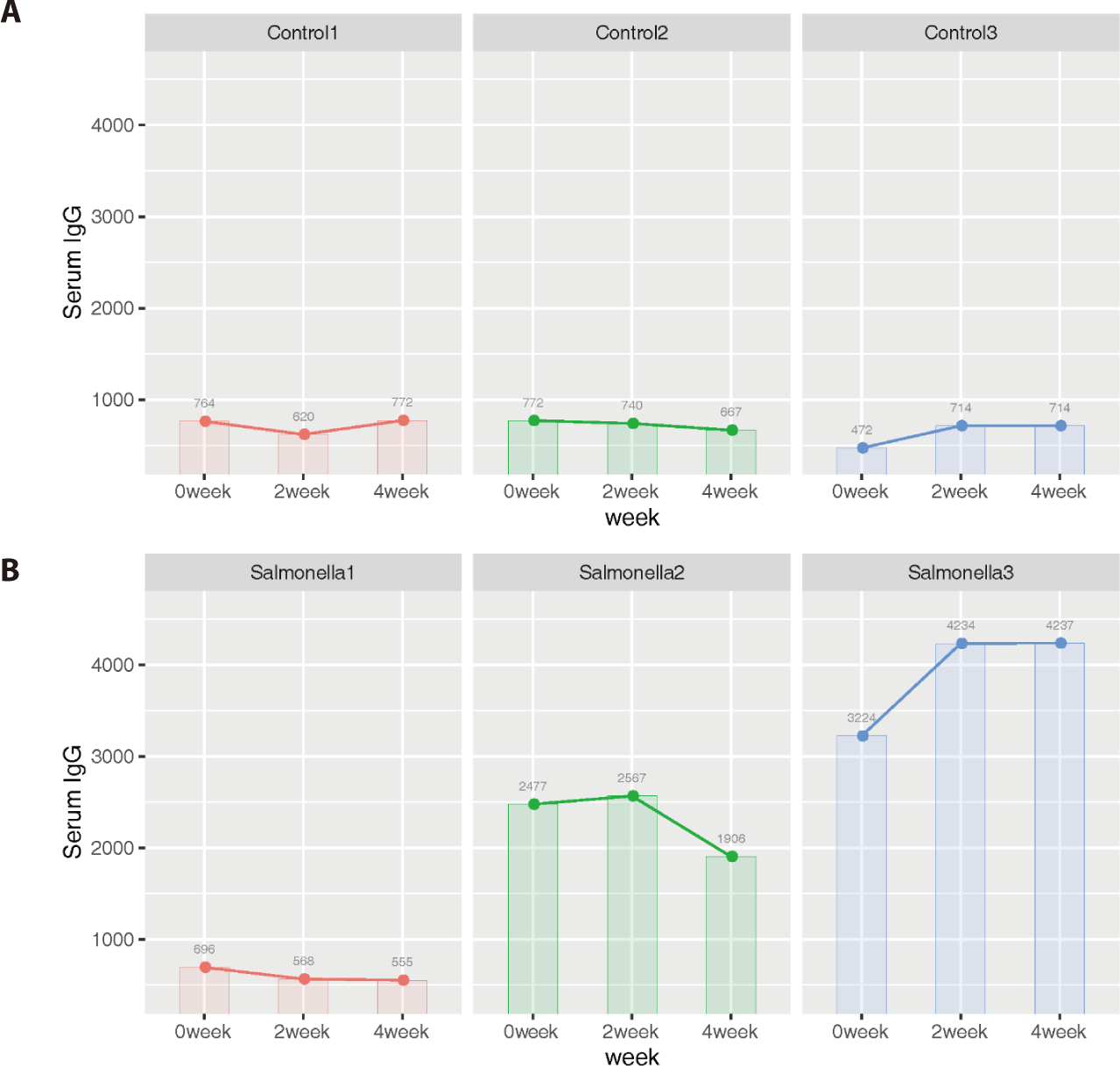
DISCUSSION
S. typhimurium is widely isolated serotype in pigs and S. typhimurium isolate HJL777 is a strongly virulent strain in mice. In pigs, the disease is susceptible to all ages, but it is most common in weaned pigs. The common clinical symptom in pigs with salmonella-infected is enterocolitis. S. typhimurium is highly contagious and can spread dramatically within pig populations once infected [4]. Piglets that recover from illness are often stunted and show slow growth. Our evaluation of clinical sign showed that all the piglets infected with salmonella had diarrhea. This indicates a change in intestinal condition caused by changes in the gut microbiota and gene expression in piglets infected with salmonella. Salmonella infections in piglets cause significant economic loss to pig farmers. Various methods to reduce or eliminate salmonella infection in young pigs have been tried, such as vaccination, competitive exclusion, feed and water treatments, antibiotic administration, disinfection of animals, and segregated weaning in clean accommodations [2]. However, when weaned pigs are infected with salmonella, little is known about the exact effects of salmonella on the intestinal microbiome, gene expression patterns, and biological functions based on genomics. To address this concern, it is desirable to eliminate the problem at the weaning step [2]. Thus, we sought to identify the change in the intestinal microbiota and biological function of piglets when infected with salmonella through the analysis of the fecal metagenome and intestinal transcriptome using 16S rRNA and RNA sequencing. Many studies have researched the metagenomics features of gut microbial using feces and the role of the small intestine, which is primarily involved in the immune system of the host. The presence of the intestinal microbiota positively contributes to the immune system of the host. For this reason, we performed analysis the changes of fecal metagenome and transcriptome in small intestine.
Photomicrographs of the epithelial layer of intestine were congruent with results from another studies [25]. In another study, in pigs 10 days after salmonella-infected, histologic analyses indicated that epithelial lesion, substantial leukocyte infiltration, mucosal hypermia, submucosal edema and lymphoid necrosis of polyphenylene sulfide (PPs) in the small intestine were caused. Histologic evaluation of the jejunum and ileum of salmonella-infected pigs showed severe inflammation compared to the control group. Similarly, another study showed that the intestinal mucosa in mouse infected with salmonella present intestinal erosion and sloughing of the surface epithelium at the tip of the villi through histological studies. Also, the villi core showed numerous inflammatory cells. Villi are important components of the gastrointestinal tract and play an important role in absorbing nutrients into the body across the small intestine [26,27]. And then, the density and size of villi are closely related to the intestinal absorption capacity. Changes in the gut microbiome ultimately alter the structure of the intestine. The structure of the mucous in the small intestine such as the size and shape of the villi was closely associated with the digestion and absorption capacity of the small intestine [28,29]. Therefore, we determined that changes in the intestinal microbiome of pigs caused by salmonella infection affected digestion and immunity by changing intestinal morphology such as the mucosal structure and villus size and shape.
By observing an increase in the diversity of gut microbiota in salmonella-infected pigs, we could predict that there was a decrease in the population of benefical microbes and an increase in harmful microbes in the infected pigs. Also, we could predict that the decrease in the number of viable cells in fecal samples of the salmonella-infected group causes a change in intestinal condition due to a decrease in beneficial microbes and an increase in harmful microbes. S. enterica serovar Typhimurium causes intestinal inflammation in pigs, disrupting microbial community composition [30,31]. For instance, in piglets infected with salmonella, Arguello et al. [32] identified that the number of beneficial bacteria, such as Bifidobacterium and Lactobacillus, decreased and the number of harmful bacteria, such as Citrobacter, and anaerobic bacteria, including Clostridium, Ruminococcus, or Diallister increased in the ileal mucosa. Therefore, intestinal inflammation related to weaning causes a disturbance of the intestinal microbiome, which promotes the growth of harmful intestinal bacteria [33].
When the fecal microbiome of the control and salmonella-infected groups in piglets was compared, we identified differences in abundance of OTUs of Firmicutes and Bacteroidetes at the phylum level. The population of Firmicutes and Proteobacteria increased and Bacteroidetes decreased in the salmonella-infected group. In a previous study, salmonella was difficult to grow in an environment with a high population of Bacteroidetes. Also, when a host was infected with salmonella, the number of Bacteroidetes decreases, making it difficult to protect the host from diseases [34]. Using inbred mouse strains with salmonella intestinal burdens, it was demonstrated that Bacteroidetes species limit salmonella infection in the gut. They found that Bacteroidetes production of the short-chain fatty acid (SCFA) metabolic byproduct propionate directly limits salmonella growth in vitro by inhibition intracellular pH homeostasis and limited intestinal expansion and fecal shedding in vivo. Therefore, this protected the mice from salmonella. The results of our study revealed that the number of Bacteroidetes decreases in the salmonella-infected group; these results were similar to the aforementioned study. In addition, beneficial microbes were similarly reduced at order and class level. Enteropathogenic salmonella cause inflammation and necrosis in the small and large intestines, which can cause diarrhea and sepsis. These disease is most commonly caused in weaned pigs [35]. Diarrheal illnesses may change the composition of microbial communities such as Firmicutes and Bacteroidetes in the intestine, causing mucosal damage. In inflammatory bowel disease (IBD), the phyla Bacteroidetes and Firmicutes shifted and Proteobacteria increased in the mucosa [36]. Increased numbers of Spirochaetes and Proteobacteria, which are well known as pathogenic microbes, were detected in the salmonella-infected group. Pathogenic Spirochaetes are the cause of important human and pig diseases such as leptospirosis and swine dysentery [37]. Spirochaetes bacteria were at the site of interaction of host tissue and the immune system [38]. Also, Spirochaetes had unique membrane structures that interacted with the immune system. Spirochaetes have LPS known as the pro-inflammatory component of gram-negative bacteria and glycolipids are associated with the inflammatory response during spirochetal infections. Proteobacteria are often the cause of intestinal diseases and constituted the most common phylum in the gut microbiome of diarrheic piglets. The phylum Proteobacteria contains many gram-negative pathogenic bacteria such as Salmonella, Helicobacter and Vibrio. And then, the increase of Proteobacteria can be seen as a potential indicator of unbalance in gut microbiome [39–41]. In studies of mice with innate immune system deficiencies, evidence was provided supporting a causative role of Proteobacteria in intestinal inflammation [42,43]. The results of our study revealed that the number of Spirochaetes and Proteobacteria increases at the phylum level in the salmonella-infected group; these results were similar to the aforementioned study. We predicted that reduction of Bacteroidetes and Firmicutes due to salmonella infection caused proliferation of salmonella in the small intestine and an increase in harmful bacteria such as Spirochaetes and Proteobacteria, which caused inflammatory reactions in the small intestine.
We confimed differences in abundance of OTUs of Prevotellaceae and Ruminococcaceae at family level. The population of Ruminococcaceae increased in the salmonella-infected group when compared to the control group. In previous study, the increase of Ruminococcaceae in weaned piglets showed a difference frome the levels during the nursing period [44]. Members of the family Ruminococcaceae have been associated with cellulolytic activity and production of SCFAs, which have many benefits in controling gut health, inhibition of salmonella growth, and having anti-inflammatory effects. Therefore, the number of Ruminococcaceae in the intestine increased in order to inhibit salmonella growth in salmonella-infected piglets.
We used PICRUSt analysis to predict functional profiling of microbial communities based on the 16S rRNA gene sequence. We analyzed functions at two levels (KO level 2 and KO level 3). Analyses of KO level 2 functions identified 11 differentially abundant KOs among control and salmonella-infected group. The pathways of biosynthesis of secondary metabolites and the immune system were identified to have a slightly higher abundance in the control group. In a previous study, microbes were shown to have the potential to biosynthesize many secondary metabolites that may mediate important host-microbe and microbe-microbe interactions [45]. Also, even if a host with normal intestinal microbiota is infected with harmful microbes, the infection does not take hold because it is affected by the immune response and metabolite production by intestinal microbes [46]. Therefore, we could predict that the salmonella-infected group had lower immune function and metabolite production capacity than control group with normal intestinal microbiota because the salmonella-infected group did not have normal intestinal microbiota due to salmonella infection. Weaned piglets have an immunity gap. This immunity gap occurs when the passive immunity from maternal immune factors provided through the sow’s colostrum has decreased and the active immunity from piglet itself has not increased quickly enough. During this time, piglets are very vulnerable to infections, which makes them susceptible to disease and increases mortality [47]. Our samples were collected 9 weeks after birth. Therefore, we could predict that when piglets susceptible to infection due to the immunity gap are infected with salmonella, the immune system will not work as well as the control piglets.
The pathway of lipid metabolism was identified as having a higher abundance in the salmonella-infected group. A previous study in chickens showed that salmonella infection could increase lipid synthesis and decrease lipid transportation in chicken hepatocytes. These changes can cause excessive lipid accumulation in the liver. Also, the necrosis of hepatocytes was observed in pig livers during salmonella infection. In mice infected-salmonella, the increased concentrations of free fatty acids (FFA) in plasma caused dyslipidemia [48]. In the current study, we could predict that intestinal microbes in piglets infected with salmonella were involved in increasing lipid metabolism and causing abnormalities. Furthermore, these microbes might be involved in causing necrosis of hepatocytes and liver disease.
We could observe functions such as butanoate metabolism, propanoate metabolism, and fatty acid metabolism in 29 differentially abundant KOs at level 3. These functions had a higher abundance in the salmonella-infected group than in the control group. Also, these functions were associated with SCFAs. SCFAs are produced by anaerobic bacteria such as Bacteroides in the intestine and include butanoate and propionate. SCFAs are components of phospholipids and glycolipids and are important materials for biofilms such as cell membranes and organelle membranes [49]. In rapidly proliferating cells, cells modulate the activation of lipid anabolic metabolism, cell membrane formation, energy storage and production of signaling molecules. Fatty acids produced by fatty acid synthases led to phospholipid biosynthesis and were used to synthesize cell membranes [50–52]. In our study, we could predict that when piglets were infected with salmonella, fatty acid biosynthesis increased in order to produce SCFAs to form the cell membranes of harmful bacteria, contributing to the proliferation of these harmful bacteria.
We performed gene ontology analysis (specifically analyzing the biological process, cellular component, and molecular function sub-ontologies) to gain a biological understanding of 31 DEGs in the control and salmonella-infected groups. In cellular component range, 5 gene ontologies were involved in extracellular mechanisms that are associated with salmonella adhesion to host cells during infection. Adhesion of salmonella to the intestinal epithelial surface is the first initiation of disease and is closely related to its colonization of the intestine. The extracellular matrix (ECM) can consist of a mixture of various polysaccharides, proteins and nucleic acids [53,54]. Salmonella forms biofilms on surfaces of host cells and produces an ECM having curli as the main protein component [55,56]. Curli are amyloid fibers that are related to adhesion to surfaces, cell aggregation, biofilm formation and environmental persistence, among other things [57]. Among the various fimbriae structures, curli fimbriae are encoded by the csg or agf operon and are known to play an important role in the adhesion of salmonella to surfaces in host cells by promoting biofilm formation upon contact with host cells [58,59]. In humans, Salmonella, which causes typhoid fever, forms a biofilm encapsulated by an ECM. The biofilm is a bacterial colony formed by various extracellular structures of bacteria and forms a network based on cellulose and LPS as well as curli fimbriae. It helps to adhere to the surface of bacteria and protects them from the external environment [60]. In addition to fimbriae, a variety of surface-adhesive proteins called adhesion are known to help in the attachment of salmonella by interacting with receptors on the host tissues or cell surfaces [61].
Derangement of the gut microbial community caused by salmonella infection could induce propagation of harmful microbes, thus causing and exacerbating intestinal inflammation [62]. We assumed that the increased intestinal microbial diversity in piglets infected with salmonella was due to increased numbers of harmful microbes in the small intestine, which would increase gut inflammation and the expression of immune-related genes to form an immune response. Therefore, we predicted the expression patterns of DEGs associated with our assumption. We performed gene ontology analysis (specifically the cellular component, molecular function, and biological process sub-ontologies) to help understand the biological functions of 31 DEGs between the control and salmonella-infected groups. We then identified 4 DEGs by gene ontology and the IIDB associated with extracellular mechanisms and inflammatory/immune responses.
Out of 4 DEGs, BGN, a key member of the small leucine-rich proteoglycan family, is an important component of the ECM with proinflammatory effects. BGN is typically expressed in the nerve, bone, cartilage, skin, and muscles, modulating the morphology, growth, adhesion, bone mineralization, inflammation, migration, and differentiation of epithelial cells [63]. BGN may regulate inflammation and innate immunity [64]. In recent studies, BGN activates inflammatory pathways by signaling through toll-like receptor (TLR) 2 and 4 in macrophages, resulting in secretion of tumor necrosis factor (TNF)α and mature interleukin (IL)-1β. In receptor knockout mice, BGN can activate the inflammatory pathway and trigger a downstream signaling pathway by directly binding to these receptors [65,66]. The upregulation of BGN has been reported in multiple types of solid cancer, including ovarian carcinoma, prostate cancer, pancreatic cancer, gastric cancer, and colon cancer. Overexpressed BGN has been reported to be associated with the aggressive growth and metastasis of tumors [67,68].
BGN expression in cancer cells was accompanied by an increased expression of the proteoglycan DCN. DCN, a member of the small leucine-rich proteoglycan gene family, is one of the important components of the ECM. DCN plays of role as a ligand of various cytokines and growth factors by directly or indirectly interacting with the signaling molecules related to cell growth, adhesion, metastasis, proliferation and differentiation [69]. DCN interacts with TLR2 and TRL4 in macrophages, at the same time causing transient activation of mitogen-activated protein kinase (MAPK) and nuclear factor κ-light-chain enhancer of activated B cells (NF-κB) signaling pathways. The result is improved release of inflammatory factors such as TNF-α, IL-12p70 and IL-10. In a mouse study, Zhao et al. the correlation between DCN expression and autophagy in the intestinal tissue of mice with IBD. The expression of DCN and the autophagy protein was increased in the intestinal tissues of the IBD mice [70]. Autophagy plays of role as an important cellular pathway for the maintenance of homeostasis. In addition, autophagy is closely associated with inflammation and is induced by a variety of inflammatory factors [71]. In a mouse study, Xiuli Bi X et al. [72] used a DCN-deficient (DCN-/-) mouse model to identify the importance of DCN in intestinal carcinogenesis. They found that targeted inactivation of DCN cause intestinal tumor formation [70].
ZFPM2 plays an important role in many cells with immune system development, differentiation, tumor formation and function. ZFPM2 is particularly associated with fine adjustment the TLR-triggered innate immune response. In mouse study, overexpression of ZFPM2 increased the production of proinflammatory cytokines such as TNF-α, IL-6 and IFN-β [73]. BPI (bactericidal permeability increasing protein) is antimicrobial protein in neutrophils. BPI specifically binds to the surface of gram-negative bacteria through the lipid A component of LPS and acts as a killer of gram-negative bacteria. It is part of the innate immune system [74]. In human studies, when salmonella is ingested by the host, salmonella may be exposed to various antimicrobial peptides and proteins such as BPI [74,75].
Four DEGs were identified by gene ontology and IIDB analysis and were found to be related to inflammatory and immune responses. Among these genes, three genes (BGN, DCN, and ZFPM2) were up-regulated and one gene (BPI) was down-regulated in piglets infected with salmonella. We predicted that in cases of up-regulated gene expression (BGN, DCN, and ZFPM2), an increase of harmful microbes in the small intestine due to salmonella infection leads to an increase in the inflammatory response as well as an increase in the expression of immune genes caused by deterioration of the intestinal condition. In the case of the down-regulation BPI, we hypothesized that an increase of harmful microbes in small intestine caused by salmonella infection would lead to a decrease of bactericidal permeability proteins and loss of the ability to kill the bacteria.
Like gene ontologies in the cellular component range, the gene ontologies in the molecular function range were related to extracellular mechanisms. LPS, the main component of the outer membrane of Gram-negative bacteria, is associated with adhesion or invasion of epithelial cells. The previous studies demonstrated that in the LPS-defective mutants of Salmonella, the colony of the mouse intestine is damaged and the mutants lacking the O antigen are attenuated in the mouse [76]. In our study, we predicted that when piglets are infected with salmonella, salmonella forms a variety of ECM such as curli fimbriae, cellulose, and LPS for attachment before invasion into tissues and cells, forming a colony called biofilm. In other words, we could predict that this extracellular mechanism would work in piglets infected with salmonella.
In the biological process range, 3 gene ontologies were associated with symptoms that occur when salmonella infection enters the bloodstream of piglet: heart development, blood vessel morphogenesis, and blood vessel development. In a previous study, it was determined that when salmonella infection enters bloodstream of the host (bacteremia), it can infect tissues throughout body of the host, including the lining of the heart or valves (endocarditis) and the lining of blood vessels [77]. Therefore, we predicted that salmonella infection in piglets could cause heart disease. Another 3 gene ontologies were associated with decrease of muscle growth and development in piglet: muscle tissue morphogenesis, muscle organ morphogenesis, and muscle cell proliferation. A previous study in chickens identified that when they were infected with salmonella, muscle growth was reduced. 1 gene ontology was involved in immune response: negative regulation of immune system process. Thus, we predicted that salmonella infection causes regulatory mechanism to suppress immunity in the host.
We subsequently performed KEGG pathway analysis and identified viral myocarditis (ssc05416) out of 10 pathways. This pathway is related to heart development, blood vessel morphogenesis, and blood vessel development among gene ontologies in the biological process range. In a previous study, when salmonella infection entered bloodstream (bacteremia) in host, it was shown that it could infect tissues throughout the body of the host, including the lining of heart or valves (endocarditis) and the lining of blood vessels in the host. Another study reported that a human male developed acute myocarditis following salmonella infection [78,79]. Therefore, we predicted that when piglets are infected with salmonella, it can cause heart diseases such as viral myocarditis.
Immunoglobulin G (IgG), an antibody present in blood and extracellular fluid, plays of role as protection the body from infection by binding pathogens such as viruses, bacteria and fungi. We expected that serum IgG would be a more direct indicator of piglet health than other indicators such as average daily weight gain and feed intake. Therefore, we measured its concentrations at 2-week intervals in the control and salmonella-infected groups. The data from serum IgG analysis implied that salmonella-infected piglets have a stronger immune response than non-salmonella-infected (control) piglets in order to resist salmonella infection in the small intestine.
CONCLUSION
In conclusion, we predicted that salmonella infection in weaned piglets causes increases of harmful bacteria in the small intestine and increases in lipid metabolism related to proliferation of harmful bacteria and the intestinal inflammatory response. Through gene ontology analysis, we identified DEGs that were involved in extracellular mechanisms associated with the adhesion of salmonella to the intestinal epithelial surface, a key first stage of pathogenesis that is central to colonization of intestinal epithelial cells. Furthermore, we confirmed that these genes were related to inflammatory and immune responses that occur during salmonella infection. Our study confirmed the change in the intestinal microbiome its function as well as the further biological function of the host based on genomic analysis from samples from salmonella-infected piglets. Our data suggests the effects of salmonella on changes in the intestinal microbiome, gene expression patterns, and biological function.